How Technology is Transforming On-Shelf Availability for FMCG and Grocery Retailers
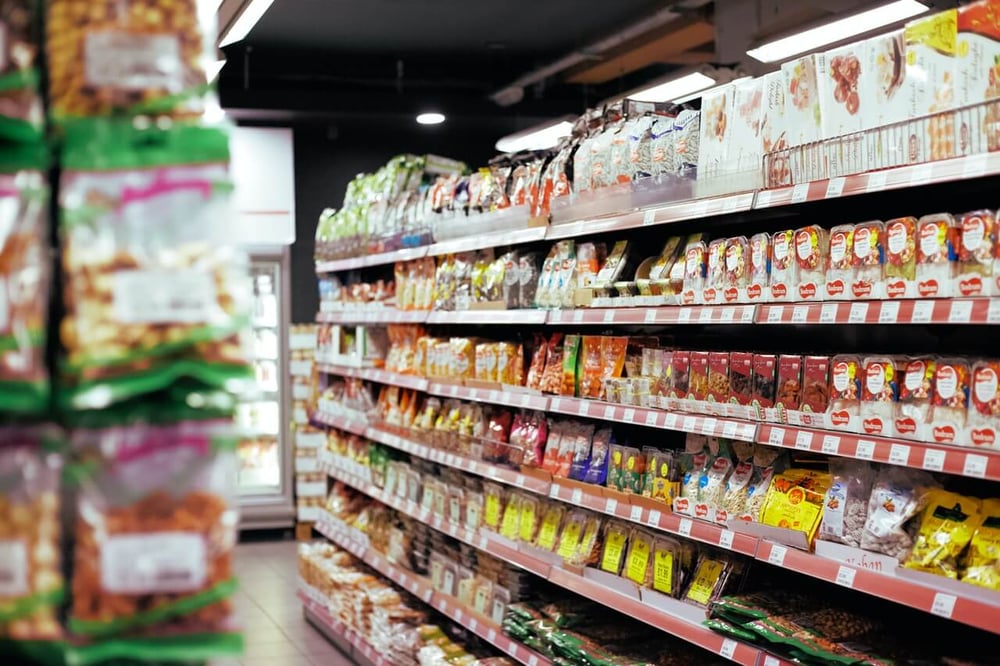
Even before the extraordinary circumstances surrounding the pandemic, the average out-of-stock rate hovered at around 8% for many years. Then, between May 2020 and February 2021, retailers in the US alone missed out on approximately $3 billion in sales due to on-shelf availability issues. Toilet paper accounted for almost $837 million in lost revenue, with paper towels close behind at $689 million. That's a lot of money going down the drain.
Fortunately, there are now some powerful ways to improve on-shelf availability and meet customers’ ever-rising expectations. Let’s look at why OSA is so important and how technology can help you get ahead.
What is on-shelf availability?
By definition:
On-shelf availability means that a product is on the shelf and available to buy, in the correct place, at the right time.
While achieving this sounds straightforward, OSA can be hampered by a variety of factors along the supply chain, for example:
- Stock is available in the store but is in the wrong place or the backroom.
- Stock is on the shelf but is damaged or in an unsaleable condition.
- Stock is in transit or at the warehouse.
- Stock is unavailable to order from the manufacturer or distribution center.
Autonomous stores are changing the way we shop. These stores use advanced technologies such as AI, computer vision, and sensor fusion to enable customers to shop without checkout lines or scanning products. In addition, autonomous stores can improve on-shelf availability by using real-time inventory tracking and predictive analytics to restock products before they run out. On-shelf availability is calculated as the amount of time a product is physically present on the shelf and available to the customer divided by the total shelf-life cycle. With autonomous stores, shopping is becoming more convenient and efficient, setting new standards for the retail industry.
Inefficiency is bad for manufacturers, retailers, and consumers
Inventory distortion – holding too much or too little stock – cost US stores $580 million in 2020, but small improvements can make an impact in several areas of the business.
Internal research by Netguru suggests that:
For on-shelf availability levels of 90% or more, improving the metric by 1% could boost SKU sales by up to 0.8%.
Also, improving the accuracy of data, such as products available on the shelves versus products in the warehouse, can reduce the time managers and staff need to spend on in-store stock management.
However, optimizing on-shelf availability is a balance between increasing revenue and the higher costs of service required to achieve a certain level. For this reason, retailers often aim to keep on-shelf availability at a specific level like 93%.
Besides the obvious monetary cost of inventory distortion, inefficient stock levels create a variety of problems for manufacturers, retailers, and consumers.
Waste
The freshness of products plays a significant role in customer experience for grocery retailers and is an important factor for customers when choosing where to shop. This consideration means that retailers must strike a fine balance between optimizing on-shelf availability, maximizing freshness, and minimizing shrinkage. At the same time, holding too much stock of any product ties up working capital and space in storerooms, warehouses, and distribution centers.
Lost customers
When shoppers can’t find what they’re looking for, 70% will buy a different brand, and 30% will visit a different store. Neither of these options is good for retailers and manufacturers who often spend significant sums of money in marketing and brand awareness to acquire those customers. Depending on the strength of the customer’s loyalty to the store or the product, the retailer and the manufacturer could permanently lose future sales.
Technology that improves on-shelf availability
Retailers currently rely heavily on statistics to drive on-shelf availability, but thanks to technology, improvements can now be made at a low cost.
Inventory tracking tools
Given that traditional stock control processes monitor stock at the store level rather than at the shelf level, video analysis, smart shelves, RFID, and object recognition tools can be game-changing.
RFID, or radio-frequency identification, uses a reader to capture digital data contained in smart tags that can be placed on products. Alternatively, you can use video analysis and object recognition capabilities with machine learning to monitor shelves and detect when items have been removed or misplaced. Both technologies can be combined with smart shelves. Thanks to weight sensors, they will improve accuracy – crucial for stock management and grab & go technologies.
Not only do these tools give you control over the critical “last mile” of getting stock on the shelves, but they can also help to identify issues such as discrepancies in stock figures due to breakages or damage.
At the same time, they can be used in checkout solutions. Amazon Go uses computer vision, sensor fusion, and deep learning to provide checkout-less shopping, and Decathlon leverages RFID to enable a smoother experience at its self-checkout kiosks.
The first step is to place a solution on your service blueprint, identify easy wins, and work on proof of concept. For example, you may find you have a blind spot between the warehouse and the selling floor. By implementing a system focused on the back office, you can significantly boost on-shelf availability without needing to map the entire store.
Machine learning-powered insights
Machine learning solutions can give you a substantial operational advantage.
Using machine learning algorithms on current data can increase the precision of identified goods, generate trend predictions, and detect anomalies.
Another particularly valuable area in which ML can help optimize inventory levels is demand forecasting. Whereas traditionally, forecasting has been based on historical data, trends, and even gut feeling, machine learning can analyze a vast array of variables to accurately predict demand.
For example, you could develop a machine learning-based forecast to automatically consider the impact of the weather on demand for particular products, such as barbecue foods.
Similarly, algorithms based on historical data can better inform safety stock decisions, allowing you to optimize inventory levels to minimize waste and simultaneously avoid out-of-stock situations.
When considering machine learning solutions, any improvements should be preceded with an exploration phase.
Adopt a holistic approach
Retailers have been investing heavily in innovation for decades, and you will likely have many systems and tools already in place. Therefore, when you’re looking at using technology to improve on-shelf availability, a key step is taking a holistic view of your legacy systems and understanding how you can best integrate a new solution.
It may take a little time and investment to get it right, but considering the potential gains in revenue, efficiency, and customer experience, it’ll be worth the effort.