Revolutionizing Retail with Generative AI: Personalized Recommendations in Ecommerce
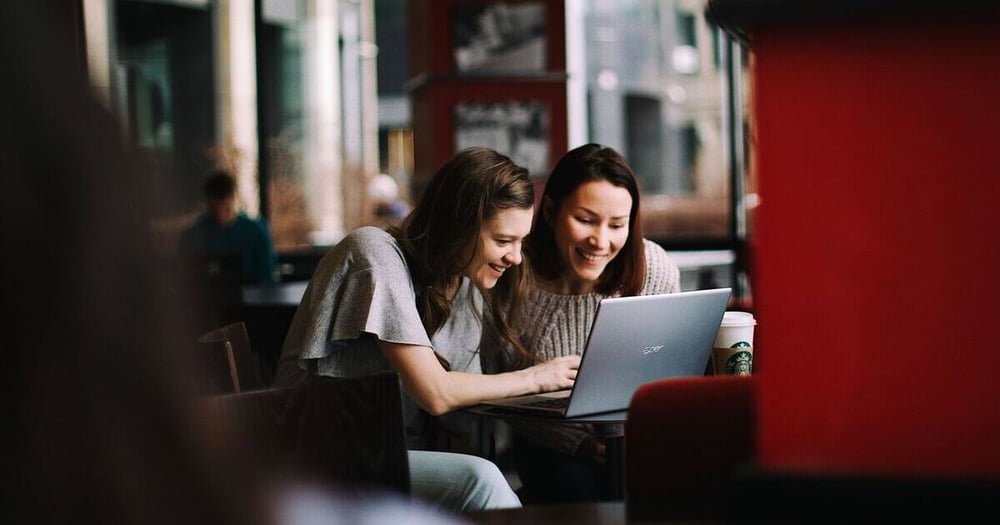
Imagine scrolling through an online store, only to be bombarded with recommendations for products you’ve already purchased? Unfortunately, this is a common issue that online consumers are facing. A recent Statista report, released in January 2023, revealed that 43 percent of survey respondents in the United States struggled with being marketed products they had already bought.
Companies must find innovative ways to stand out from the crowd to foster customer satisfaction and drive sales. One such strategy is through personalized product recommendations. By leveraging generative AI and ai product recommendations, businesses can create highly targeted suggestions based on customer preferences, purchase history, and browsing patterns. This approach not only improves customer satisfaction but can also significantly boost sales.
In this article, we’ll explore how generative AI works, what are its benefits for personalization and how it can revolutionize the customer experience.
Understanding User Behavior for Personalized Recommendations
Understanding user behavior is crucial for creating effective personalized recommendations. By analyzing user interactions, such as clicks, purchases, and search queries, businesses can gain valuable insights into their customers’ preferences and interests. This information can be used to create personalized recommendations that cater to individual users’ needs, increasing the likelihood of conversion and customer satisfaction.
There are several ways to analyze user behavior, including:
-
Clickstream analysis: This involves analyzing the sequence of pages a user visits on a website to understand their navigation patterns and interests. By tracking these interactions, businesses can identify which products or categories are most appealing to their customers.
-
Purchase history analysis: By examining a user’s purchase history, businesses can identify patterns and preferences that can inform personalized recommendations. For instance, if a customer frequently buys fitness equipment, the system can suggest related products like workout apparel or supplements.
-
Search query analysis: Analyzing search queries can provide insights into a user’s interests and preferences, allowing businesses to create targeted recommendations. For example, if a user frequently searches for vegan recipes, the system can recommend vegan cookbooks or ingredients.
By leveraging these insights, businesses can create personalized recommendations that are tailored to individual users’ needs, increasing the likelihood of conversion and customer satisfaction. This approach not only enhances the shopping experience but also fosters long-term customer loyalty.
The role of generative AI in personalized product recommendations
Generative AI refers to a set of advanced machine learning algorithms capable of generating new data points based on existing data. This technology enables the creation of highly personalized and relevant product recommendations by analyzing customer preferences, purchase history, and browsing patterns.
In the context of personalized product recommendations, generative AI can analyze vast amounts of customer data to identify patterns and trends, allowing businesses to create highly targeted marketing campaigns and product suggestions. AI recommendations leverage this data to enhance user experiences by predicting preferences based on past behaviors, aiding product discovery, and improving customer engagement.
Harnessing data for better customer insights
To fully harness the power of generative AI, businesses must first engage in data collection and analyze vast amounts of customer data. This data can come from various sources, including:
-
Purchase history: By analyzing past purchases, businesses can identify which products or services resonate with their customers and make relevant suggestions for future purchases. For example, if a customer frequently purchases baby clothes, generative AI can recommend baby accessories such as blankets or pacifiers.
-
Browsing patterns: Tracking how customers interact with a website or app can reveal their interests, preferences, and potential pain points, allowing businesses to create more targeted marketing messages and product recommendations. Suppose that a clothing ecommerce seller notices that many customers spend a lot of time browsing a leather jackets category or searching for specific leather jackets, this could reveal their interests and preferences. The retailer can then create more targeted marketing messages and promotions related to those products or categories, increasing the chances of a purchase and strengthening customer loyalty.
-
Customer preferences: Customers may voluntarily provide information about their likes and dislikes through surveys, reviews, or feedback forms. This data can be invaluable in crafting personalized product recommendations that meet their unique needs and preferences.
The power of machine learning algorithms
Once businesses have gathered and analyzed their customer data, they can leverage machine learning algorithms to identify patterns and generate personalized product recommendations. Some common types of machine learning algorithms used in this process include:
Collaborative filtering
This algorithm, known as memory-based collaborative filtering, analyzes the behavior of similar customers to make recommendations. For example, if Customer A and Customer B both purchased a specific product, the algorithm might suggest other products that Customer A has purchased to Customer B, and vice versa.
Content-based filtering
This approach focuses on the attributes of the products themselves, such as price, category, or brand, to make recommendations. If a customer has a history of purchasing products within a specific price range or from a particular brand, the algorithm might suggest similar products that align with those preferences.
Hybrid models
Collaborative filtering algorithms are integral to these models, which combine both collaborative and content-based filtering methods to create more accurate and comprehensive recommendations. By leveraging the strengths of both approaches, businesses can deliver highly personalized product suggestions that cater to a wide range of customer preferences and behaviors.
Leveraging Purchase History for Personalized Recommendations
Purchase history is a valuable source of data for creating personalized recommendations. By analyzing a user’s purchase history, businesses can identify patterns and preferences that can inform targeted recommendations. For example, if a user has purchased a product in the past, they may be interested in similar products or complementary items.
There are several ways to leverage purchase history for personalized recommendations, including:
-
Collaborative filtering: This involves analyzing the purchase history of similar users to identify patterns and preferences that can inform recommendations. For instance, if two customers have purchased the same book, the system might suggest other books that one customer bought to the other.
-
Content-based filtering: This approach focuses on the attributes of products that a user has purchased in the past to identify patterns and preferences. For example, if a customer frequently buys products from a specific brand, the system can recommend other items from that brand.
-
Hybrid approach: Combining collaborative filtering and content-based filtering creates a more comprehensive understanding of a user’s preferences and interests. This method leverages the strengths of both approaches to deliver highly personalized recommendations.
By leveraging purchase history, businesses can create personalized recommendations that are tailored to individual users’ needs, increasing the likelihood of conversion and customer satisfaction. This strategy not only boosts sales but also enhances the overall shopping experience.
Real-world examples of generative AI in action
-
Amazon’s personalized recommendations. Amazon utilizes generative AI algorithms to analyze vast amounts of customer data, delivering highly personalized product recommendations that have contributed to their continued success. A personalized recommendation system, like the one used by Netflix, significantly boosts viewer engagement and revenue growth. According to Forbes, in 2021 35 percent of what consumers purchase on Amazon was a result of product recommendations.
-
Spotify’s music suggestions. Spotify employs generative AI to analyze user listening patterns and preferences, generating curated playlists and personalized music recommendations that keep users engaged with the platform.
-
ASOS’s personalized styling service, which leverages generative AI to create a virtual catwalk that provides customers with personalized styling offers. The AI analysis a customer’s body shape and suggests outfits accordingly, generating a new outfit combination each time the user refreshes the page.
-
Wayfair’s recommendations based on uploaded images create personalized product suggestions for clients by analyzing the images of their rooms and their preferences, which leads to more individualized and pertinent recommendations.
Benefits of personalized product recommendations
Implementing personalization for product recommendations powered by generative AI can significantly enhance user engagement and provide numerous advantages for businesses, including:
Improved customer satisfaction
Customers appreciate when a company understands their needs and preferences. By providing personalized product recommendations, businesses can create a more enjoyable shopping experience, leading to higher customer satisfaction levels. This, in turn, can foster long-term loyalty.
Increased sales and revenue
Personalized product recommendations have a higher likelihood of conversion compared to generic suggestions. By targeting customers with relevant products that align with their preferences and purchase history, businesses can significantly increase the probability of a sale. This results in higher average order values, boosting overall revenue.
A research from Monetate reveals that product recommendations can lead to a 70% increase in purchase rates, both in the initial session and in return sessions, and 33% higher average order values.
Enhanced brand perception
By utilizing generative AI to deliver personalized product recommendations, businesses can position themselves as forward-thinking and innovative. This enhanced brand perception can set a company apart from its competitors, attracting new customers and retaining existing ones. In an increasingly crowded market, staying ahead of the curve and embracing cutting-edge technology can be a crucial differentiator.
Streamlined inventory management
By better understanding customer preferences, businesses can make more informed decisions about their inventory. This can help companies optimize stock levels, reduce waste, and improve their overall supply chain efficiency.
Efficient marketing efforts
Personalized product recommendations enable businesses to create highly targeted marketing campaigns that resonate with their audience. By using generative AI to analyze customer data, marketers can craft messages that are more likely to engage and convert, ultimately increasing the return on investment (ROI) of their marketing efforts.
Challenges and limitations of generative AI for personalized recommendations
Data quality and quantity
Generative AI algorithms require large quantities of high-quality data to generate accurate and relevant recommendations, which can be challenging to obtain and maintain. Unlike personalized recommendations that leverage data and machine learning algorithms, business rules can lead to static recommendations based on factors like low stock or best sellers.
Algorithm complexity and computational requirements
The complexity of generative AI algorithms and their computational requirements can pose challenges in terms of processing power and infrastructure.
Maintaining relevance over time
As customer preferences and market trends change over time, businesses need to ensure their generative AI algorithms remain relevant and up-to-date.
Data privacy and security
As businesses collect and analyze vast amounts of customer data, it's essential to prioritize data privacy and security. Companies must ensure that they adhere to relevant data protection regulations, and implement robust security measures to safeguard their customers' information.
Ethical AI practices
With the rise of AI technology comes the responsibility to ensure that it's used ethically. Businesses should be transparent about their use of AI and ensure that their algorithms are unbiased and fair. This may involve regular audits of the AI system to identify and rectify any potential issues or biases.
Measuring Success and Optimizing Recommendations
Measuring the success of personalized recommendations is crucial for optimizing their effectiveness. There are several metrics that can be used to gauge the success of personalized recommendations, including:
-
Conversion rate: This measures the percentage of users who make a purchase after receiving a personalized recommendation. A higher conversion rate indicates that the recommendations are effectively driving sales.
-
Click-through rate: This measures the percentage of users who click on a personalized recommendation. A high click-through rate suggests that the recommendations are relevant and engaging to users.
-
Average order value: This measures the average value of orders placed by users who receive personalized recommendations. An increase in average order value indicates that the recommendations are encouraging users to purchase more items.
By analyzing these metrics, businesses can identify areas for improvement and optimize their personalized recommendations to increase their effectiveness. This can involve refining the algorithms used to generate recommendations, adjusting the frequency and timing of recommendations, and testing different types of recommendations.
Additionally, businesses can use A/B testing to compare the effectiveness of different personalized recommendation strategies and identify the most effective approach. By continuously measuring and optimizing personalized recommendations, businesses can increase their effectiveness and drive revenue growth. This ongoing process ensures that the recommendations remain relevant and valuable to customers, ultimately enhancing the overall shopping experience.
Is it possible to implement generative AI for small businesses?
Small businesses may face challenges when implementing generative AI, such as limited resources, budget constraints, and the need for technical expertise.
Despite these challenges, there are affordable solutions and resources available for small businesses, such as pre-built AI recommendation engines, open-source software, and AI-as-a-service platforms.
Embracing generative AI for personalized product recommendations
In today's competitive business environment, offering personalized product recommendations powered by generative AI can provide a significant advantage. By leveraging customer preferences, purchase history, and browsing patterns, businesses can create highly targeted marketing campaigns and product suggestions that improve customer satisfaction, boost sales, and enhance their overall brand perception.
By prioritizing data privacy and security, adhering to ethical AI practices, and committing to ongoing optimization, businesses can successfully harness the power of generative AI to revolutionize the customer experience and drive long-term success.