14 Key Trends in Data Science for 2021
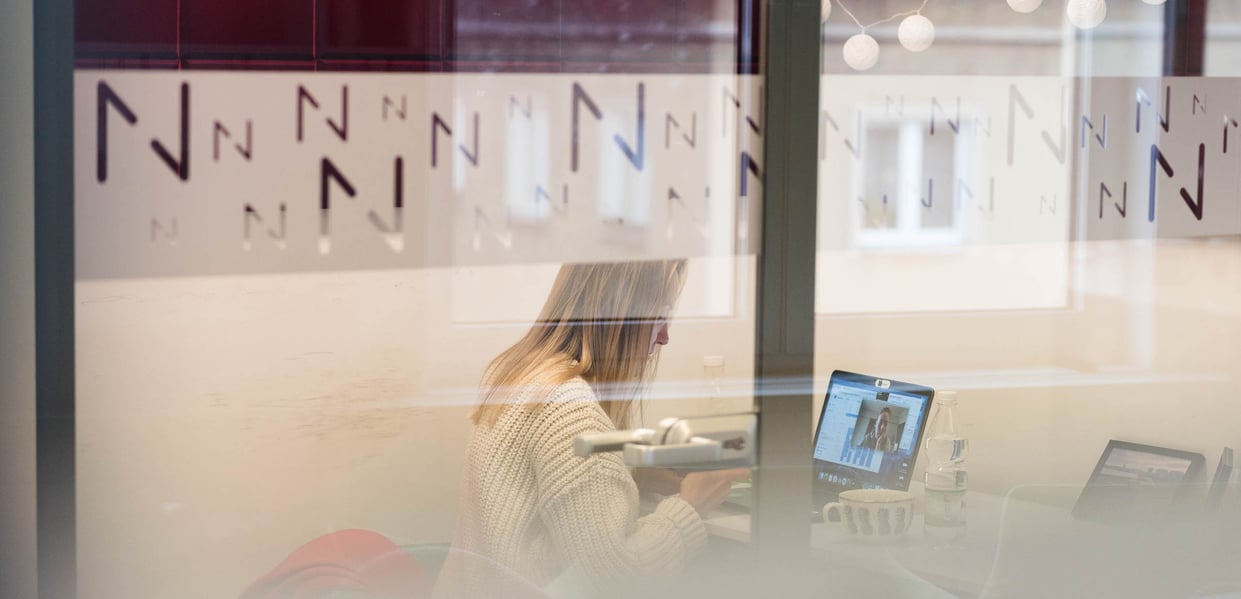
With a greater reliance on online services and e-commerce, data looks very different from how it did in the pre-pandemic world, and using it properly has never been more important for your business.
2021 is therefore a year of catching up, adapting to new data science tools, and rethinking how data is captured and analyzed.
Businesses of all sizes are investing heavily in hiring data analysts and data scientists to make sense of the new digital world and work out how they can take advantage of it by adapting their business processes. These data analysts will revolutionize the visualization and application of the data they analyze, making it accessible and understandable for employees at all levels.
The latest trends in data science suggest that a new viewpoint is taking shape. Data is no longer a science reserved for a select group of specialists but has instead become an invaluable opportunity for every professional within a business to improve and refine their practice.
1. Scalable AI and “small data”
Covid-19 has heavily disrupted the types of data that have been available for analysis, and therefore the way that data can be used.
With more people online, there is a broader range of data available to be analyzed, but this data is very different from more historical sets of ‘big data.’ For this reason, ‘small data’ AI techniques are taking precedence, based on smaller incidents of customer behavior.
Artificial Intelligence (AI) must therefore be scalable in response to this, despite the well-known fact that large sets of data are historically better at making accurate predictions.
Machine learning must also adapt to the new restrictions to analysis that come as a result of greater online activity. New privacy rules, such as the California Consumer Privacy Act of 2020, mean that this focus on ‘small data’ is likely to stick around and broader ranges of historical data will be harder to access.
2. Data fabric
The need for a unified foundation on which to build and store the composable data and analytics of each business has increased with the complexity of data and its potential value.
Using data fabric as the central architecture facilitates the effective cohesion of hardware and software, allowing access across a range of locations both internally and externally without breaking data privacy laws.
Pre-existing data lakes, hubs, and warehouses can be woven together with new software tools and approaches, revolutionizing data governance for individual businesses. As a result, less integration and maintenance is required, allowing businesses to more quickly provide effective updates to the customer experience.
3. Data provenance concerns
With the rise of AI and deep fakes in advertising, the quality and reliability of data are now being called into question more than ever. When analyzing data for marketing or financial purposes, one of the biggest initial hurdles is deciding whether the data can be trusted.
Untrustworthy data can lead to inaccurate predictions or recommendations, so the capabilities of machine learning have developed as a result of this, and can now provide enhanced data quality. More sophisticated algorithms are required to interpret big data from broader periods of time to separate the false data from the truth; see Intelligent Feature Generation for more details.
4. Cloud computing
The move to cloud-based data storage has been a point of contention for many businesses who enjoy the security of local servers and view the cloud only as a tool for transactions, as was its original purpose.
However, with the rapidly accelerating developments in cloud technology, new data science trends have attracted many businesses to rethink their data storage. Providers such as Amazon, Microsoft, and Google are now the prime way for businesses to store their data and offer built-in analytics that help streamline the data management process.
According to Gartner, by the end of 2022, 90% of data and analytics innovation will require public cloud services, and, within a year of that, cloud-based AI will be five times as prominent as it was in 2019.
Another caveat to cloud-based data is homomorphic encryption, which means that computational calculations and analysis can be performed on data without decryption; therefore keeping your data even more secure and removing the need for the holder of the decryption key to be in the same location as the data. Using Cloud-based solutions minimizes the risk of bugs and errors, as the services are used widely and matured over the years of maintenance.
5. Augmented analytics
Going hand-in-hand with cloud-based data is the trend of augmented and user-friendly analytics. While it was previously necessary for trained specialists to interpret and evaluate data, employees at any level are now able to do so thanks to integrated data technology.
The rise of Internet of Things (IoT) devices ensures that every employee is in possession of a smart device that is capable of processing data of some kind. Employees from different departments are able to share and compare data and come up with solutions and ideas that will benefit everyone via predictive analytics and trend forecasting.. This analytics trend marks a move towards universal access to analytics in conjunction with a sharper focus on the specific needs of different business departments, business types, and individual employees.
Instead of relying on the opinions and experience of a select handful of specialists and a set of predetermined general questions, businesses can benefit from the varied, experiential viewpoints of all of their employees, who will be specifically tuned into the particular workings of their department.
Gartner predicts that the next step in this transition will be easy-to-use mobile dashboards that combine the functionality of older dashboard systems with a new utilization of all employees at every level. Employees will each have their own personalized dashboard with insights that are tailored to their roles, spreading a greater amount of useful knowledge to a wider range of people.
6. Python instead of R
While data and analytics have historically used R as their primary coding language, the shift towards a more user-friendly focus has led to a greater focus on Python.
Not only is this coding language a suitable all-rounded for a range of business types, but it is also known for requiring significantly fewer lines of code to achieve the same goals as R. As a result, it is a lot simpler to pick up, and therefore more accessible to those who have less coding experience.
Python also has the automatic ability to associate matching types of data, which can prove invaluable for streamlining data analysis.
The diversity of Python also ensures that all your data analysis can be written in the same language, from machine learning models to blockchain applications.
Free data science and machine learning libraries can assist in putting together meaningful predictions and actionable plans without the time-intensive data mining that was once necessary. In addition, Python provides easy integration with existing software, whereas R is more of a closed ecosystem.
7. Increased AI automation
With the fast-paced development of AI technology and capability, the amount of usable and translatable data has increased exponentially.
This is in part due to new automated processes and machine learning solutions that happen before the data reaches the analyst. Computers are getting better at understanding natural speech patterns, actual human queries, and the relationships between different words and meanings.
Deep learning allows machines to operate in a way that mimics the neurons of the human mind. This means that what was once a complex network of data has now become useful and actionable almost immediately, often in real-time.
Data is now taking the form of actionable human stories at an incredibly fast rate, helping businesses to set, define, and achieve their goals sooner.
One of the reasons for this rapid advancement in AI understanding is the sheer number of IoT devices that are now in circulation. With the help of the ethical limitations of so-called responsible AI, data has never been more available to collect, and, by extension, to learn from.
8. Customer personalization
In 2020, the pandemic drew many more consumers to the internet than ever before. The necessity of working from home meant that customers became reliant on a smaller range of devices, allowing each device to paint a more accurate picture of each consumer’s life.
The importance of each individual consumer became more important, with businesses placing increased value on the lifetime prospects of each customer and defining the moments of greatest value within that trajectory.
For this reason, in 2021, there is a greater need for more personalized experiences and journeys for the consumer.
Data science will focus on pinpointing the right moment and the right platform on which to capture a potential customer and bring them on board. After this, a customer must be kept happy and engaged to encourage a better relationship and a higher lifetime value.
9. Real-time data
One of the biggest new capabilities of data analysis in 2021 is real-time automated testing. This signals a move away from historic data that is by definition out-of-date. Companies can now engage with customers of their product or service more effectively, reacting to customer actions as they happen rather than reviewing the data at a later date.
According to Seagate, by 2025, 75% of the world’s population will have an interaction with data every 18 seconds, making it essential to increase the speed of data analysis and the subsequent reactions.
10. Graph analytics
Graph technologies have been used as a way to explain and interpret data since its foundation. They enable useful collaboration between different users and departments; and, with the increased focus on AI, machine learning, and automated data analysis, the importance of finding new applications has never been more apparent.
Graphs are an effective way of drawing parallels and similarities between audiences and products without having to translate the data into code beforehand, thus cutting out a time-intensive part of the data analysis process.
With so much new data now available, thanks to real-time analytics, countless IoT devices, and a greater engagement online, separating the anomalies in data is essential for drilling down on actionable trends. Graphs can help companies see the bigger picture of the data they have collected.
The results of automatic processes such as machine learning analytics can be refined through the use of a graph, to distinguish those valuable sets of data from those that are less helpful. The preparation of data for analysis is ultimately streamlined and simplified when processed by graph databases.
11. Intelligent feature generation
As machine learning is now a valuable and vital part of sophisticated data analysis, developing intelligent features for each unique case is essential for enhancing the overall accuracy of the machine learning models.
Features are determined by what is considered most important to the individual data sets and/or business.
Examples include measuring the distance between the peaks and troughs in a set of data (to potentially detect any defects or problems), creating simplified queries to stand in for more complex coded queries, and building sets of facts and data into scenarios that require certain reactions (if a certain scenario is perceived by the AI, then a certain reaction will be triggered).
12. Blockchain data analytics
Blockchain was once associated almost exclusively with cryptocurrency. One of the key features that makes it so attractive as a means of tracking transactions and currency is the near impossibility of manipulating it.
The computer processes that would be required to alter the blockchain once it has been written are so energy-intensive that the practicality of it is infeasible. For this reason, the transparency of blockchain is a reliable method of tracking data, even beyond the realm of cryptocurrency.
Data analysts have been quick to explore the potential of blockchain to cure the data provenance concerns mentioned above and provide even more accurate, reliable predictions than was previously possible.
In addition, blockchain seamlessly integrates with the new cloud-based systems that have rapidly been replacing hardware storage and can use data straight off the edge of IoT devices.
13. From DataOps to XOps
Gartner has predicted that a more effective way of dealing with DataOps is to expand it to XOps, facilitating the collaboration between data science, machine learning, AI governance, and AI platform management.
By combining all of these processes together, businesses can work towards a more complete end-to-end AI automation, from edge computing and data architecture through to managing the AI endpoints.
XOps encourages collaboration between the teams that deal with collecting and visualizing data, implementing intelligent feature generation, and then producing useful action plans, eventually refining the production line leading up to the final point and saving a great deal of time and power.
14. Solution to the explainability crisis
The explainability crisis draws together all the new data science trends for 2021. The key trends mentioned on this list amount to one significant trend: processing data into a form that can be understood by all employees of the business they relate to.
The primary way that this is being achieved is through augmenting traditional statistical models with rule-based formalisms and logic. Currently, most data is categorized after analysis, particularly for those in the financial sector that deal with risk.
Solving the explainability crisis will mean that each decision made by AI in this respect comes with an explanation of why the data has been categorized in this way.
The answer comes from a combination of greater semantic and linguistic understanding, a more human logic-based rule system, and an effective network of intelligent features that have been tailored to the business type.
Data science trends for 2021 and beyond
The advancements in data science in 2021 mark the beginning of a digital transformation in the fields of data, machine learning, and AI capabilities.
Data has never been more accessible and valuable to businesses of all sizes, with revolutionary data technologies now available. The data industry trends listed here give us insight into the market’s new key priorities: automation, accessibility, and intuition.