Business Intelligence Culture: Data-Driven Decisions in the World of Insurtech
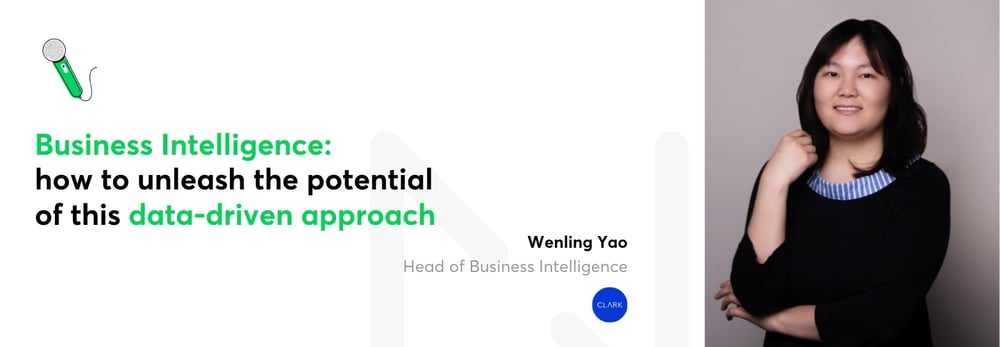
What's more, a few organizations from this sector have mastered the art of continuously validating and improving all business-related ideas. All their decisions are based on a solid analytical approach instead of trusting guesswork.
We met with Wenling Yao, Head of Business Intelligence at Clark, a company operating in the insurtech sector, to discuss Clark's BI culture, unveil the full potential of this data-driven approach, and get to know the expert's opinion on the trends in BI.
Paulina Golab, Netguru: Please, share a few words about Clark
Wenling Yao, Clark: Clark is a digital insurance manager. With our app, we aim to provide a digital platform for our customers to manage their insurance contracts. We act as an independent insurance broker to give our customers advice and help them handle their insurance digitally.
Our target audience comprises young professionals who are very keen on using digital products for many aspects of their lives, like urban transportation, scheduling doctor's appointments, financial planning, and so on.
At Clark, we believe that insurance should be the next area to be digitalized and made much easier for them to access and leverage. That is why Clark exists as a company and that's our mission.
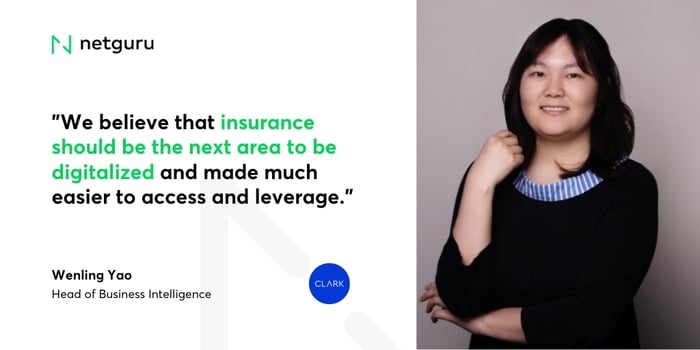
So Clark moves all interactions that used to happen face-to-face online. What are your ideas to automate areas like life and equity insurance, as it's proven that human to human contact is more efficient there?
Because the nature of these products is pretty complex, automating the sales process is not an easy thing to do and full automation will never be our goal for these specific products. A big part of it is still human-driven, because customers value expert support in this area. This is why our customers can interact with our insurance consultants via video chat, messenger or telephone in addition to our in-app solution.
At the core of what we have achieved so far, we have made the recommendation stage fully automated. Our customers only need to answer a few simple questions: their family status, their age, their income, their profession, their ownership of cars and properties. Based on that information, our system can instantly provide an automatic assessment of a client's current life and insurance situation and recommend them the most suitable life insurance plan.
The second automation we have done in this area is that we have a so-called retirement check where we only need customers to provide us with some information about their current income and pension insurance details. This data enables us to identify the income gap they may have once they retire and offer optimized recommendations on how they could fill out the gap.
Actually, it is a Q3 OKR for the Business Intelligence department to plan a data-supported sales process.
However, for now, automation does not encompass the whole process?
It doesn’t, because there will always be products that customers will want personal consultancy on, which we gladly offer them. But we do aim to automate matchmaking between customers and consultants. The assignment of consultants to customers is mainly based on some simple logic like capacity and expertise. But we want to develop logic that can improve the efficiency of this matchmaking using historical data.
One solution is to identify the best dynamic touchpoints with interested customers. Suppose we spot that some customers have viewed the recommendation details of a specific insurance offer more than five times in a day but have not taken any further action. In that case, we can send them a friendly reminder and assess their reaction. Thanks to that, we can analyze user behavior, identify the best touchpoint, and adjust our further interactions with certain groups of customers.
Do you notice any particular trends in the insurtech sector related to digitization and the role of technology?
One aspect that I found very interesting when I was researching the markets is the possibility to collect health data by wearable devices that can be later used for automation in the life insurance area.
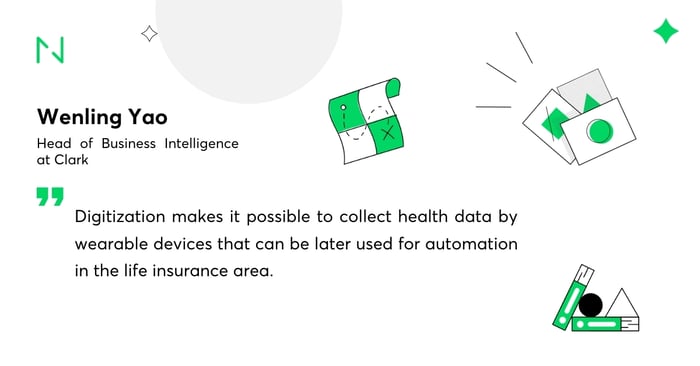
Customers can provide insurers with data from their smartwatches. This information, leveraged by automation, will help insurance companies to assess any risks more accurately than they are able to do now. Based on that unique data collection, they can offer fully customized policies for individual clients and, for example, offer them discounts, because they exercise regularly and lead a healthy lifestyle. It's all about analyzing the right data.
Speaking of data. What does Business Intelligence mean to you? Why do you think companies should implement this kind of approach?
To me, BI is the bridge between business and technology, the one that speaks both languages and helps both worlds to understand each other. A BI culture is a fact-based culture, where ideas are continuously validated and improved.
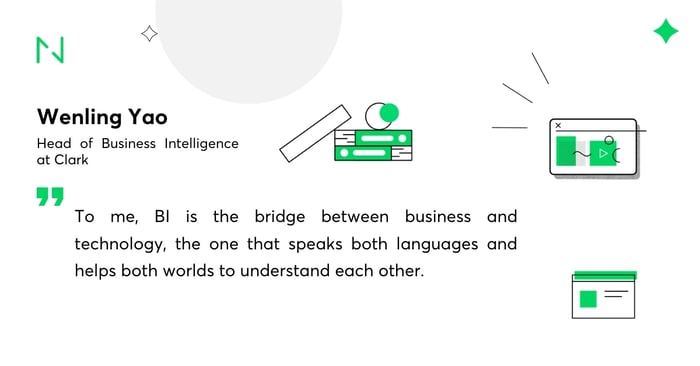
We, humans, inherit a Business Intelligence approach. In our early days, we started to collect tools, we started herding animals, and we already started to count at that time. We counted how many animals we have today and how we should distribute resources throughout our tribe. And I believe we started to realize the importance of measuring our performance at that point.
So the basic idea behind Business Intelligence is not something new. However, we leverage it in a new way.
What should motivate a company to invest in technology to implement a Business Intelligence department is the possibility to enable every employee to be able to read data, make data-driven decisions and understand what results their work brings.
Business Intelligence also gives companies the ability to improve their performance by looking for patterns in their business history and learning from it. This kind of approach is vitally important in this big data era.
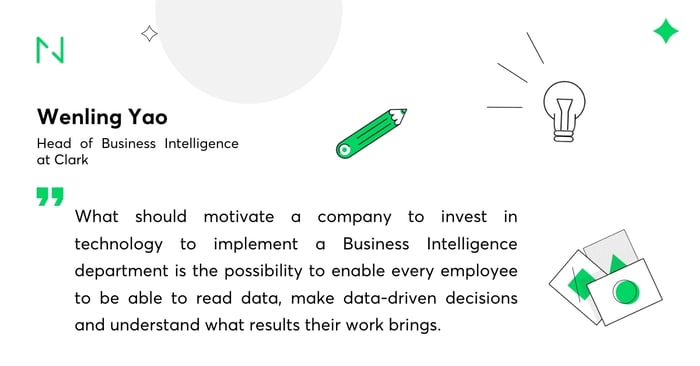
Is implementing a Business Intelligence approach a huge challenge for a company?
In the ideal world, everyone should leverage data while making business decisions. So it is a big challenge for an organization to implement such an approach company-wide.
I can only speak about my experience at Clark, though. Fortunately, this data-driven DNA was rooted within the company from the very beginning when I joined Clark four years ago. I started in the technology team, where I helped to build the reporting infrastructure from scratch: setting up tools, initial dashboards, and initial metrics that we should look at.
As the business grew, the BI function moved from the technology team more to the executive team. I started looking at a higher, strategic level, and leverage Business Intelligence there.
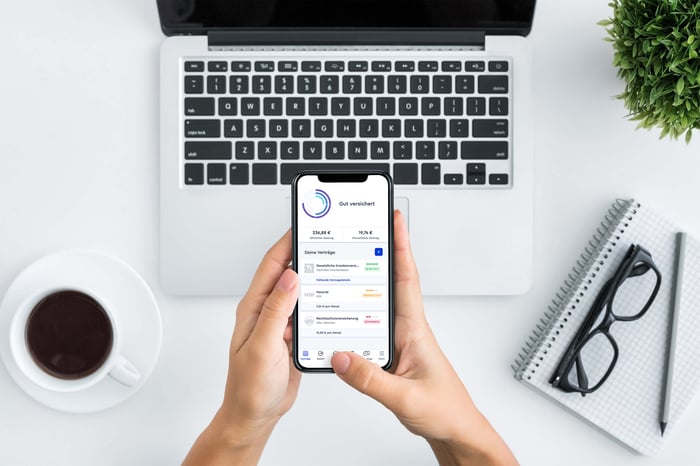
How has BI changed Clark and the decision-making processes within the company? Could you share some examples of BI-driven decisions?
Based on the logic tree of business KPIs, BI provides a mini business case builder for all stakeholders to play around with their assumptions and envision the impact on key business results.
Let's say, a marketing department wants to plan the next advertising campaign. They can leverage BI tools and analysis to decide how to shape their campaign, what audience, or industry they should focus on. And their decisions will be informed, based on data and facts, not assumptions or feelings about a certain initiative.
Your goal as the Head of BI is also to make sure that all employees understand the importance of data-driven decisions. How do you achieve that?
So we have some initiatives already in place, and then one initiative is still on the way. We make sure every new joiner at Clark goes through a one-week onboarding journey, where they meet department leads, and one of the department leads of BI is also there. Their role is to introduce us and describe the way we work.
We present a logic tree and aim to make new joiners aware of how their team is contributing to the company's success and what results from their performance they can expect to see every month. We also have a kind of onboarding test journey at the end with several questions to test if new joiners understand some basic facts about the Business Intelligence approach.
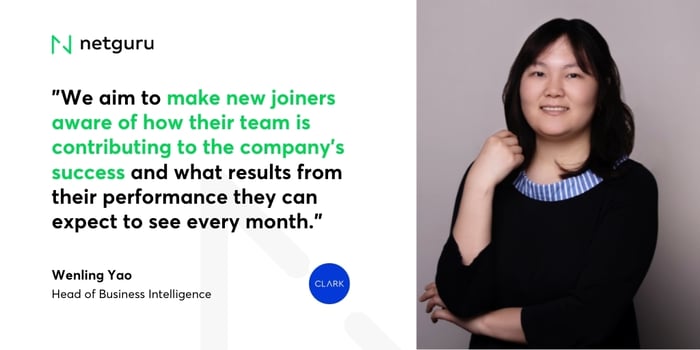
The goal is to make every employee know data such as: how many customers Clark has, what is their average age, what is the average income from certain products, etc.
We believe it helps employees to understand the bigger picture, make better-informed decisions, and provides more context to make them realize that those basic facts are essential.
There's also a dictionary of the most commonly used terms related to Business Intelligence to keep everyone on the same page.
Marketing and sales teams can have different definitions of, let's say, a potential customer, right? The marketing team could say, "Anyone who sees our advertisements is a potential customer". While salespeople may think that anyone they talk with on the phone is a potential buyer.
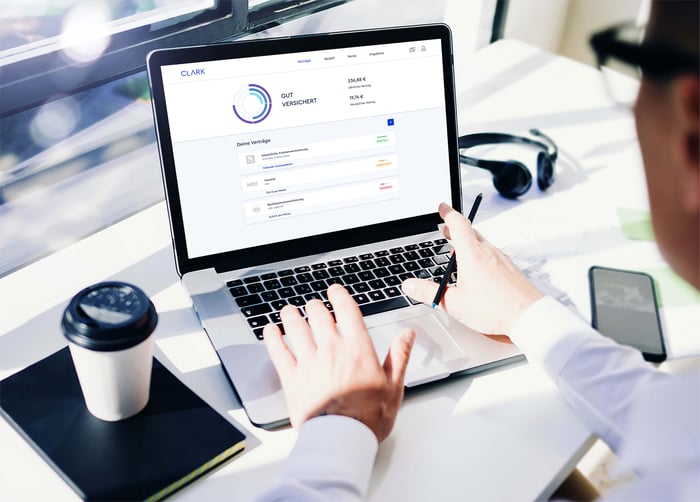
But the truth is somewhere in between. By having this dictionary in place, we want to make sure that we mean exactly the same thing when we use the term "potential customer". And we don't have to waste a lot of time on clarifying and explaining our understanding to each other. It reduces communication costs.
And there's the 3rd initiative. At Clark, we use a general BI tool called Superset. It's an online data visualization platform connected to our database to provide automated reporting. And we have regular training for the whole company and also on request for each department to enable users to build simple data visualizations and generate reports on their own.
How do you encourage your employees to follow this approach? What's their motivation to rely on data?
For each product feature, we require a success metric to be defined and a target to be set. Target setting relies on historical data whenever possible, we implement everything in a way that enables us to report on a KPI that is incorporated in the feature. The NPS widget is a good example.
The process of motivating and educating employees is ongoing. Project Managers are already fully aware that whenever they have a new feature chosen for ideation, they always need to define the success metric to measure this feature's impact on the business. This is like a must component of each product development discussion. The target line must also be set.
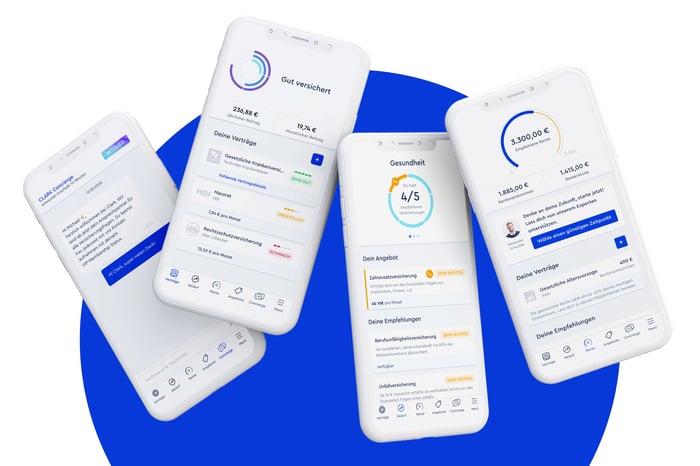
Business Intelligence also plays a role in helping them or suggesting the selection of KPI and in setting up target lines if they need help.
The second thing is that sometimes the success metric is not measurable based on the data we already have in place. In such a case, we have to make sure that the tracking implementation needed to measure this metric is also in place, and we will be able to gather missing data. Otherwise, the feature will not be put into the implementation phase.
And this where BI jumps in to offer some consultation. We make data more accessible, and we want to center all our decisions around it.
How do you assess the degree of maturity of Business Intelligence in the world? Do companies widely use this approach?
I would say that there is a lot of potential. The degree of maturity of BI among startups is generally much higher compared to traditional industries like fast-moving consumer goods, for instance.
Because such companies have a long history and have a lot of legacy in place, their systems could be circumvented -- they may have a lot of data, but spread across different systems. This makes it hard to consolidate change and connect the dots.
So I think that would be the challenge that most companies in the traditional industry are facing. They need to organize all the data they have first to start making fully data-driven decisions. And obviously, those blocks need to be broken down to unlock the full potential of implementing a Business Intelligence approach.