How Hyperspectral Imaging Contributes to Sustainable Agriculture?
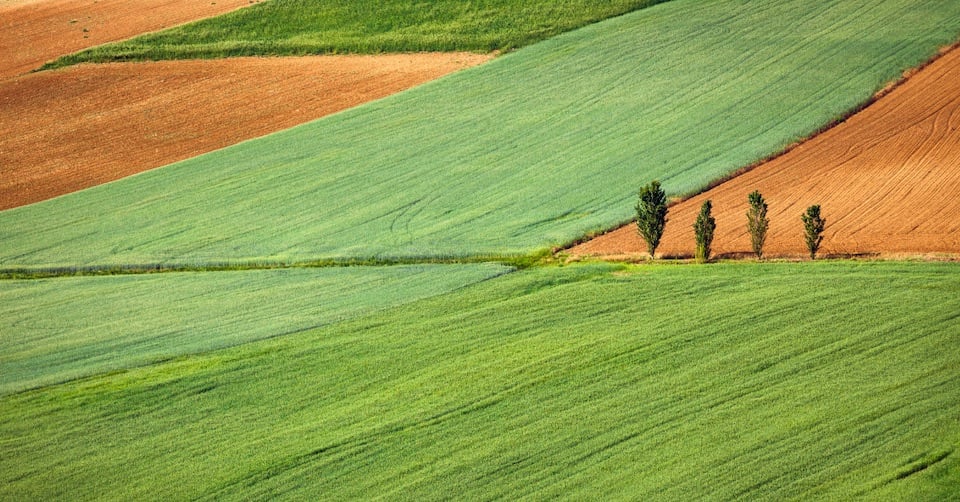
The conventional approach, however, has been to view agricultural yields and environmental protection as some sort of a zero-sum game in which the latter was constantly out-prioritised by the former.
As a result, we have only managed to exacerbate the already adverse environmental impact of agriculture in terms of groundwater depletion, water pollution from fertilizer runoffs, biodiversity loss, soil erosion, and high rates of greenhouse gas emissions.
We now face the crying need to increase food production by 70 percent to ensure the food security for a global population of over 9 billion by 2050. Reverting to conventional farming techniques to achieve that objective will only result in an increase in the environmental effects of food production, cause yields to decline, and prematurely derail our Food Security by 2050 campaign.
This is the Gordian knot that sustainable agriculture has to untie. And sustainable farming principles powered by digital-era technologies such as hyperspectral imaging and advanced machine learning are ushering in a new era of precision agriculture.
What Is Precision Agriculture?
Precision agriculture harnesses the power of sophisticated digital technologies to empower farmers to increase their yields while reducing risks, volatility, and environmental impact.
Data is the key driver of precision agriculture. Precision agriculture prioritises the mapping and analysis of data from every input, process and activity in farming operations. This technology-led, data-driven, and insight-based approach enables accurate real-time mapping of all elements of food production, including soil testing, field mapping, crop scouting, irrigation management, yield monitoring, and weather tracking and forecasting.
Today, precision agriculture relies on a range of advanced technologies including sensors, drones, robotics, guidance systems, remote sensing, and variable rate technology. Hyperspectral imaging and advanced machine learning are two key components that drive strategic decision making in sustainable agriculture.
Data Mapping Using Hyperspectral Imaging
Spectroscopy, the study of how different materials interact with light, is a popular technique used to detect, identify, and analyse different materials based on their unique spectral fingerprint. Spectral imaging combines spectroscopic and spatial data to create a chemical map of the imaged area.
There are several types of spectral imaging, including multispectral and hyperspectral imaging (HSI), distinguished primarily by their spectral resolution. A standard camera, for instance, is designed to detect light from the visible spectrum across three parts of visible spectrum (red, blue, and green), which we call colors. In comparison, multispectral imaging can capture light from even beyond the visible spectrum, encompassing three to ten spectral bands.
HSI covers a broad electromagnetic spectrum, like multispectral imaging, but can operate across tens or hundreds of much narrower bands. This results in images with a much higher spectral resolution, containing more detailed data about the target (Fig. 1). This data is available as a three-dimensional cube (Fig. 2) – comprising spectral data along one dimension and spatial data across the other two – that can be used to create a detailed pixel-by-pixel chemical and spatial map of the target.
Fig. 1: Multispectral vs hyperspectral imaging (source: gamaya.com)
Fig. 2: Data cube with spectral/spatial dimensions. (source:researchgate.net)
There is a huge range of applications for HSI in agriculture, including vegetation mapping, yield estimation, disease monitoring, pest and pollutant detection, etc.
There are, however, some serious data challenges when it comes to the analysis of hyperspectral imagery. Notwithstanding the wealth of spectral information embedded in these images, the high dimensionality created by the thousands of pixels can make classification and analysis a challenging task. In the next section, we will see how advanced machine learning models are rising to this challenge and helping unlock the full potential of HSI.
Data Analysis Using Deep Learning
Inherent HSI data characteristics, such as high dimensionality, non-linear correlations, and large spatial variability of spectral signatures, can make classification and analysis a challenge even for traditional machine learning methods. But recent successes with deep learning in areas like image recognition, object detection and computer vision suggest a huge potential for applying these models to the analysis of hyperspectral imaging.
Deep learning solutions, especially convolutional neural networks (CNNs), have proven to be successful in the classification of hyperspectral images. The deep convolutional layers used in these models enable them to extract high-level spatial-spectral features effectively, and deep learning models are better at extracting even more abstract features.
Although deep models and CNNs fare better than conventional machine learning techniques, they face a new set of challenges. Primary among these is the requirement for a large number of training samples, which can be expensive and time consuming.
But new frameworks are constantly being explored to mitigate these challenges. For instance, one approach proposes a new deep learning framework that integrates gray level co-occurrence matrix (GLCM) features into CNNs to account for the limited number of samples. Experiments with deep&dense CNN models also seem to show a lot of promise in addressing some of the current limitations and enhancing the classification accuracy for hyperspectral imaging.
The Way Forward
Until recently, hyperspectral cameras were too bulky and expensive to facilitate widespread deployment. But the increasing availability of compact, low-cost equipment is rapidly expanding the global HSI systems market. In parallel, the development of unmanned aerial vehicles (UAVs), including mini-UAV-borne hyperspectral remote sensing systems is accelerating the adoption of spectral imaging in agriculture and in other civilian applications.
AgTech startups like Gamaya and FluroSat are already offering commercial solutions that combine airborne remote sensing with HSI and artificial intelligence to improve the efficiency and sustainability of agriculture. As early as 2016, researchers in Finland were able to convert an iPhone into the world’s first hyperspectral mobile device. Developments like these are constantly making these technologies more affordable and accessible for mainstream agricultural applications.
The combination of hyperspectral imaging and deep learning has the potential to deliver a host of actionable insights that can help farmers increase the productivity, profitability, and sustainability of their agricultural operations. Accelerating and expanding the adoption of these technologies to agricultural communities across the world is imperative if we are to be able to truly realise the value of sustainable agriculture in time for our 2050 deadline.