Automating Financial Reports with AI for Efficiency and Accuracy
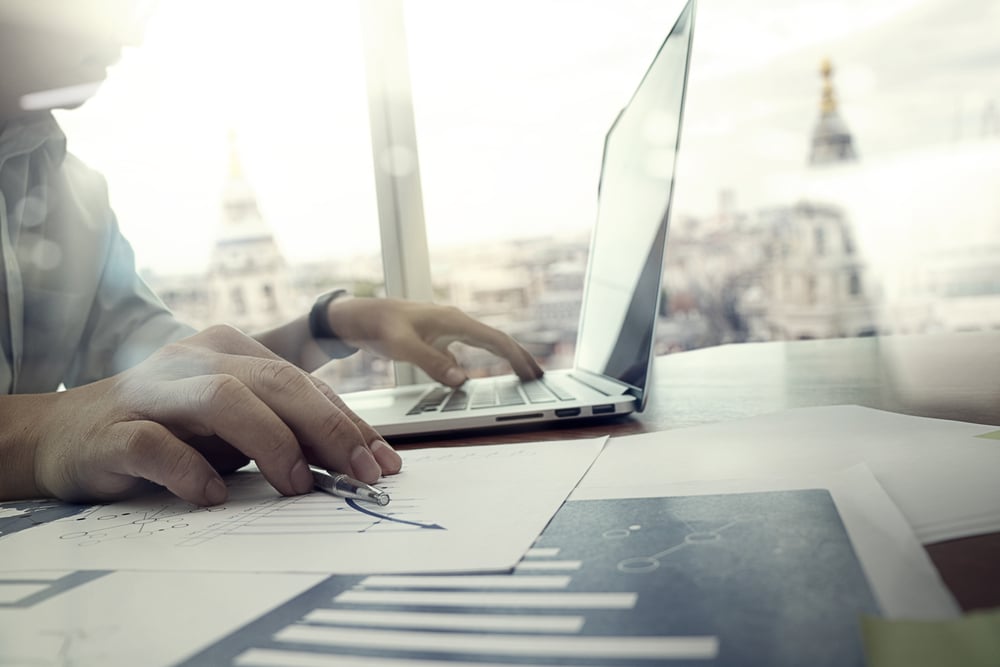
Preparing the reports manually requires significant time and effort from finance teams to gather, consolidate, and analyze data from various sources. As businesses grow and generate more data, the task becomes increasingly difficult to scale.
Manual reporting often focuses on basic financial metrics, such as balance sheets and income statements, while overlooking the potential insights hidden within the data. This limits the ability and increases time and resources needed to perform in-depth analysis and uncover valuable patterns, trends, or anomalies that could inform strategic decision-making.
AI technologies, such as machine learning and natural language processing, can automate data collection, analysis, and report generation processes.
AI techniques used in automation of financial reports
Machine learning
Machine learning algorithms are widely applied in financial data analysis. They can automatically learn from historical data, identify patterns, and make predictions or classifications. ML techniques such as regression, decision trees, random forests, and support vector machines can be used for tasks such as credit risk assessment, fraud detection, market trend analysis, and portfolio optimization.
Natural language processing (NLP)
NLP enables computers to understand and interpret human language. In financial data analysis, NLP techniques are utilized to extract insights from unstructured data sources like news articles, social media feeds, and financial reports. Sentiment analysis, topic modeling, named entity recognition, and text summarization are some NLP applications used to analyze financial sentiment, news sentiment, and market trends.
Deep learning (DL)
Deep learning is a subset of machine learning that utilizes artificial neural networks inspired by the human brain. Deep learning algorithms, such as convolutional neural networks (CNNs) and recurrent neural networks (RNNs), are capable of handling complex financial data, such as images, time series data, and sequences. DL techniques are used for tasks like image recognition, time series forecasting, fraud detection, and algorithmic trading.
Data mining
Data mining techniques aim to discover hidden patterns and relationships within large datasets. These techniques, including clustering, association rules, and anomaly detection, can be applied to financial data analysis to identify customer segmentation, detect market trends, find correlated financial indicators, and discover abnormal behavior or fraud patterns.
Predictive analytics
Predictive analytics involves using historical data and statistical modeling techniques to make predictions about future outcomes. By leveraging algorithms like regression analysis, time series forecasting, and ensemble models,predictive analytics can provide insights into stock prices, customer behavior, loan defaults, market trends, and other financial predictions.
Robotic process automation (RPA)
RPA involves automating repetitive tasks using software robots. In financial data analysis, RPA can be used to automate data collection, data preprocessing, and report generation processes, reducing manual effort and improving efficiency.
Algorithmic trading
It involves using AI algorithms to automate trading decisions, executing trades based on predefined rules and market conditions. Machine learning models, reinforcement learning, and statistical arbitrage are utilized to develop trading strategies that analyze market data, identify patterns, and optimize trading execution.
What can you achieve with AI in finance?
- Data extraction and aggregation: AI algorithms can help extract data from multiple sources, such as accounting software, spreadsheets, and databases, and aggregate it into a centralized system. This eliminates the need for manual data entry and reduces the chances of errors or inconsistencies.
- Data cleansing and validation: An AI tool can support in automatically cleaning and validating financial data by identifying and correcting errors, inconsistencies, or missing values. This ensures the accuracy and integrity ofthe data used for generating reports.
- Automated report generation: AI-powered systems can generate financial reports automatically based on predefined templates and formats. These systems can extract relevant data, perform calculations, apply accounting rules, and produce comprehensive reports without human intervention.
- Data visualization: Those responsible for financial reports can use AI in automating data visualization, enhancing the presentation and interpretation of financial data. For example, charts, interactive dashboards, and personalized visualizations can be created.
- Customizable report templates: Businesses can create customizable report templates, enabling businesses to tailor reports to their specific requirements. Users can define the structure, layout, and metrics to be included, ensuring that the reports align with their unique reporting needs.
- Real-time reporting and alerts: AI enables real-time monitoring of financial data and the generation of reports on demand. It also allows for automated alerts and notifications based on predefined thresholds or specific events, ensuring that stakeholders receive timely information for decision-making.
- Fraud detection: Anomaly detection can be achieved by analyzing large volumes of financial data, detecting anomalies and patterns that may indicate fraudulent behavior. By establishing baseline patterns and comparing new data against them, AI-powered systems can identify suspicious transactions, unusual spending patterns, or discrepancies that may warrant further investigation.
Benefits of automating financial report generation
Automating financial report generation offers numerous benefits to businesses. Firstly, it significantly reduces the time and effort required to compile and create reports, enabling finance teams to allocate their resources to more strategic and value-added activities.
Secondly, automation minimizes the risk of human errors and inconsistencies by extracting data directly from financial systems, ensuring accuracy and reliability in the reports.
Additionally, automated report generation allows for increased agility and flexibility, as reports can be generated on-demand or scheduled to run at specific intervals, providing real-time insights for decision-making. This streamlined process enhances
- Overall efficiency
- Improves data integrity
- Empowers organizations to make informed financial decisions in a timely manner.
Automation of financial report generation can bring scalability benefits to businesses. As organizations grow and generate increasing amounts of financial data, manual report generation processes become arduous and time-consuming.
Conclusions
With automation, businesses can easily handle larger volumes of data and generate reports efficiently, regardless of the size or complexity of their financial operations. Automating financial report generation using AI not only enhances efficiency and accuracy but also enables businesses to unlock valuable insights, streamline processes, and scale their operations effectively.