AI and Design Thinking: Traditional vs AI Design Processes

It’s now transforming traditional design thinking and design processes, too.
While it excels at tasks like sentiment and data analysis or user story generation, you can only see AI’s full potential and creativity when paired with human work and diverse datasets.
What I believe to be most interesting is how GenAI affects the daily work of product development teams.
In this piece, I examine the differences between traditional design thinking and design thinking AI.
Understanding traditional design thinking
Design thinking is a strategic method for innovation. This flexible, iterative process helps teams to understand user needs, challenge assumptions, identify problems, and create solutions to prototype and test. It’s handy for tackling complex or ill-defined problems. Typically, it involves five phases:
- Empathize: Research your users' needs to gain deep insights, without making any assumptions beforehand
- Define: Gather and analyze all your research to create clear problem statements and user personas
- Ideate: Run brainstorming sessions to come up with several potential solutions to the problems you’ve identified in the earlier stage
- Prototype: Build low-cost, experimental versions of products to explore potential solutions
- Test: Engage real users to evaluate prototypes, refine solutions, or revisit problems as necessary.
The evolution of design thinking in the age of AI
The stages mentioned above are not linear but iterative, ensuring a comprehensive understanding of user needs and solution development. This dynamic approach promotes creativity and innovation within product teams.
Originally, design thinking followed a model known as the ‘Double Diamond’. It was even-handed, devoting equal attention to each step in the process, as you can see below. It circled around finding divergent and convergent ways of alternating between all stages:
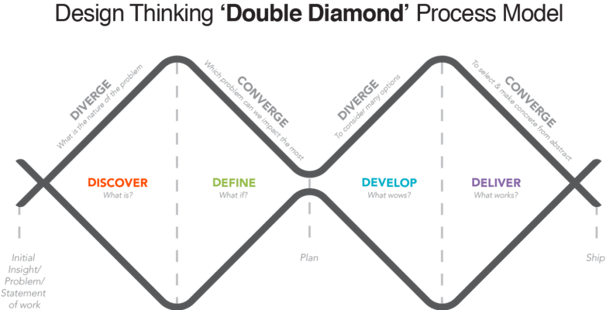
In this methodology, the first of the two diamonds includes two phases:
- Discover, where you take the defined insight, problem or statement of work, and collect information regarding users impacted by the issue.
- Define, in which you turn to insights from the discovery phaseand define the main problem, identify user personal, and create a brief.
Meanwhile, the second diamond begins in the ‘planning’ stage. It covers two steps:
- Develop, which is when you build a proof of concept based on your user personas, and test out your ideas’ viability.
- Deliver, where you develop the solution to the problem and seek feedback from the market.
However, once AI-powered technologies came into play, a discussion arose around whether this process should be modified. Among others, the design community suggested that programmers could develop an AI model by training it with design-related data and fine-tuning them based on performance. Still, it wouldn’t be able to do unsupervised work.
Areas like iterative testing and fine-tuning would still require significant human oversight to ensure relevance and accuracy.
Additionally, the ‘Develop’ stage could now also be completed faster than previously by using AI-driven platforms like Figma, which help designers quickly create and modify design elements.
To give you a sense of what ‘quick’ means in the context of proof of concept, I’d like to refer to an example of a project we rolled out at Netguru. We were able to build a text-to-speech app using the Bubble.io solution in just 25 minutes.
The Double Diamond model, shown below, is owned by the Board of Innovation and should be considered an experiment, not an industry standard. While different in some aspects, it still resembles the original double diamond (DD) shape, as shown in the image below. The AI begins by trimming unnecessary parts or those with the least desirable outcomes. At each stage, humans can intervene to guide further refinements.
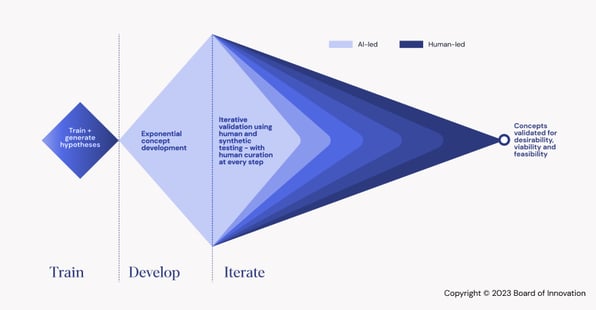
The ‘Iteration’ stage involves repeated cycles, where product team members gradually reduce the AI's influence with each round.
They make adjustments to achieve the final goal, balancing three key aspects: feasibility, desirability, and viability. This ensures the outcome is practical, appealing, and financially sustainable.
Impact of technological advancements on the design process
Traditional design thinking has always been valued for its lasting effectiveness in solving problems. However, with the birth of GenAI, there’s been a significant shift towards integrating LLMs into these processes.
Employing AI design thinking offers many advantages to businesses. It enhances their comprehension of user needs, pinpoints issues, generates ideas, prototypes solutions, and conducts more efficient testing.
AI enriches the design thinking process with advanced data analysis, pattern recognition, and predictive capabilities. These abilities help teams navigate complex challenges more efficiently.
I believe AI's capability to quickly analyze large datasets uncovers important patterns in user behavior, offering deeper and more accurate insights into what customers want. It also provides that ‘edge’ that lets brands stand apart from competitors.
The AI design thinking process
AI’s integration into design thinking can be visualized using our previous chart, but with these modifications:
- Empathize: AI-powered user research and sentiment analysis
- Define: Data-driven problem definition and identification
- Ideate: AI algorithms for solution generation
- Prototype: Rapid prototyping powered by AI tools
- Test: Utilizing AI for feedback-collection and analysis
Let’s look at how the design process plays out across all stages.
Key stages of the AI design process
Data collection and analysis
Some hypotheses suggest that traditional research is becoming unnecessary due to the vast amount of data available. AI can predict user needs and behaviors, essentially performing the entire discovery phase for us.
Using AI for data collection allows us to automate tedious tasks, such as transcribing user interviews, or integrate case-specific insights by analyzing data from other projects.
Discovery can also focus on competitive analysis, evaluating the strengths and weaknesses of competitors. It should explore viable technologies, assessing their potential to be integrated in ways that address and enhance user needs.
Model training and development
As mentioned earlier, AI tools might operate on training data which doesn’t ideally reflect your go-to user personas or the target market. So, it’s important to ‘calibrate’ it through experimentation and comparing AI and human output. If you see that the results don’t fully meet your expectations, then you’ll need to feed it with the right data so it can learn and improve over time.
Some organizations, such as the Board of Innovation, are already testing AI-powered discovery processes. These AI tools have achieved accuracy scores as high as, or even higher than, those created entirely by humans.
AI-driven ideation and prototyping
Here, we provide initial ideas which the AI can then develop further. Currently, AI can automatically generate a set of wireframes for multiple ideas, resilient enough for testing, particularly in the early stages of idea generation.
When faced with choices, such as deciding between 'A' or 'B', we've traditionally used sketch prototypes, pen and paper, or user stories for testing. Now, you can either transform your hand-drawn ideas into digital designs using tools like Sketch2Code, or even generate digital, low-fidelity prototypes fully with AI.
For more advanced work, designers can use no-code platforms like the above-mentioned Bubble.io to quickly create interactive, high-fidelity prototypes.
Continuous testing and improvement
You can use AI tools to conduct A/B tests, allowing designers to compare the performance of different design variations and identify which elements best achieve user goals. Additionally, designers can optimize designs for accessibility, tailoring products to meet the needs of users with varying abilities.
Comparing traditional design thinking and AI design processes
Training the modern model is more efficient than the DD model’s ‘Discover’ portion. Once the AI takes over concept development, the process is quick. The results are validated with both human and synthesized testing. Finally, you get a finished product with results similar to those achieved by lean start-ups using agile planning.
Paradoxically, in 2024, when you're delivering an AI driven solution, the lean approach is seen as ‘hot’ again, because you need to deliver and test as soon as possible. It's interesting when we take a look back and recall that the lean startup methodology was flagged as ‘dead’ 3-4 years ago. This was an oversimplification from the community. Lean principles have always been valuable and evolve continuously, especially now that we have access to AI.
The overlap of Lean and AI lies in continuous testing, where AI can collect real-time user feedback, run A/B tests, and create prototypes, while designers oversee its work and ensure human empathy and creativity.
Addressing key challenges in design thinking AI
Eliminating bias
The reason AI systems are biased is because they are trained on data created by humans. And the reason why we operate on stereotypes is because our brains wouldn’t be able to function without them due to the amount of everyday stimuli.
When we recognize that both humans and AI are biased, we can leverage AI to our advantage.
Despite its imperfections, AI outperforms humans in tackling complex problems, creating new opportunities.
We can train it on diverse datasets making sure they’re representative of our target audience, eliminating a partial approach as much as possible. Secondly, though bias is there in the database, we can prompt the AI correctly so that it is aware of it and tries to work around it.
Furthermore, you must run regular audits – as new data comes in, bias can easily creep in. This should be done by a human evaluator, who’ll be responsible for spotting and addressing any biased results.
Securing buy-in for AI output
I think one of the biggest challenges with AI is securing stakeholder buy-in. Even when it offers objective data, decision makers want to hear from a human before making a decision. What I suggest is organizing workshops with stakeholders to show AI capabilities and gather their input. It’s also worth involving them in prototyping sessions, so they can see AI in action and share feedback.
Involving stakeholders in the design process is the only way to make sure that they understand, trust, and support the decisions that the team makes. If we skip the human factor, there’s a risk that those who have the final say will refuse to use the solution – even despite evidence and “hard data”.
I also recommend building proof of concept by sharing case studies and success stories of AI-driven projects. Alternatively, you could also build a small pilot project to give stakeholders a taste of AI’s capabilities and build their trust gradually.
Ethical considerations
The long-term potential commercial applications of AI remain uncertain. It’s not just about what the technology is capable of – it’s primarily about meeting legal and ethical requirements.
A good example is our collaboration with Neuronest, where we were tasked with building an app for Alzheimer’s Patient Management. The project raised a lot of ethical and design questions, such as: “How do we deal with consent?”. “Do we inform the users that we use AI?”. “And if we do, how do we inform them?”
The dilemmas we’ve faced while building a healthcare app show just how careful we must be when using AI tools – especially if they become more autonomous over time.
What I recommend when you experience a similar situation is to put transparency forward. Clearly communicate to users when and how you’re going to use AI and explain the AI’s decisions.
Also, to secure your organization from a legal standpoint, remember to obtain users’ explicit consent before you collect and analyze their data.
Opportunities for AI in the design thinking process
AI enhances the discovery phase by analyzing large datasets to uncover deep user insights and trends. It synthesizes data in the 'Define' phase, pinpointing key issues for focused problem-solving. Integrating artificial intelligence ensures design efforts are both user-centered and data-informed. The ideation process driven by AI, generates a wide array of ideas based on data, suggesting innovative solutions and refining product designs. In prototyping, AI's low-code tools accelerate development, facilitating rapid iteration and enhanced insights for efficient product refinement.
The future of design thinking AI
AI's role in the design and innovation process is not about replacing humans but rather serving as a supportive co-pilot. By assisting with specific tasks while still under human oversight and direction, generative AI transforms the process into a powerful tool within a complex framework. This enhances efficiency in targeted areas. Such a collaborative approach ensures that designers remain fully engaged from start to finish, closely collaborating with developers throughout the project lifecycle.
As AI continues to advance, its influence on design thinking will expand. Future prospects include the ability to generate user interface concepts tailored to specific inputs and preferences, allowing designers to concentrate on strategic and creative endeavors.