6 Ways Machine Learning is Changing Insurance [Updated]
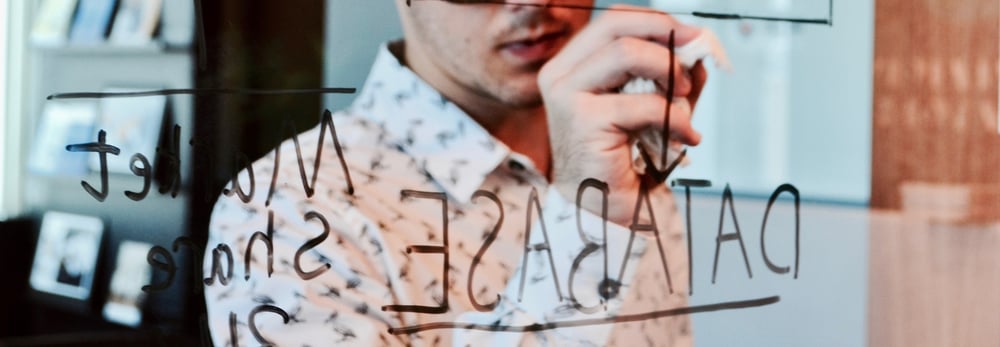
Insurers are using machine learning to increase their operational efficiency, boost customer service, and even detect fraud. And there is a plethora of insurtech startups, eager to take a slice of an insurance industry.
Here are 6 ways machine learning is transforming the insurance industry.
----------------------------------------------------------------------------------------
Note: this post has been updated, to reflect the progress of new technologies and business moves by companies mentioned in the article.
----------------------------------------------------------------------------------------
1. Automation and process improvement
The insurance industry is regulated by specific legal requirements. It processes thousands of claims and responds to even more customer queries.
Machine learning can improve this process and automatically move claims through the system – from the initial report to analysis and contact with the customer.
In some cases, these claims may not require the work of human employees at all, allowing them to dedicate more time to more demanding claims.
Insurance companies are already automating some parts of their claims process, benefiting from significant time savings and increased quality of service.
.jpg?width=700&name=Untitled%20design%20(1).jpg)
For example, Captricity has developed algorithms able to extract handwritten or typed forms into a digital form with a smashing 99.9% accuracy, helping insurers to reduce cycle times.
On February 2019 Captricity announced Captricity READ, the first AI-powered software that, according to company's claims, outperforms humans at reading handwriting.
That is a watershed moment for digital automation. Enterprises have struggled with automation technology due to extremely low accuracy in reading handwriting and poor-quality images. (...) Captricity READ is the missing link - said Kuang Chen, founder and CEO of Captricity.
Lemonade is an insurance company that uses AI to process claims more quickly and provide customers with fast payouts using various applications, such as a chatbot.
That is one of the fastest growing company in insurance space - Lemonade raised $780 million already, and is using the funding to expand into Europe (with the Germany as their first market).
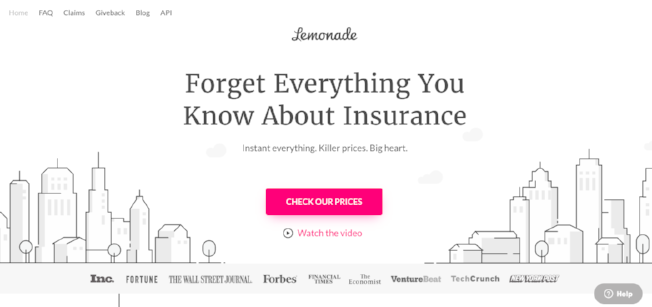
“The value proposition we’ve created resonates with young consumers in a universal way,” said Daniel Schreiber, founder and CEO of Lemonade, as quoted on TechCrunch.
Another example is the technology provided by Tractable, a startup that offers software for assessing damage and predicting repair costs to accelerate claim processing.
On April 2019, Tractable hosted webinar to showcase their technology - the company invited participants from all over the world to submit photos and evaluate the results of its visual algorithms.
2. Data insurance - more sophisticated rating algorithms
Rating serves as the foundation of insurance companies. There’s a famous saying in the insurance world: “There are no bad risks, only bad pricing.” That means companies are able to accommodate most risks as long as they find a good match in pricing.
However, many insurers still rely on traditional methods when evaluating risk. For example, when calculating property risks, they may use historical data for a specific zip code. Individual customers are often assessed using outdated indicators, such as credit score and loss history.
In this context, machine learning can offer agents new tools and methods supporting them in classifying risks and calculating more accurate predictive pricing models that eventually reduce loss ratios.
An example of this is vehicle telematics – the combination of vehicles, computers, and wireless telecommunication technologies that facilitate the flow of information over vast networks. Here’s a case study of the Italian market which has the highest coverage of telematics-based motor policies in the world.
Another example is Zendrive, a mobile app that monitors the driving behavior of customers to potentially offer them significant discounts on car insurance premium.
Based on 60bn journeys's data from 2018, Zendrive estimate that rate of smartphone use behind the wheel may be as high as 60% (source: The Economist).
- The current models of auto insurance - using proxy variables for responsibility like age, education, marital status, homeownership, etc. - have little direct impact on save lives on our roads - Noah Budnick, Data Practice & Policy Director at Zendrive told us.
- Zendrive wants to help communities achieve Vision Zero - the elimination of traffic deaths and injuries - and our technology can help identify the driver behaviors that are most likely to contribute to serious crashes, injuries and deaths. With this data, insurers can create policies to save lives on the roads - he added.
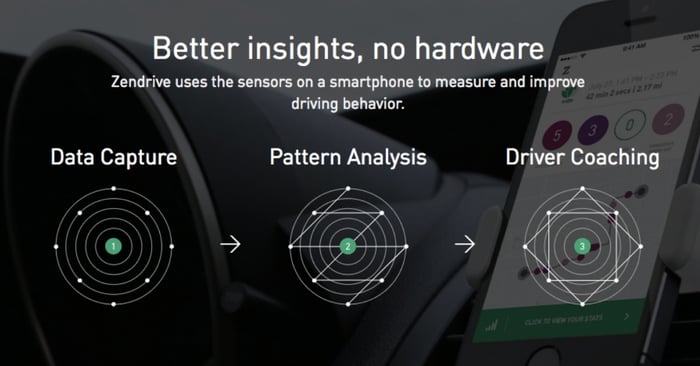
3. Improving underwriting
One area where machine learning can bring benefits in the process of underwriting is healthcare. Healthcare insurance provides coverage of costs incurred by disease, accident, disability, or death. This area of insurance stands to benefit a lot from data-driven approaches as the healthcare analytics market is on the rise.
Insurance companies need to provide better services and reduce their costs. They can now use machine learning-powered tools that help to consolidate insights from massive volumes of highly varied data such as insurance claims data, membership and provider data, benefits and medical records, and many others. These solutions can structure and process data to offer healthcare insurance businesses insights leading to costs reduction, higher quality of care, and fraud detection.
An example of such technology is Daisy Intelligence, which provides price suggestions for different customers based on their individual risk factors, such as age, location or even blood pressure.
On April 2019, the company has beaten 12 other startups to won the Canadian Fintech 3.0 Summit’s Future of Retail pitch battle.
- We’re establishing a new category, A.I. empowered category management – and delivering disruptive financial benefits to retailers and insurance companies - said Gary Saarenvirta, Daisy’s founder and CEO.
Another area that stands to benefit from machine learning technologies is property insurance.
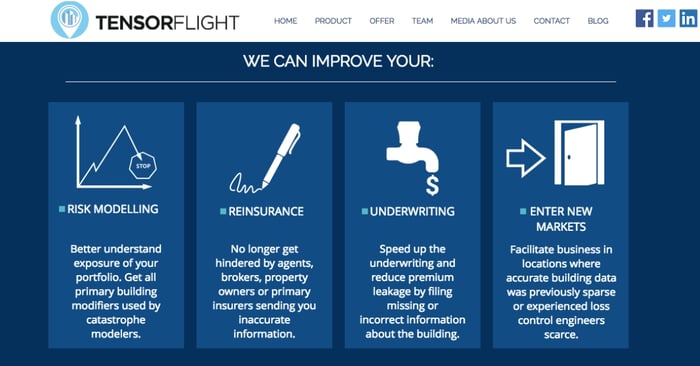
Projects such as Tensorflight provide satellite and street image processing tools to improve the underwriting process and eliminate misclassification errors that are expected to cause the US sector to lose $4.5 billion over the next 4 years.
4. Better customer lifetime value (CLV) prediction
Customer lifetime value (CLV) is a complex metric that represents the value of a customer to a company as the difference between the revenue gained and expenses incurred – all projected onto the entire relationship with a customer, including the future.
Insurance companies predict CLV with the help of customer behavior data that allows them to assess the customer's potential profitability for the insurer and create a more personalized marketing offer.
Such behavior-based machine learning models can be applied to forecast retention or cross-buying, all critical factors in the company's future income.
Machine learning tools also help insurers to predict the likelihood of a particular customer behavior – for example, their maintenance of the policies or surrender.
5. Personalization in marketing
Customers expect to receive personalized services that match their needs, preferences, and lifestyles. Creating personalized insurance experiences with the help of advanced analytics and machine learning is now an option companies can use to improve their marketing ROI.
They can draw insights from data about individual preferences, behaviors, attitudes, lifestyle details, and hobbies to create personalized products such as policies, loyalty programs, and recommendations. All of that is possible thanks to machine learning algorithms applied to data sets to develop suggestions that fit specific customers through sophisticated selection and matching mechanisms.
By taking advantage of machine learning solutions, insurers can offer customers personalized services, machine-generated insurance advice or even employ chatbots like ABIE to help insurers make the right policy choice. Consumers have nothing against that – in fact, 74% of them are happy to interact with machines in that context.
6. Fraud detection and prevention
Fraud is a serious concern that costs the US insurance sector over $40 billion a year. If insurance companies found methods to mitigate fraud effectively, they could positively impact their profit and loss statements. And that's where machine learning algorithms can help.
They are already being used by the industry leaders to identify claims that are more likely to be fraudulent than others and subject them to further investigation by human employees.
Machine learning tools enable insurance companies to take action against fraud much more quickly than when relying on human analytical capabilities alone.
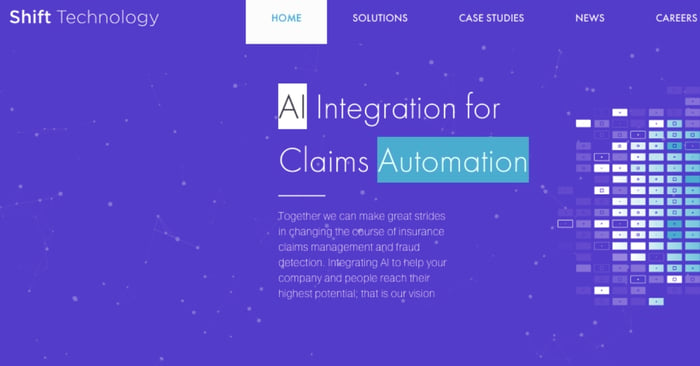
For example, the Paris-based startup Shift Technology has developed an AI-powered solution to better spot potential fraud among insurance claims and provide fraud handlers with clear and actionable rationale for why the claim was scored with a high potential for fraud and best next steps for investigating the claim.
The solution supposedly boasts a 75% accuracy rate, double the market standard. The accuracy rate refers to claims identified as potentially fraudulent that the handler elects to move forward.
- As for key milestones in 2019, we’re really excited about the trajectory the company is on. Since our launch in 2014, we’ve raised more than $40M, expanded our global footprint – most recently opening new offices in Boston and Tokyo – and we’ve signed contracts with more than 60 insurers throughout Europe, Asia, and the Americas - Rob Morton from Shift Technology told us.
- Most recently, we announced a strategic partnership in the US with the National Insurance Crime Bureau and signed on HyreCar, an innovator in both the ridesharing and insurance industries as a Shift customer - he added.
AI and Insurance - Key Takeaways
Machine learning is on its way to causing a massive disruption across many different industries, and insurance is no exception. Q1 of 2018 saw $728 million invested into insurtech companies across 66 transactions.
Since insurance companies have always worked with data, it only makes sense that they ride the digital transformation wave and implement machine learning solutions that give them a more in-depth look into this data to uncover new insights. And these come in handy for a broad range of purposes, from fraud detection to development of rating algorithms that determine the best pricing strategies.
Machine learning is on the rise, so we're bound to see these applications mature and new ones appear on the insurance scene to accelerate the sector's digital transformation.