AI-Based Demand Forecasting: Improving Prediction Accuracy and Efficiency
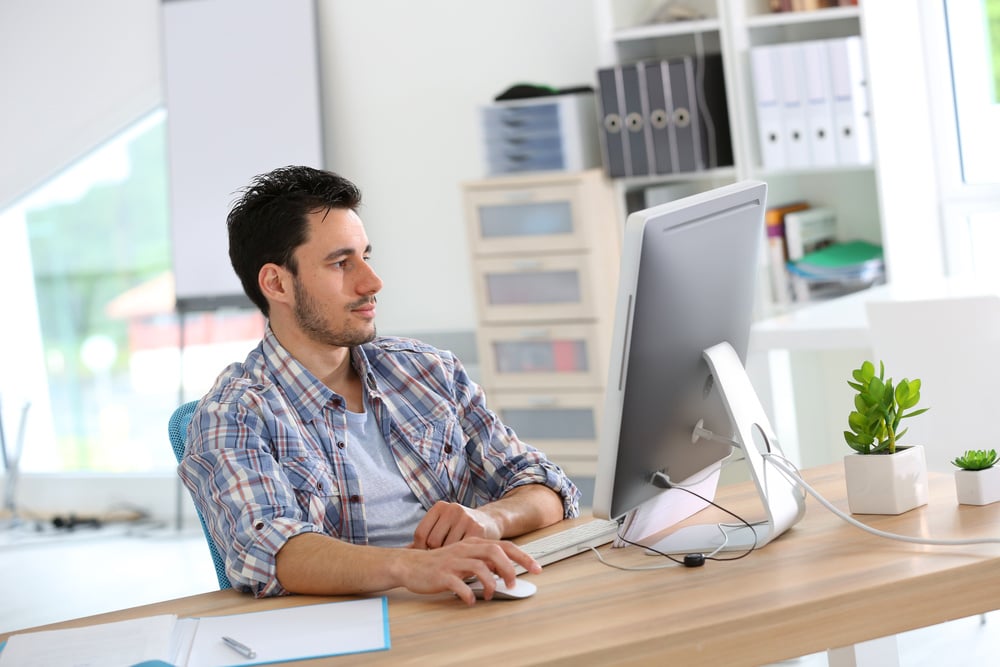
Advanced machine learning algorithms can sift through data, identifying seasonal trends and adjusting forecasts in real time. This capability allows businesses to respond more swiftly to market changes and external influences that traditional methods could not quickly adapt to. As a result, AI-based demand forecasting is setting new standards for precision in predicting future demand.
The efficiency brought about by AI in forecasting is multi-dimensional. It reduces the time and resources spent on manual data analysis, enables more accurate inventory planning, and minimizes the risks of stockouts or overstock situations. By leveraging AI, businesses are not only improving their bottom lines but also offering better service to their customers through more reliable product availability.
The Evolution of Forecasting: From Traditional Methods to AI Integration
Historically, demand forecasting relied on traditional methods that utilized past sales data to project future demand. While effective to a degree, these methods often struggled with large volumes of data and complex variables, leading to less reliable predictions. The integration of AI has transformed this landscape, employing advanced machine learning algorithms to analyze data more comprehensively. This evolution marks a transition from a reliance on simple historical trends to a more dynamic analysis that incorporates seasonal trends, external influences, and real-time data, vastly improving forecasting accuracy.
Traditional vs AI-Based Forecasting: A Comparative Analysis
Comparing traditional forecasting methods to AI-based approaches reveals stark differences in their capabilities. Traditional methods, while foundational, lack the agility to account for sudden market shifts or external influences effectively. They operate within a set framework that struggles to handle the complexity and volume of data characteristic of today's markets. In contrast, AI-based forecasting thrives on this complexity, leveraging it to provide more accurate and nuanced predictions that traditional methods cannot achieve.
The Driving Forces Behind the Shift to AI in Demand Forecasting
The shift towards AI in demand forecasting is driven by the need for greater accuracy, efficiency, and adaptability in predicting market demands. As businesses operate in increasingly volatile markets, the limitations of traditional forecasting methods become more apparent. AI's ability to process and analyze vast datasets rapidly, consider a multitude of variables, and learn from outcomes propels its adoption. This shift is not merely a trend but a response to the pressing need for more sophisticated forecasting tools in the digital age.
Understanding AI-Based Demand Forecasting
AI-based demand forecasting represents a leap forward in how businesses predict future demand. By harnessing forecasting accuracy and making precise predictions, companies can optimize their supply chain operations more effectively than ever. This approach leverages the power of AI to analyze patterns and predict future needs, ensuring businesses are better equipped to meet market demands efficiently.
Fundamentals of AI in Demand Forecasting
At the core of AI-based forecasting lies the strategic application of machine learning and data analytics to predict future demand trends. This process involves collecting historical and real-time data, analyzing it to identify patterns, and applying these insights to make informed predictions. Through AI, businesses can achieve a level of precision and efficiency previously unattainable with traditional forecasting methods.
Data Collection and Preparation
The initial step in AI-based demand forecasting involves collecting data from various sources. This stage is crucial, as the quality and breadth of collected data directly influence the accuracy of the predictions. Businesses must meticulously collect data, ensuring it is clean, relevant, and comprehensive to form a solid foundation for the forecasting models. This preparation paves the way for more sophisticated analyses and predictions.
Selecting Appropriate AI Algorithms
Choosing the right AI algorithms is vital in demand forecasting. Neural networks and linear regression are among the most commonly used algorithms due to their ability to handle large datasets and identify complex patterns within the data. The selection process involves evaluating the specific needs of the business and the nature of the data to determine the most effective algorithm for accurate demand forecasting.
The Mechanics of AI for Enhanced Demand Forecasting
The integration of AI into demand forecasting significantly improves both accuracy and efficiency. AI algorithms analyze past and present data to predict future demand with a higher degree of precision. This capability enables businesses to make informed decisions quickly, adapting to market changes with agility.
Predictive Analytics and Machine Learning
Predictive analytics and machine learning are at the forefront of AI-based demand forecasting. These technologies allow for the analysis of historical data patterns to forecast future demand, including seasonal variations. Machine learning models continually learn and improve from new data, enabling them to adapt predictions to changing market conditions dynamically.
Integration of NLP and Image Recognition
The integration of natural language processing (NLP) and image recognition into demand forecasting represents a significant advancement. NLP allows for the analysis of unstructured data, such as customer reviews or social media posts, to gauge consumer sentiment and predict demand trends. Image recognition can analyze consumer behavior and preferences, offering additional insights that enhance the accuracy of demand forecasts.
The Multifaceted Benefits of AI-Based Demand Forecasting
AI-based demand forecasting offers a range of benefits, from increased accuracy to enhanced operational efficiency. By leveraging the predictive power of AI, businesses can anticipate market fluctuations more effectively, ensuring they are well-positioned to meet demand and capitalize on market opportunities.
Surge in Forecast Accuracy and Operational Efficiency
The incorporation of internal and external data into AI-based forecasting models leads to a significant improvement in forecast accuracy and operational efficiency. By analyzing a broader range of data points, AI algorithms can provide more accurate predictions, enabling businesses to optimize inventory levels, reduce waste, and improve overall operational performance.
Strategic Decision-Making and Increased Profitability
Enhanced forecasting accuracy directly contributes to more strategic decision-making and increased profitability. With more reliable demand predictions, businesses can allocate resources more efficiently, streamline their supply chains, and minimize costs associated with overproduction or stockouts. This strategic advantage not only boosts profitability but also strengthens competitive positioning in the market.
Tailored Demand Predictions and Real-Time Insights
AI-based demand forecasting enables businesses to predict demand with a high degree of precision, leveraging both natural language processing and analysis of internal and external data. This tailored approach provides real-time insights into consumer behavior and market trends, allowing companies to adjust their strategies proactively and maintain a competitive edge in fast-moving markets.
Strengthening Supply Chain Relationships Through Data Democratization
Data democratization within the realm of AI-based demand forecasting marks a pivotal shift from manual methods to more collaborative and transparent supply chain planning. This transition not only enhances customer service but also fosters a more resilient supply chain ecosystem. By providing all stakeholders with access to crucial forecasting insights, businesses can align their strategies more effectively, ensuring that supply meets demand in the most efficient manner possible. This approach minimizes silos, promotes openness, and encourages shared responsibility for meeting consumer needs.
Practical Applications: AI Demand Forecasting Across Industries
The practical applications of AI-based forecasting are vast, spanning multiple industries where the need to streamline processes is paramount. By harnessing the power of AI, businesses can predict market demands with unprecedented accuracy, ensuring they remain competitive and responsive to changes in consumer behavior. This technological advancement is not just about automating tasks but about enabling smarter, data-driven decisions that can significantly impact the bottom line.
Retail and E-Commerce: Dynamic Pricing and Inventory Management
In the retail and e-commerce sectors, generative AI has revolutionized dynamic pricing and inventory management, thereby maximizing revenue. By analyzing vast datasets, AI can predict consumer buying patterns, adjust prices in real-time, and ensure inventory levels meet demand without overstocking. This not only improves profitability but also enhances the customer experience by ensuring product availability and competitive pricing.
Manufacturing: AI-Driven Production Optimization
Manufacturing has seen a significant transformation with AI-driven production optimization. By analyzing data from various sources, AI algorithms can predict production needs, optimize resource allocation, and reduce waste. This ensures that manufacturing processes are lean, efficient, and aligned with market demand, thereby reducing costs and improving product availability.
Healthcare: Patient Flow and Resource Allocation Forecasting
The healthcare sector benefits from generative AI for accurately forecasting patient flow and resource allocation, including staffing levels. By analyzing usage patterns, AI can predict peaks in patient admissions, ensuring that resources are allocated efficiently. This not only improves patient care but also optimizes the use of facilities and personnel, leading to a more responsive and cost-effective healthcare system.
Energy Sector: Real-Time Energy Demand Prediction
AI plays a critical role in the energy sector by enabling real-time energy demand prediction. This capability allows for more efficient energy distribution, reducing waste and ensuring that energy supplies are optimized to meet consumer and industrial needs. By predicting fluctuations in energy demand, providers can adjust their production strategies in real time, contributing to a more sustainable and efficient energy landscape.
Automotive: Streamlining Production Planning
In the automotive industry, an AI-based demand forecasting system significantly enhances resource management and production planning. By accurately forecasting demand for various models, manufacturers can adjust production schedules, manage supply chains more effectively, and reduce inventory costs. This not only streamlines operations but also ensures that consumer demand is met efficiently, maintaining competitive advantage.
Implementing AI in Demand Forecasting
Implementing AI in demand forecasting involves a comprehensive approach, incorporating neural networks, natural language processing, linear regression, and other AI technologies. Data scientists play a crucial role in developing models that can sift through vast datasets, identify patterns, and predict future demand with high accuracy. This process not only enhances forecasting accuracy but also provides businesses with actionable insights to inform strategic decisions.
Identifying Objectives and Outlining Scope
At the outset, identifying objectives and outlining the scope for AI-powered demand forecasting tools is crucial for optimizing inventory management. This step ensures that the deployment of AI technologies is aligned with business goals, addressing specific challenges such as reducing stockouts or improving product availability. Clear objectives guide the selection of data sources, AI models, and analytics strategies, laying a solid foundation for successful implementation.
Navigating Data Collection and AI Model Development Hurdles
Navigating the complexities of data collection and AI model development presents significant challenges. Ensuring data quality, integrating diverse data sources, and selecting the most effective AI algorithms require meticulous planning and expertise. Overcoming these hurdles is essential for developing robust AI models that can accurately predict demand and drive strategic business decisions.
Ethical Considerations and Regulatory Compliance
Ethical considerations and regulatory compliance are paramount in the development and implementation of AI-based demand forecasting. It is imperative to address privacy concerns, ensure data security, and comply with relevant regulations. Transparent AI practices and adherence to ethical guidelines not only build trust with stakeholders but also safeguard businesses against legal and reputational risks.
Ongoing AI Model Management and Evolution
Effective AI model management and evolution involve continuous monitoring, updating, and refinement of algorithms to adapt to changing market conditions. This iterative process ensures that AI-based forecasting remains accurate and relevant, providing businesses with the insights needed to make informed decisions. Regular evaluation and adjustment of AI models are crucial for maintaining their effectiveness over time.
Overcoming Challenges in AI-Based Demand Forecasting
Overcoming challenges in AI-based forecasting requires a strategic approach to ensure forecasting accuracy and precise predictions. Key issues such as data quality, integration, and the complexity of supply chain operations necessitate innovative solutions. AI streamlines the forecasting process, but businesses must remain vigilant in addressing these challenges to fully leverage AI's potential for enhanced decision-making.
Addressing Data Quality and Integration Issues
Addressing data quality and integration issues is crucial for the success of AI-based forecasting. AI streamlines the analysis of complex datasets, but the accuracy of its predictions depends on the quality of the data fed into it. Ensuring data is clean, comprehensive, and well-integrated across systems is fundamental to achieving reliable forecasting outcomes.
Simplifying Complexity and Enhancing Model Transparency
Simplifying the complexity of AI models and enhancing their transparency are essential steps toward building trust and understanding among stakeholders. By making AI algorithms more interpretable, businesses can facilitate better decision-making and foster greater confidence in AI-driven forecasts. This not only demystifies AI but also encourages broader adoption across industries.
Adapting to Global Uncertainties and Continuous Maintenance
In the dynamic realm of AI-based demand forecasting, the capacity to adapt to global uncertainties and ensure continuous maintenance of AI models stands paramount. These models, by design, must evolve with changing market conditions, geopolitical events, and unexpected global disruptions to maintain their predictive accuracy. The agility of AI systems in assimilating real-time data and learning from new patterns enables businesses to swiftly adjust their strategies in response to unforeseen challenges. Furthermore, continuous maintenance, involving regular updates and training of AI models with fresh data, ensures that the forecasting remains relevant and accurate, thereby safeguarding businesses against the volatilities of global markets.
Breakthroughs and Innovations in AI for Demand Forecasting
AI technology has ushered in a new era of breakthroughs and innovations in demand forecasting, transforming how businesses predict future demand and make strategic decisions. Advances in machine learning algorithms, data processing capabilities, and real-time analytics have significantly enhanced the accuracy and efficiency of demand forecasts. These innovations not only enable a deeper understanding of consumer behavior and market trends but also empower businesses to optimize their operational processes, reduce waste, and increase profitability. As AI continues to evolve, its role in demand forecasting is set to become even more critical, driving operational excellence and competitive advantage.
Generative AI and Its Influence on Forecasting Accuracy
Generative AI has emerged as a game-changer in the field of demand forecasting, significantly influencing forecasting accuracy. By leveraging the power of generative models, businesses can simulate and analyze countless scenarios, enabling the generation of highly accurate demand predictions. This capability not only enhances decision-making processes but also allows companies to anticipate market changes more effectively. The predictive prowess of generative AI, rooted in its ability to understand and recreate complex patterns, marks a substantial leap forward in forecasting, offering businesses the tools to navigate market dynamics with unprecedented precision.
AI Breakthroughs: Neural Networks and Beyond
The advent of neural networks and their application in AI-driven forecasting has marked a significant milestone in the realm of strategic planning. These AI technologies excel at analyzing vast amounts of data, including weather patterns and market shifts, far beyond the capacity of human analysts. This process ensures that predictive analytics can be utilized to forecast demand with a high degree of accuracy, thereby optimizing storage costs and adjusting to emerging market trends. The ability of AI to process and analyze data at this scale enables businesses to make informed decisions rapidly, ensuring they remain agile in a constantly evolving market landscape.
Case Studies: AI Transforming Business Strategies Across Sectors
Across various industries, AI-based forecasting has revolutionized how businesses streamline processes and make strategic decisions. For instance, in the retail sector, AI-powered tools analyze consumer behavior and market trends to predict future demand, thereby optimizing inventory management and reducing waste. In manufacturing, AI-driven forecasts inform production planning, ensuring that supply meets anticipated demand efficiently. These case studies underscore the transformative impact of AI on business strategies, demonstrating its ability to drive operational efficiency, reduce costs, and enhance customer satisfaction.
Partnering for Success: Choosing the Right AI Development Service
Selecting the right AI development service is crucial for businesses aiming to leverage AI for demand forecasting. The ideal partner should offer a blend of advanced AI tools and strategic insights, enabling companies to anticipate demand, optimize inventory levels, and enhance supply chain efficiency. Critical factors include expertise in predictive analytics, generative AI capabilities, and a proven track record in integrating AI systems into existing business operations. Such a partnership empowers businesses to harness the full potential of AI in forecasting, driving strategic decision-making, and achieving a competitive edge in their respective markets.
Criteria for Selecting an AI Development Partner
Choosing an AI development partner necessitates a careful evaluation of several critical criteria. Foremost among these is the partner's proficiency in predictive analytics and generative AI, which are pivotal in generating accurate and advanced demand forecasts. Equally important is their experience in customizing AI systems to align with specific business operations and objectives. The ability to seamlessly integrate these systems into existing workflows, coupled with a deep understanding of production planning and demand trends, ensures that the chosen partner can effectively address potential risks while maximizing supply chain efficiency and strategic decision-making capabilities.
Spotlight on Leading AI Solutions Providers
The landscape of AI solutions for demand forecasting features several standout providers, each bringing unique strengths to the table. These leaders excel in deploying cutting-edge AI technologies, including predictive analytics and machine learning, to offer comprehensive forecasting solutions. Their expertise encompasses a broad spectrum of industries, enabling tailored approaches that meet specific business needs. By prioritizing innovation and customer success, these providers play a pivotal role in helping businesses navigate the complexities of demand forecasting, ensuring that they remain agile and competitive in a rapidly evolving market.
Navigating the Future of AI in Demand Planning
The future of AI in demand planning promises a landscape of continuous improvement and refinement. As AI technologies advance, businesses can expect even more accurate insights and predictive modeling capabilities. This evolution will enable companies to adjust production more effectively, optimize service levels, and respond proactively to market changes. The ongoing development of AI tools and algorithms will further empower businesses to harness the full potential of data analytics, ensuring that they can navigate the complexities of demand planning with confidence and strategic agility.
The Ongoing Evolution of AI Technologies and Their Impact
The ongoing evolution of AI technologies continues to reshape the landscape of demand forecasting. With each advancement, AI becomes more adept at providing businesses with the tools needed for making data-driven decisions. Enhanced computational power and sophisticated algorithms allow for deeper analysis of market trends and consumer behavior, leading to more precise predictions and strategic planning opportunities. As AI technologies grow more integrated into demand forecasting processes, their impact on operational efficiency, market responsiveness, and competitive advantage becomes increasingly profound, marking a new era in strategic business planning.
Preparing for Future Trends and Innovations in AI Demand Forecasting
As AI technologies advance, preparing for future trends and innovations in demand forecasting becomes essential for businesses aiming to maintain a competitive edge. This preparation involves staying abreast of developments in AI and machine learning, understanding the potential impact of these technologies on demand planning, and being ready to adapt business models accordingly. By embracing a forward-thinking approach, companies can leverage AI to not only improve forecasting accuracy but also to innovate and redefine their strategies to meet the demands of a rapidly changing market.
FAQs on AI-Based Demand Forecasting
AI-based forecasting represents a significant advancement in how businesses predict and respond to market demands. By leveraging AI-driven forecasting, companies can analyze vast amounts of data to make precise predictions, enhancing customer satisfaction through improved product availability and service delivery. This approach enables businesses to adapt more effectively to weather patterns, market shifts, and emerging market trends, ensuring they remain competitive in a dynamically changing environment.
How Does AI Enhance the Accuracy of Demand Forecasts?
AI-driven forecasting enhances the accuracy of demand forecasts by leveraging the ability to analyze vast amounts of data, including historical sales data, market trends, and even weather patterns. This comprehensive analysis, far beyond the scope of human analysts, allows AI technologies to identify patterns and predict future demand with a high degree of precision. The process ensures that businesses can make informed strategic planning decisions, optimize inventory levels, and reduce waste, thereby significantly improving operational efficiency and profitability.
Can AI Demand Forecasting Models Adapt to Seasonal Variations?
Yes, AI demand forecasting models are specifically designed to adapt to seasonal variations, making them highly effective for businesses that experience fluctuating demand throughout the year. These models analyze historical sales data and identify patterns related to seasonal changes, enabling them to anticipate shifts in demand. This capability allows businesses to adjust their inventory and production planning accordingly, ensuring they can meet customer demand efficiently while minimizing the risk of overstocking or stockouts.
Integrating AI Demand Forecasting into Existing Systems: What’s Involved?
Integrating AI demand forecasting into existing systems involves several critical steps to ensure seamless operation and maximum benefit. Initially, it requires a thorough assessment of current business processes and IT infrastructure to identify the most effective integration points. Following this, data must be prepared and cleaned to feed into the AI models accurately. The integration process also involves configuring the AI tools to align with specific business objectives and training staff to interpret and act on the insights generated. With careful planning and execution, businesses can enhance their forecasting capabilities, resulting in improved operational efficiency and decision-making.
The Role of Continuous AI Model Training and Validation
Ensuring the efficacy of AI-based forecasting hinges on continuous AI model training and validation. This process is paramount, as it allows the models to adapt to new data, emerging trends, and shifts in consumer demand. Continuous training refines the model's ability to make highly accurate predictions, enhancing its forecasting accuracy over time. Meanwhile, rigorous validation processes assess the model's performance against real-world outcomes, ensuring that predictions remain reliable. This cycle of training and validation is vital for maintaining the relevance and accuracy of AI in demand forecasting, enabling businesses to anticipate market shifts with greater confidence.
Capturing the Horizon: The Next Steps in AI-Based Demand Forecasting
The trajectory of AI-based demand forecasting is poised towards more nuanced and granular predictions. The next steps involve leveraging advanced AI algorithms to analyze deeper layers of data, uncovering intricate patterns that traditional models may overlook. This will likely include the integration of real-time data streams, enhancing the model's responsiveness to immediate market changes. Furthermore, the development of more sophisticated forecasting techniques that can dynamically adapt to various industry-specific challenges will be crucial. These advancements promise to unlock unprecedented levels of accuracy and relevance in demand forecasting, offering businesses a sharper competitive edge.
Towards a Smarter, More Predictive Future: The Road Ahead
The road ahead for AI-based demand forecasting is paved with the potential for transformative impacts on supply chains. Enhanced forecasting accuracy will empower businesses to optimize inventory planning, reduce waste, and improve resource utilization. The ability to anticipate demand shifts with greater precision will also enable supply chains to become more agile and resilient to market volatilities. As AI technologies continue to evolve, they will play a crucial role in creating supply chains that are not only efficient but also more sustainable and responsive to the changing needs of the global market.
Leveraging AI for Competitive Advantage: Strategic Insights for Businesses
Businesses can gain a substantial competitive advantage by harnessing AI-based forecasting to inform strategic decisions. By leveraging predictions based on comprehensive data analysis, companies can identify lucrative market opportunities and mitigate potential risks more effectively. This insight enables the formulation of strategic decisions that are both forward-looking and grounded in data. Moreover, the ability to anticipate market trends and align business strategies accordingly will be a key differentiator in increasingly competitive markets. AI's role in facilitating these strategic insights will become an indispensable tool for businesses aiming to lead in their respective sectors.
In Conclusion: The Transformative Power of AI in Demand Forecasting
The journey from traditional forecasting methods to AI-driven solutions marks a significant leap in how businesses anticipate and meet consumer demand. Artificial intelligence in demand forecasting has not only increased the accuracy of predictions but has also revolutionized inventory planning and strategic decision-making processes. By enabling efficient analysis of trends and patterns, and offering highly accurate demand predictions, AI empowers businesses to optimize their operations and resource utilization. As AI continues to evolve, its impact on demand forecasting promises to usher in an era of unparalleled precision and strategic agility, cementing its role as a cornerstone of modern business intelligence.
Reflecting on the Journey from Traditional Methods to AI-Driven Solutions
The transition from traditional forecasting models to AI-driven solutions represents a paradigm shift in how businesses approach demand planning. Traditional models, often constrained by the limitations of statistical models and historical data analysis, struggled to adapt to rapid market changes or capture emerging trends. In contrast, AI algorithms analyze vast datasets, identifying patterns that elude conventional methods. This shift has not only improved forecasting accuracy but also transformed strategic planning, enabling businesses to anticipate market shifts more effectively.
Embracing the Future: The Enduring Impact of AI on Demand Forecasting
The enduring impact of AI on demand forecasting is evident in its capacity to continuously learn from new data, refining its predictions to reflect current market realities. This adaptability is crucial for staying ahead in dynamic markets, where consumer demand can shift unexpectedly. AI's role in forecasting demand extends beyond mere prediction; it influences marketing campaigns, inventory planning, and overall strategic planning. As businesses embrace AI, they unlock new levels of operational efficiency and market responsiveness. The future of demand forecasting, powered by AI, promises not only more accurate predictions but also a deeper understanding of market dynamics, enabling businesses to navigate the complexities of the modern marketplace with confidence.