Data Mining versus Machine Learning – What Is the Difference?
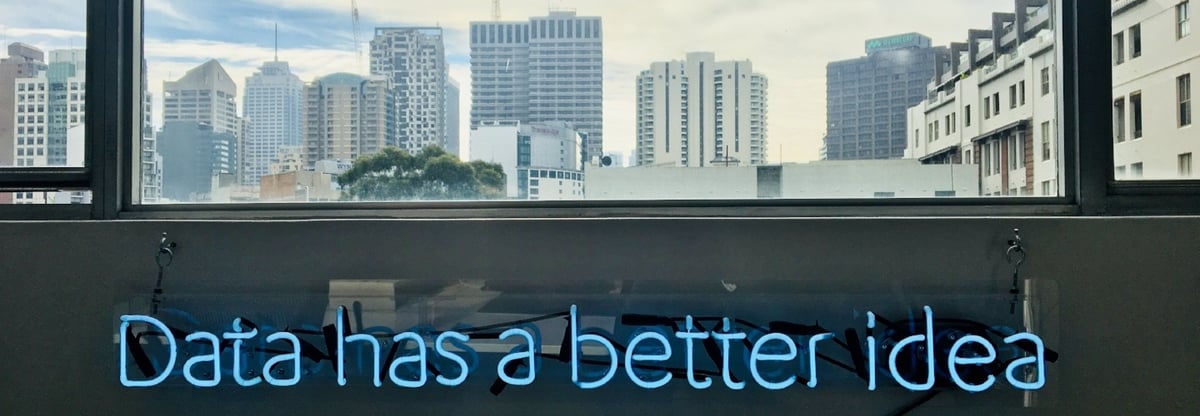
Moreover, what differs data mining from machine learning is the approach to data analysis as well as their different applications.
It’s no secret that big data and advanced analytics are changing the face of the business world. Insights gained from big data are revolutionising foundational business processes such as sales and marketing, supply chain management, and R&D; giving companies the information they need to increase revenues, reduce costs, and improve customer experience.
Despite this prevalence of data science in the business arena, there is still much confusion around the terminology used to define the analytical processes involved. In particular, the terms data mining and machine learning are often used interchangeably, and while they share similarities, they are not the same.
So what is the difference between data mining and machine learning, and what part do they play in the big data revolution? To answer this question, let’s first take a look at the definitions of each.
Data mining versus machine learning: The definitions
Data mining is the process of analysing vast amounts of data from various sources to extract useful information. This is done through the discovery of previously unknown patterns, correlations, and anomalies, which can then be used to predict future outcomes.
A sub-domain of artificial intelligence, machine learning, takes things further by using algorithms and an iterative process to learn from new data and automatically become better at analysis and prediction. It can do this without the need for human intervention.
What are the similarities between data mining and machine learning?
It’s easy to see how the concepts of data mining and machine learning have become confused. Both share a foundation in data science, and there certainly is overlap between the two. Data mining can use machine learning algorithms to improve the accuracy and depth of analysis, and vice-versa; machine learning can use mined data as its foundation, refining the dataset to achieve better results.
You could also argue that data mining and machine learning are similar in that they both seek to address the question of how we can learn from data. However, the way in which they achieve this end, and their applications, form the basis of some significant differences.
Different approaches to analytical process
The most obvious contrast between data mining and machine learning is their approach to data analysis. Data mining allows analysts to pull together and interpret vast amounts of structured or unstructured data, but it does not drive any processes by itself. Machine learning, on the other hand, can learn from new data and become more intelligent, without being programmed.
For example, data mining can be used by e-commerce retailers to identify which products are frequently bought together, allowing them to recommend complementary products when a customer makes a purchase. Machine learning, however, goes deeper and can learn from customers’ buying habits to improve its ability to recommend products, and through an iterative process, becomes better at doing so over time.
Distinct applications of machine learning and data mining
Another key difference between data mining and machine learning is what they are typically used for.
As well as the e-commerce example above, data mining is widely used in retail to determine sales trends and customer purchase patterns, allowing companies to forecast sales and optimise their marketing efforts. Businesses also use data mining for market analysis and to guide financial planning decisions, while financial institutions use it to help understand market risks, identify investment opportunities, and detect fraud faster.
Machine learning can provide more accurate insights, often in real time, which makes it ideal for rapidly detecting and preventing fraud, protecting both banks and retailers. It is also revolutionising sales and marketing, with businesses now able to personalise a customer’s shopping experience based on their purchase history. Similarly, many companies are now using AI to improve their customer service function, with capabilities such as automatically responding to typical email requests, AI chatbots, and routing customers to the right department.
The impact of machine learning and data mining on business
The digital universe is growing at an astounding rate and data analytics are set to impact all areas of our lives. While we have seen data mining versus machine learning, particularly around their approaches to data analysis and their applications, it is possible that we will see more overlap as the two are used in combination to improve the usability and predictive capabilities of vast quantities of data in the business world.