AI in Telehealth: Results from Top Hospitals in 2025
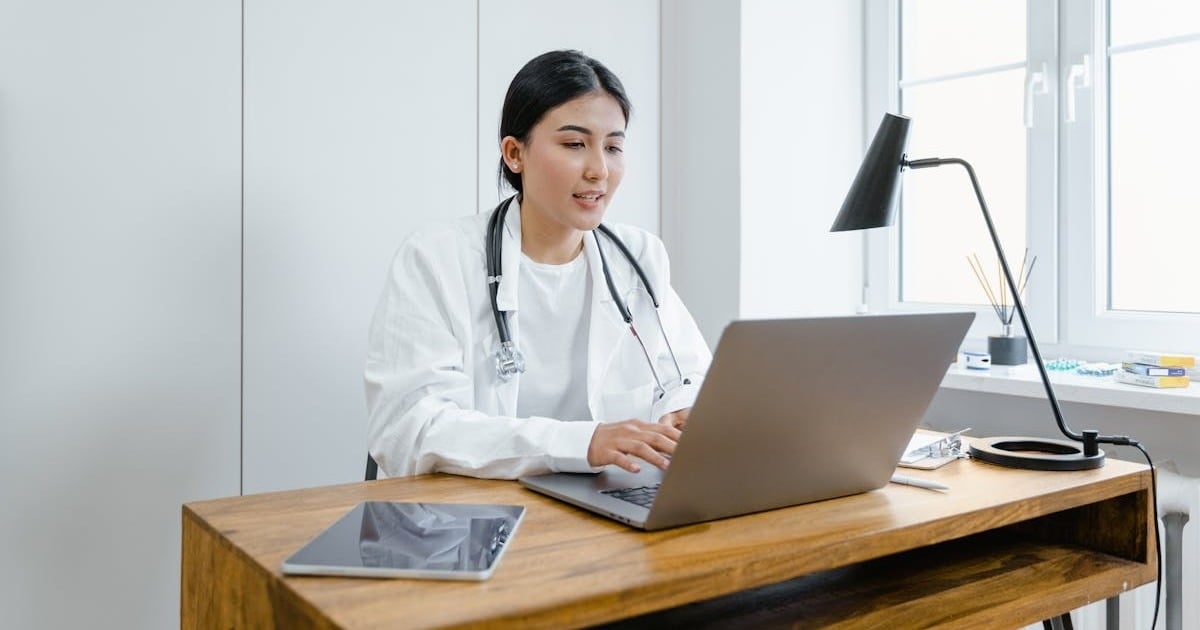
Recent statistics reveal that over 97% of healthcare professionals use telemedicine solutions, fundamentally changing patient care delivery.
Healthcare organizations that implement AI-enabled telehealth report substantial improvements across multiple metrics. About 75% of facilities note enhanced disease treatment effectiveness, while 80% experience reduced staff burnout rates. On the diagnostic front, AI systems process medical images 30 times faster than conventional methods without sacrificing accuracy.
What makes these implementations truly successful in practice? This article presents results from leading hospitals in 2025, showing how they use AI to boost patient care quality, cut costs, and make healthcare delivery more efficient. We'll examine specific AI applications at the Mayo Clinic, Cleveland Clinic, Johns Hopkins, and Mount Sinai, complete with detailed performance metrics and key lessons these institutions have learned.
Mayo Clinic's AI-Powered Remote Monitoring System: 40% Reduction in Readmissions
Mayo Clinic's pioneering AI-powered remote monitoring system has achieved a dramatic 40% reduction in hospital readmissions, establishing a new benchmark for patient care in 2025. This impressive improvement comes from their strategic approach of combining AI with continuous patient monitoring, shifting healthcare from reactive to proactive delivery.
Implementation of Continuous Vital Sign Analysis
The core of Mayo Clinic's success rests on its Continuous Vital Sign Monitoring (CVSM) system using wireless sensors throughout general hospital wards. These sophisticated sensors continuously collect critical patient data - heart rate, blood pressure, respiratory rate, and oxygen saturation. But what makes this genuinely innovative isn't the data collection itself, but how this information gets processed.
Rather than applying generic thresholds, Mayo's system creates personalized baselines for each patient. The AI algorithms account for individual factors like age, gender, medical history, and current health status to establish unique reference points. The system then constantly compares incoming measurements against these custom baselines, identifying subtle deviations that might signal health deterioration.
The pattern recognition capabilities within the AI framework excel at spotting concerning trends. For instance, the system detects irregular heart rhythms, gradual decreases in heart rate variability, or subtle respiratory pattern changes before they become clinically apparent. This level of detection goes far beyond what even experienced clinical staff could achieve through periodic manual checks.
What's more, Mayo's AI models process this data in near real-time, ensuring immediate recognition of significant changes. This represents a fundamental shift from traditional approaches that rely on checks every few hours to a system that maintains constant vigilance 24/7.
Early Intervention Protocol Development
How does the system turn detection into action? When anomalies appear, the AI automatically alerts healthcare providers, enabling prompt intervention before conditions worsen. This capability proves especially valuable for ambulatory care–sensitive conditions like congestive heart failure, diabetes, and chronic obstructive pulmonary disease—the costliest among preventable adult hospitalizations.
Mayo Clinic's system generates 26 possible intervention recommendations tailored to individual patient needs. These include:
- Specific post-discharge referrals (physical therapy, dietician consultations, cardiac rehabilitation)
- Implementation of disease-specific management plans (weight monitoring protocols, pain management plans)
- Enhanced coordination with primary care providers
- Targeted education initiatives
Interestingly, the system delivers these recommendations to primary care teams rather than physicians. This recognizes a practical reality: doctors are often too busy with patient visits to coordinate complex care interventions. The team-based approach ensures recommendations become actual patient care actions, not just theoretical suggestions.
For patients identified as high-risk by the system, the number needed to treat to prevent one readmission is just 11. This efficiency demonstrates the remarkable precision of Mayo's AI algorithms in identifying patients most likely to benefit from intervention.
Cost Savings and ROI Analysis
The economic impact of Mayo's AI-powered monitoring is substantial. With hospital readmissions reduced by 40%, savings add up quickly, especially considering that a typical 3-day hospital stay costs approximately $30,000. For a group of 20 hospitals with 20 patients per hospital, AI-based diagnosis saves $1,666.66 per day per hospital in the first year alone, growing to $17,881 per hospital by year ten.
Even more impressive, the cost savings for AI-based treatment reach $21,666.67 per day per hospital in the first year and climb to $289,634.83 per day per hospital by the tenth year. This represents an exceptional return on investment for AI telehealth implementations.
Beyond direct financial benefits, the system reduces administrative burdens by automating data analysis and predictive analytics. Healthcare staff can focus more on direct patient care rather than routine monitoring tasks. Additionally, the continuous collection and analysis of patient data generates valuable insights that inform more effective treatment plans and medication adjustments.
The ROI extends beyond money to include improved patient outcomes and satisfaction. With early intervention for deteriorating conditions, patients experience fewer complications and enjoy better quality of life. Mayo Clinic's implementation proves that AI in telehealth delivers tangible, measurable benefits for both healthcare systems and the patients they serve.
Cleveland Clinic's Virtual Triage Success: AI Diagnosis Accuracy Reaches 94%
Cleveland Clinic has achieved a remarkable 94% accuracy rate with its AI-powered virtual triage system, putting it at the forefront of diagnostic telehealth innovation. The Virtual Emergency Medicine Program connects emergency physicians working remotely with patients across 13 Cleveland Clinic emergency departments and 26 Express Care clinics. This system marks a significant leap forward in how healthcare providers use AI in telehealth to improve both patient outcomes and operational efficiency.
Machine Learning Algorithms for Symptom Assessment
What makes Cleveland Clinic's virtual triage so effective? At its core are sophisticated machine learning algorithms that analyze patient symptoms and medical data. These algorithms identify patterns in symptoms and test results to predict patient outcomes with impressive accuracy. The system relies on a combination of supervised machine learning techniques, including linear classifiers that separate data points into different categories using linear decision boundaries.
The advanced algorithms detect conditions that physicians might otherwise miss, significantly improving patient outcomes. Take stroke cases as an example - the AI system analyzes brain scans before any human has even opened the file. When a brain scan is taken, the image goes directly to a server where programs like Viz.ai rapidly analyze it using neural networks to reach a preliminary diagnosis.
From there, the system automatically triggers a series of communications to alert everyone in the care chain—doctors, neurosurgeons, neurologists, and radiologists. This immediate notification allows healthcare providers to expedite care in time-sensitive situations, saving crucial minutes that often determine whether a patient recovers fully or suffers permanent damage.
Integration with Electronic Health Records
The seamless connection with Electronic Health Records (EHR) forms a critical component of Cleveland Clinic's virtual triage success. After evaluating symptoms, physicians can order diagnostic tests, prescribe medications, and document follow-up care instructions directly through Cleveland Clinic's electronic health record system. This integration gives providers comprehensive patient information, including medical history, lab results, medications, and demographics.
This integration offers several key advantages:
- Physicians receive valuable diagnostic insights based on symptoms, medical image analysis, lab results, and other data points.
- AI algorithms clean and manage diverse datasets, identify dynamic patterns to improve clinical care processes, and provide sophisticated algorithms for better outcome prediction.
- The system enables real-time viewing of patients' health by bringing together EHR, imaging, and genomics data for more accurate predictions.
Particularly impressive is how AI enhances EHR functionality through natural language processing and ambient listening technology. This technology helps physicians generate progress notes from conversations in exam rooms and instantly create draft responses to patient questions. Though still under development, initial testing shows that healthcare generative AI effectively translates free-text clinical documentation to discrete billing codes, streamlining the coding workflow.
Patient Satisfaction Metrics
Patient satisfaction with Cleveland Clinic's virtual triage system has been exceptionally high. Surveys show that 83% of patients report high satisfaction with their virtual interactions. This positive feedback stems from several factors, including minimal wait times—patients typically connect with a physician in less than two minutes.
Patients value having one-on-one time with emergency medicine physicians, even in a virtual setting. The ability to view test results and follow-up care instructions through Cleveland Clinic's MyChart app further enhances their experience. This accessibility not only improves convenience but also empowers patients by giving them greater control over their healthcare journey.
Virtual triage ensures patients receive care in the most cost-effective setting, benefiting individuals and insurance providers. With 24/7 availability, patients can use self-triage tools during weekends and evenings when traditional facilities might be closed or difficult to access. This availability proves especially valuable for people uncertain whether their symptoms warrant medical attention.
The success of Cleveland Clinic's virtual triage program shows the significant impact of AI in telehealth on healthcare delivery. By achieving 94% diagnostic accuracy while maintaining high patient satisfaction, the system demonstrates how artificial intelligence can improve both clinical outcomes and patient experience simultaneously.
Johns Hopkins' Telehealth AI for Chronic Disease Management
Johns Hopkins Medicine has developed sophisticated AI-enabled telehealth systems for chronic disease management that deliver remarkable clinical results. Building on its reputation for medical innovation, Johns Hopkins has created targeted solutions addressing healthcare's most pressing challenges: diabetes management, heart failure monitoring, and sustained patient engagement.
Diabetes Care Outcomes: HbA1c Improvements
The integrated digital health platform from Johns Hopkins featuring AI-driven dietary management shows impressive improvements in glycemic control. Patients using the platform experienced HbA1c reductions of 0.49% over 24 weeks and maintained improvements of 0.44% at 48 weeks. These results significantly outperformed control groups who saw minimal changes (-0.06%) or even worsening conditions (0.07% increase) over the same periods.
What makes this system particularly effective? Its simplicity. The AI requires a single photograph of each meal to provide immediate dietary feedback. This straightforward approach drives consistent usage—patients averaged 26.07 interactions weekly even after 48 weeks in the program. Johns Hopkins researchers confirmed that regular application use directly correlates with greater HbA1c improvements, validating the value of sustained engagement.
Personalized "nudges" form another cornerstone of the Johns Hopkins approach. AI algorithms analyze patient data to deliver tailored recommendations at optimal moments. Similar AI-powered reminder systems have shown a 3x increase in engagement, leading to additional 0.4-point reductions in A1c levels (from 8.2% to 7.8%). These personalized interventions improved medication adherence by up to 97% among enrolled patients.
Heart Failure Monitoring Results
Heart failure monitoring represents another area where Johns Hopkins has applied AI to telehealth with compelling outcomes. Their telemonitoring program significantly reduces mortality and rehospitalization rates among heart failure patients. Unlike traditional approaches requiring manual review of collected data, Johns Hopkins' system uses machine learning algorithms to automatically assess incoming patient data and identify those at the highest risk.
The system's effectiveness is clear from the numbers: in simulations, their machine learning model achieved an impressive 0.855 ROCAUC score, substantially outperforming conventional algorithms (0.727). The system continuously evaluates patient risk, with scores typically rising during the three weeks preceding heart failure hospitalizations—creating a critical window for intervention.
Perhaps most impressive is the efficiency gain: by reviewing only the highest-risk third of patients identified by the AI, clinicians could detect 95% of heart failure hospitalizations within the following seven days. This approach mirrors success seen at other institutions where similar remote monitoring programs reduced 30-day readmissions for heart failure by 50%.
Patient Engagement Statistics
Patient engagement statistics reveal the human impact of Johns Hopkins' telehealth innovations. The institution has developed a sophisticated "video visit technical risk score" integrated into its Epic electronic health record system. This color-coded rating identifies patients who might need additional support with telehealth technology, ensuring equitable access across different digital literacy levels.
Though initially focused on improving technological adoption, Johns Hopkins has expanded its engagement strategies to include:
- Conversational AI through voice assistants achieved 32% better insulin medication adherence and completed optimal insulin dosing 41 days faster than standard care (15 days versus 56 days)
- AI chatbots that maintain engagement rates exceeding 90% among enrolled patients
- Remote dielectric sensing systems that monitor lung fluid levels, providing early warning of potential heart failure exacerbations
Johns Hopkins researchers note that AI's most valuable contribution may be making continuous outpatient monitoring practical at scale. Unlike inpatient settings where alarm fatigue is common, their AI systems provide precise intelligence around incoming data, making it "clinically relevant, clinically meaningful and interpretable" within each patient's specific context.
The ongoing success of these technologies demonstrates how AI in telehealth can simultaneously improve clinical outcomes and patient experience while reducing provider burden—a critical combination for the future of telemedicine.
Mount Sinai's AI-Enabled Telehealth for Mental Health Services
Mount Sinai Health System stands at the forefront of applying AI-enabled telehealth specifically for mental health services. The institution's pioneering approach combines cutting-edge technology with evidence-based psychiatric care, resulting in measurable improvements for patients struggling with various mental health conditions.
Depression Screening and Treatment Protocols
Mount Sinai's implementation of AI technology for depression screening delivers impressive results. Their AI voice biomarker technology can detect signals consistent with moderate to severe depression with 71.3% sensitivity and 73.5% specificity when benchmarked against standard PHQ-9 scores. What makes this approach particularly valuable is its accessibility - this non-invasive screening method requires only 20-25 seconds of speech, making it considerably more accessible than traditional screening approaches that currently reach less than 4% of primary care patients.
In partnership with IBM Research, Mount Sinai launched the Phenotypes Reimagined to Define Clinical Treatment and Outcome Research (PREDiCTOR) study, funded by a $20 million grant from the National Institute of Mental Health. This groundbreaking initiative analyzes audiovisual data from clinical interviews, including spoken language, eye contact, and facial expressions, along with smartphone-collected behavioral data such as physical activity metrics, sleep patterns, and social interactions. The study specifically targets 15-30-year-old patients, a demographic experiencing rising mental health challenges.
The effectiveness of Mount Sinai's AI-based clinical decision support systems for depression treatment is clear from physician feedback. 86% of physicians report that the AI-enabled system provided a more comprehensive understanding of patient situations. Additionally, 71% of physicians felt the AI predictive model was useful when deciding treatment approaches.
Virtual Therapy Session Effectiveness
How effective is telehealth compared to traditional in-person therapy? The data provides a clear answer. Telehealth has demonstrated comparable effectiveness to in-person care for depressive disorders and superior effectiveness for anxiety disorders. This finding holds particular significance for clinical practice, suggesting telehealth may be especially well-suited for treating anxiety disorders in youth.
Patient engagement metrics show substantial adoption growth, with the average number of telehealth sessions per user jumping from 2.3 per year in 2019 to 8.7 per year in 2022. When researchers analyzed user feedback, they found that 68% of youth expressed positive sentiments toward telehealth services, with convenience (45%) and privacy (38%) cited as primary benefits.
The benefits aren't limited to younger populations. Among all patients, 62% reported improved care due to AI tools, and 46% reported somewhat or significantly improved physician-patient relationships. Equally important, patient trust remains high, with 62% of patients rating the AI tools as trustworthy.
Crisis Intervention Success Rates
Mount Sinai's telehealth crisis intervention capabilities show particular promise in suicide prevention efforts. Studies found telehealth interventions for suicide prevention to be well-accepted, with notably high retention rates. This success becomes even more critical when we consider that up to 20% of police calls involve a mental health or substance use crisis.
The effectiveness of virtual crisis care appears in concrete outcomes. In a related pilot program using similar telehealth approaches, out of 181 encounters predicted to result in involuntary committal, only 19 ended up requiring immediate inpatient care—a 90% reduction in unnecessary hospitalizations. What's particularly striking is that over half of these calls involved suicidal ideation, and 15% involved aggressive behavior.
AI risk modeling in this context involves analyzing complex patient data including previous diagnoses, medication history, healthcare utilization patterns, social determinants, and even real-time data from wearables. This comprehensive approach enables Mount Sinai to move beyond traditional first-come, first-served models toward prioritization based on clinical need, ensuring limited resources are allocated where they can have the greatest impact.
Materials and Methods: Data Collection Across Hospital Systems
How do we know AI telehealth systems work? To examine the effectiveness of AI in telehealth implementations across major hospitals, researchers established rigorous methodological frameworks and data collection protocols. These approaches ensure validity and reliability in evaluating health outcomes across diverse patient populations.
Standardized Outcome Measurements
What metrics tell us the true story of telehealth success? Researchers employed industry-standard measures, primarily Healthcare Effectiveness Data and Information Sets (HEDIS), which are widely used by hospitals, health systems, and government agencies including the Centers for Medicare & Medicaid Services for national quality reporting. The comprehensive evaluation frameworks included sixteen HEDIS measures across five domains of primary care:
- Cardiovascular care (including antiplatelet therapy, lipid panel, and β-blocker therapy)
- Diabetes management
- Prevention and wellness (including vaccination rates)
- Behavioral Health
- Pulmonary care
Throughout the evaluation process, the National Quality Forum (NQF) framework served as a foundation, offering a comprehensive guide for developing telehealth measures under four critical domains: access to care, financial impact, patient experience, and clinical effectiveness. This approach aligns with recommendations from the Agency for Health Research and Quality (AHRQ), which emphasizes including health outcomes impact in any telehealth evaluation.
Patient Demographics and Selection Criteria
Patient cohorts were carefully divided based on their exposure to telehealth services. Most studies classified patients into those with office-only visits versus those with telehealth exposure (either video-only visits or a blend of video and in-person care). This methodology allowed for direct comparisons of outcomes between traditional and AI-enabled telehealth approaches.
Given that the future of telemedicine depends on equitable implementation, researchers collected de-identified demographic data stratified by age, race, ethnicity, sex, and social determinants of health. This attention to demographic factors helps address potential equity gaps, particularly for families who cannot access telehealth due to technology resource limitations.
Selection criteria typically followed the Strengthening the Reporting of Observational Studies in Epidemiology (STROBE) reporting guideline for cohort studies. In one large-scale assessment, researchers analyzed 102,059 virtual primary care encounters over four months, adjusting for case mix to ensure diagnostic accuracy exhibited minimal variation across demographic characteristics.
Statistical Analysis Methodology
The research approach primarily used retrospective cohort study designs, extracting de-identified, secondary data from electronic medical records to compare quality performance across outpatient care sites. For many studies, this involved creating standardized data extraction forms to gather essential information covering study characteristics, specifics of telehealth interventions, measured outcomes, and key findings.
For AI-enabled telehealth systems specifically, researchers developed multifaceted machine learning approaches. These included:
- Supervised learning techniques (random forest and gradient boosting) to predict health outcomes based on telehealth usage patterns
- Logistic regression for more interpretable insights into factors influencing telehealth effectiveness
- Principal component analysis to identify key factors driving outcome variability
Propensity score matching proved essential in ensuring fair comparisons between telehealth users and non-users. Additionally, difference-in-differences analysis helped assess the impact of telehealth policy changes on health outcomes.
To evaluate patient perspectives regarding the use of AI in telemedicine, researchers conducted comprehensive literature searches across interdisciplinary electronic databases, including gray literature. For assessing patient satisfaction with AI-enabled telehealth systems, validated instruments like the Telehealth Usability Questionnaire, Telemedicine Satisfaction and Usefulness Questionnaire, and the Net Promoter Score were employed.
Model validation didn't stop at simple accuracy measures. Techniques included k-fold cross-validation, performance metrics such as AUC-ROC and F1 scores, and sensitivity analyses to assess the impact of critical assumptions and potential biases. Furthermore, researchers utilized SHapley Additive exPlanations values and Local Interpretable Model-agnostic Explanations to provide insights into model predictions and feature importance.
Comparative Analysis of AI Telehealth Implementations
Comparative analysis across major hospital AI telehealth implementations reveals consistent patterns of improvement in multiple domains. This systematic evaluation provides crucial insights into how AI in telehealth is reshaping healthcare delivery at scale.
Cost-Effectiveness Metrics
The economic impact of AI-enabled telehealth presents compelling value across all measurements. AI-driven remote patient monitoring has reduced hospital readmissions by up to 50%, generating substantial cost savings considering that a typical 3-day hospital stay costs approximately $30,000. The financial efficiency of these AI-powered interventions is remarkable, with some implementations cutting costs by USD 5.54 million compared to conventional approaches.
Looking at market projections, the global AI in healthcare market, valued at USD 19.27 billion in 2023, is expected to grow at a CAGR of 38.5% from 2024 to 2030. Within this broader trend, the US AI in telemedicine market specifically is forecast to expand from USD 6.7 billion in 2024 to USD 48.2 billion by 2033.
Clinical Outcome Improvements
When it comes to patient health, AI telehealth systems demonstrate significant improvements across multiple conditions:
- Diabetes management platforms achieve HbA1c reductions of 0.49% over 24 weeks.
- AI-driven screening for diabetic retinopathy reaches a sensitivity of 96.3% and specificity of 80.4% in optimal cost-effective implementations.
- Depression detection through AI voice biomarkers attains 71.3% sensitivity and 73.5% specificity.
Perhaps the most compelling evidence comes from chronic disease management, where continuous AI monitoring identifies high-risk patients days before clinical deterioration becomes apparent.
Staff Efficiency Gains
On the provider side, AI-enabled automation addresses burnout—a critical issue affecting nearly half of US physicians according to the American Medical Association. The impact on workflows is substantial: AI documentation tools reduce charting time by 72%, saving up to two hours per provider per day. What's more, AI-powered scheduling systems have improved patient flow and reduced wait times by over 80% in some clinics.
Patient Access Expansion
AI has dramatically expanded healthcare access, particularly for underserved populations. About one-third of Medicaid enrollees reported using telehealth services, with 63.3% stating they received care they otherwise would not have accessed. Patient satisfaction remains high, with 92.1% reporting that telehealth adequately addressed their health concerns.
The integration of AI chatbots for triage allows patients to begin their healthcare journey before connecting with providers. This approach determines appropriate care pathways while accelerating processes, making quality care available regardless of geographic location. Overall, AI-powered telemedicine has overcome barriers to healthcare access through its ability to provide remote consultations, enhanced by AI diagnostic support and continuous monitoring.
Future of Telemedicine: Next-Generation AI Applications in Development
The next wave of innovation in AI in telehealth extends beyond current applications toward technologies that anticipate needs, integrate multiple data streams, and blend seamlessly into clinical workflows. These emerging solutions promise to fundamentally alter how healthcare is delivered in the coming years.
Predictive Analytics for Disease Prevention
Predictive analytics is becoming essential to disease prevention by identifying patterns and trends that can anticipate future health events. This isn't just theoretical—it's already happening. Through analysis of patient data—including demographics, medical history, diagnostic tests, and treatment outcomes—AI algorithms generate predictive models with greater precision than traditional methods. Currently, AI systems analyze data from sub-Saharan Africa to predict malaria outbreaks by recognizing patterns in mosquito breeding conditions, allowing governments to take pre-emptive action.
The future looks even more promising. Predictive models will increasingly develop customized treatment plans based on patient's genetic profiles, lifestyle factors, and environmental backgrounds. This personalized approach will help physicians provide optimal treatments while minimizing side effects. The shift from reactive to proactive healthcare represents one of the most significant transformations in medical practice.
Multimodal AI Systems Combining Voice, Visual, and Biometric Data
Multimodal technology marks a significant advancement through integrating multiple forms of data inputs to process information. Recently developed models like GPT4Vision, Gemini, and Whisper can interpret and generate various types of content:
- Images and medical scans
- Voice and sound inputs
- Genomic data
- Biometric measurements
- Electronic health record notes
What makes these systems truly powerful? By combining these different inputs, healthcare providers gain comprehensive diagnostic capabilities and can create truly personalized treatment plans. There's an added security benefit too—multimodal systems create an additional security layer, as each biometric mode serves as an independent verification step. This approach proves particularly valuable in healthcare environments where patient identification must be both accurate and efficient, especially during emergencies.
Ambient Clinical Intelligence
Ambient clinical intelligence (ACI) might be the most transformative technology for day-to-day clinical practice. By combining AI and voice recognition to automate documentation, this technology captures patient encounters during doctor-patient conversations, generating detailed clinical notes while physicians focus on patients rather than computers.
The adoption numbers tell an interesting story. Already, 968 physicians have enabled ambient AI scribes in at least 100 patient encounters each, with one physician using it for 1,210 encounters. The feedback is overwhelmingly positive—physicians report that this technology facilitates more meaningful patient interactions while reducing after-hours clerical work.
Development continues at a rapid pace. Ongoing enhancements focus on direct EHR integration, improved capabilities for incorporating medical interpretation, and enhanced workflow personalization. As these systems mature, they'll likely become as fundamental to healthcare as the stethoscope once was.
Limitations of Current AI-Enabled Telehealth Systems
Despite the impressive advances in AI in telehealth, significant obstacles still stand in the way of widespread implementation. These limitations affect multiple aspects of telehealth delivery and require systematic approaches to overcome before we can realize the full potential of these systems.
Technical Infrastructure Challenges
What's holding back AI telehealth adoption on the technical front? Infrastructure and technical barriers represent the most frequently reported obstacles, with a relative frequency occurrence of 6.4% according to high-quality evidence. These challenges include insufficient network capabilities, lack of existing technologies, and limited device availability.
Rural and countryside regions face particularly severe technical hurdles, where connectivity issues and inadequate access to electricity create implementation barriers. Though telemedicine systems can relay data over large distances, device outages occur periodically.
The problems extend beyond basic connectivity. Technical challenges also include compatibility with daily workflows, interconnectedness difficulties, and the absence of standardized systems across different facilities. These interoperability issues mean that even when the technology works, it might not integrate smoothly with existing healthcare processes.
Healthcare Provider Adoption Barriers
Technology means nothing if healthcare professionals don't use it. Provider resistance represents a significant hurdle, with psychological and personal issues related to AI telehealth adoption showing a relative frequency occurrence of 5.3%. Additionally, concerns about increasing working hours or workload account for 3.9% of adoption barriers.
What specifically drives this reluctance? Resistance to change, difficulty understanding technology, technophobia, and perception of decreased human interaction all contribute to hesitation among providers. Trust and reliability remain major barriers, as many patients may not trust an AI device as much as they trust their general practitioner.
Healthcare staff often struggle with technical literacy; indeed, technically challenged staff was identified as the largest obstacle to telemedicine adoption. High-quality, real-time technical support appears crucial for increasing provider efficiency and decreasing implementation fears.
Regulatory Compliance Issues
The legal landscape presents perhaps the most complex set of challenges. Regulatory frameworks currently struggle to keep pace with AI in telehealth innovations. Privacy and security concerns represent significant limitations, alongside unclear legal liability regarding response protocols.
The expiration of the Public Health Emergency in May 2023 created a regulatory dilemma, with lawmakers unprepared to make decisions about long-term telehealth policy. Previously, HIPAA flexibilities waived requirements for HIPAA-compliant telehealth platforms during the pandemic.
Currently, regulatory complexity effectively obstructs providers from using telemedicine, with state-specific license requirements creating peculiar situations like the "telemedicine parking lot phenomenon" where patients drive to locations within provider states to receive telehealth services. These regulatory inconsistencies create an uncertain environment that discourages investment and adoption of AI telehealth solutions.
Conclusion
AI-enabled telehealth systems demonstrate remarkable success across major U.S. hospitals, transforming healthcare delivery through measurable improvements in patient outcomes and operational efficiency. Mayo Clinic achieved a 40% reduction in hospital readmissions, while Cleveland Clinic reached 94% accuracy in virtual triage. Johns Hopkins proved the effectiveness of AI-driven chronic disease management, particularly for diabetes and heart failure patients. Mount Sinai's mental health applications showed significant progress in depression screening and crisis intervention.
The financial impact of these implementations is equally impressive. AI-powered remote monitoring reduces typical hospital stays and generates millions in cost reductions. On the clinical front, healthcare staff report significant efficiency gains, saving up to two hours daily through AI documentation tools. Perhaps most importantly, patient satisfaction remains consistently high, with over 80% reporting positive experiences across various telehealth platforms.
Current systems aren't without their challenges. Technical infrastructure limitations, provider adoption barriers, and regulatory hurdles create obstacles to wider implementation. Yet the path forward looks promising. Next-generation applications will enhance capabilities through predictive analytics, multimodal AI systems, and ambient clinical intelligence. These advancements will enable healthcare providers to deliver more personalized, proactive care while maintaining the human touch essential to medical practice.
The evidence paints a clear picture: AI-enabled telehealth represents a fundamental shift in healthcare delivery, offering improved access, better outcomes, and reduced costs. Success stories from leading hospitals prove that thoughtful implementation of AI technology creates measurable benefits for patients, providers, and healthcare systems. As these technologies mature and adoption barriers fall, we can expect AI in telehealth to become an increasingly vital component of modern healthcare delivery.