Hand Detection using ObjectDetection API on Android
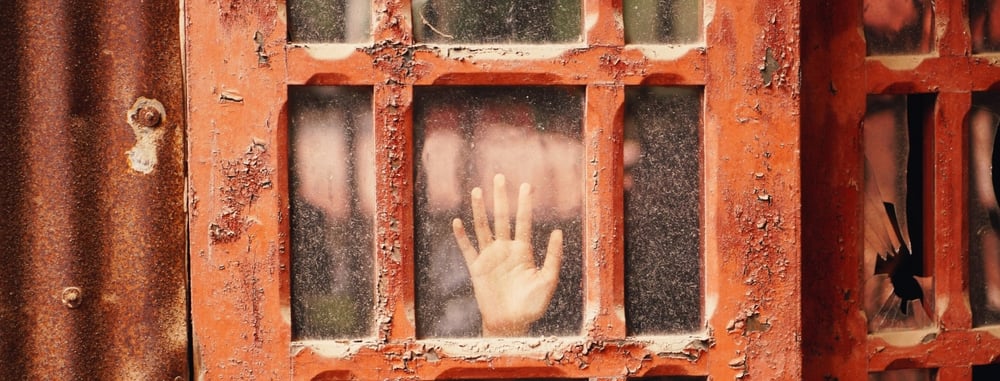
For some time now I’ve been interested in machine learning and I thought of implementing this myself. To solve this problem I’ve used Object Detection API SSD MultiBox model using
- The first step is to install all the necessary dependencies and clone the Object Detection API repository. The
ObjectDetection API repository can be cloned from https://github.com/tensorflow/models. - Before we can start training the model we need some input data for training and evaluation, in a format accepted by the
ObjectDetection API -TFRecord .Additionally we should specify the label map, which does the mapping of class id toclass name. Labels should be identical for training and evaluation datasets. To accomplish this step I’ve used this script, that fetches human hand pictures dataset from http://www.robots.ox.ac.uk/~vgg/data/hands/index.html and creates necessary files.Output of this script: hands_train.record, hands_val.record, hands_test.record and hands_label_map.pbtxt. - When the input files are ready we can start configuring our model.
ObjectDetection API usesprotobuf files to configure train and eval work, more info about configuration pipeline can be found here.ObjectDetection API provides several sample configuration. Those configurations are a good starting point, with minimal effort you’ve gotworking configuration. As I wroteon the beginning of this post I’ve used ssd_mobilenet_v1_coco.config. I’ve changed following parameters: - num_classes to 1 because I wanted to detect only one type of objects - hand.
- num_steps to 15000 because running locally can take forever :D
- fine_tune_checkpoint to location of earlier downloaded frozen model ssd_mobilenet_v1_coco_2017_11_17/model.ckpt.
- input_path and label_map_path of train_put_reader and eval_input_reader path to previously generated hands_train.record, hands_test.record and hands_label_map.pbtxt files.
- I’ve trained
model on my local machine, to do this I’ve usedscript fromlibrary :
python object_detection/train.py \
--logtostderr \
--pipeline_config_path=object_detection/ssd_mobilenet_v1_hands.config \
--train_dir=object_detection/training/ - The library also provides a script to evaluate the model during and after training:
python object_detection/eval.py \
--logtostderr \
--train_dir=object_detection/training/ \
--pipeline_config_path=object_detection/ssd_mobilenet_v1_hands.config \
--checkpoint_dir=object_detection/training/ \
--eval_dir=object_detection/training/ - After the work is done we can freeze our trained model using the following script:
python object_detection/export_inference_graph.py \
--input_type image_tensor \
--pipeline_config_path object_detection/ssd_mobilenet_v1_hands.config \ --trained_checkpoint_prefix object_detection/training/model.ckpt-15000 --output_directory object_detection/frozen/ - Now it’s time to use our model to detect a hand on a mobile device’s camera preview. To implement this quickly I’ve used a demo project from the TensorFlow repo. I’ve cloned it and imported a project from examples/android directory.
Project can be build using different build systems:bazel ,cmake , makefile, none. I’ve builtproject usingcmake , to do this I’ve changed inbuild .gradle variable nativeBuildSystem tocmake (I hada problems with others build systems). - In order to use the frozen model and labels, we need to put them in the assets directory, then assign the assets names to the TF_OD_API_MODEL_FILE and TF_OD_API_LABELS_FILE variables in DetectorActivity class.
Additionally I’ve changed the camera preview to use the front camera and implemented a toast message to pop up when a hand is detected. - Results of my experiment:
Thanks to the