Harnessing the Potential of Price Optimization with Machine Learning
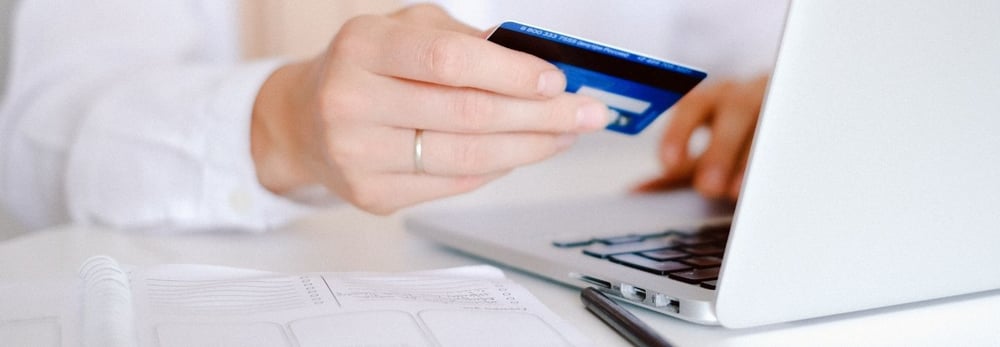
Machine learning models can analyze vast amounts of data and make accurate predictions, enabling businesses to set optimum prices and maximize their profits.
Advanced tech solutions now play a crucial role in price optimization. By applying machine learning algorithms to historical sales data, businesses can gain insights into customer behavior and market trends, which can be used to set more competitive prices. These data-driven decisions have the potential to significantly increase revenues and profits.
The future of pricing in retail and other industries lies in harnessing the power of machine learning. As technology continues to evolve, we can expect to see even more sophisticated machine learning models being used for price optimization. This promises to make pricing even more accurate and effective, further boosting the bottom line for businesses.
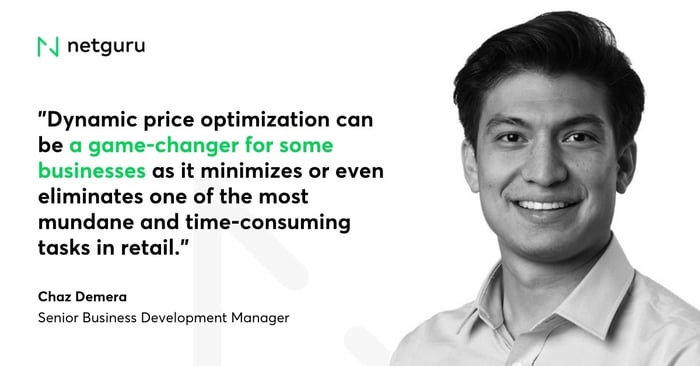
Understanding the Concept of Price Optimization
Price optimization is a strategy used by businesses to determine the best price for their products or services. The objective function is to maximize profits by balancing the price with the expected market demand. This involves analyzing various factors, such as cost, competition, and customer behavior, to find the price point that will generate the most revenue.
With the integration of machine learning, price optimization becomes even more effective. Machine learning algorithms can analyze vast amounts of data, identify patterns, and make predictions, providing businesses with valuable insights that can be used to optimize their pricing strategy. This approach to price optimization is highly data-driven, resulting in more accurate and profitable pricing decisions.
Price Optimization vs Dynamic Pricing vs Automatic Pricing
While price optimization, dynamic pricing, and automatic pricing may seem similar, they represent different concepts in the realm of pricing strategy. Price optimization is a broad strategy that can use any kind of pricing strategy to reach its goals, including dynamic and automatic pricing. It aims at profit maximization by setting the most advantageous price considering various factors such as market demand, competition, and cost.
Dynamic pricing, on the other hand, is a specific pricing strategy where businesses change prices in real-time based on market conditions. It's particularly useful in highly competitive scenarios where market demand fluctuates rapidly. Automatic pricing, also known as price automation, is the use of technology to adjust prices without human intervention. These strategies, when combined with a robust price optimization solution, can provide businesses with a significant competitive advantage in today's rapidly evolving market.
The Intersection of Price Optimization and Machine Learning
Price optimization and machine learning intersect at the juncture where data-driven decision-making transforms the traditional art of pricing into a precise science. This confluence leverages the power of machine learning to understand, predict, and adapt to market trends, customer behavior, and other influential factors. In essence, advanced tech solutions are utilized to streamline pricing decisions, transitioning from human-made decisions to machine-driven ones.
Such a shift in approach offers a significant advantage in the rapidly evolving retail landscape. With machine learning, businesses can dynamically adjust prices based on a multitude of variables, enhancing profitability and customer satisfaction. The future of price optimization, therefore, lies in harnessing machine learning's potential to deliver data-backed, agile, and efficient pricing strategies.
The Role of Machine Learning in Price Optimization
Machine learning plays a pivotal role in price optimization by automating and refining the pricing process. The traditional pricing methods, which often involve manual rule setting and frequent adjustments, are time-consuming and prone to errors. Machine learning, on the other hand, uses historical data to generate predictive models, enabling businesses to make informed pricing decisions.
These machine learning models continuously learn and adapt to changes in market conditions, customer preferences, and other variables. They can identify patterns and trends that humans may overlook, leading to more accurate and effective pricing. The role of machine learning in price optimization, therefore, extends beyond automation to deliver strategic insights, enhanced accuracy, and improved overall performance.
An Overview of Machine Learning-Based Pricing Strategy
Machine learning-based pricing strategies differ significantly from traditional pricing methods. Instead of relying on manual rule-setting, these strategies use machine learning models to predict optimal prices based on historical data. This data-driven approach allows for a granular understanding of various factors that can impact purchase probability, such as customer behavior, market trends, and product attributes.
The machine learning models used in this approach are self-evolving, becoming more accurate with every decision made. They manage to establish their own logic, adapting to the constant changes in the market game rules. This not only makes the pricing process more efficient but also eliminates the need for constant reprogramming, making the businesses' lives easier.
How Machine Learning Reshapes Price Optimization
Machine learning is reshaping price optimization by transforming it from an art based on intuition and experience to a science rooted in data and analytics. With machine learning, businesses can leverage advanced tech solutions to optimize their pricing strategies, making them more responsive and effective. This shift to machine-driven pricing decisions represents a significant leap forward in the retail industry, offering unprecedented levels of accuracy and efficiency.
Furthermore, machine learning models facilitate the automation of the pricing process, reducing the need for human intervention. They can analyze vast amounts of historical data, identify patterns and trends, and make accurate predictions about future pricing scenarios. This ability to analyze and learn from data not only enhances the accuracy of pricing decisions but also allows for a more dynamic and flexible pricing strategy, capable of adapting to changing market conditions and customer behaviors.
Key Advantages of Applying Machine Learning to Price Optimization
Applying machine learning models to price optimization presents several advantages. These models are capable of handling a vast number of products and can optimize prices globally. The intricate nature of parameters, coupled with multiple sources and channels, allows the models to make informed decisions using precise criteria. This would be a challenging task if done manually or using basic software. Moreover, the use of machine learning to optimize prices leads to more accurate results compared to human-based approaches. Retailers can adjust their Key Performance Indicators (KPIs) and immediately observe how the models recalibrate prices for the new goals.
One of the significant benefits of applying machine learning to price optimization is its ability to boost sales during low-peak periods. Additionally, it assists in better understanding and utilizing price elasticity and streamlining inventory management. Machine learning models also facilitate the targeting of new customer segments.
Sales Boost During Low-Peak Periods
Machine learning models can be instrumental in improving sales during off-peak times. Most businesses experience high sales volume during certain months of the year. However, the challenge lies in maintaining sales momentum during the slower months. Machine learning algorithms can help enterprises devise effective pricing strategies that can increase sales frequency during these periods. For example, the model might suggest bundling several products at a discounted rate to enhance their appeal or identifying specific customer segments more inclined to make purchases during off-peak times.
Such strategic measures can lead to a consistent sales volume throughout the year, ensuring that businesses do not suffer from drastic fluctuations in revenue. This aspect of price optimization is particularly beneficial for industries that experience significant seasonal variances in demand.
Utilizing Price Elasticity to Your Advantage
Price elasticity is a crucial factor in determining pricing strategies. It measures how sensitive the demand for a product or service is to changes in price. Price optimization with machine learning can identify customers who prioritize price over time and vice versa. With this information, enterprises can maintain regular or premium pricing for time-sensitive customers eager for immediate purchases.
In contrast, they can offer discounts or lower-cost options to customers willing to wait. For instance, in the transportation industry during holiday seasons, demand is inelastic as people need to travel. Airlines might increase prices but also ensure that more flights are available. However, they may offer discounted fares during off-seasons to attract price-sensitive customers. Thus, machine learning enables businesses to leverage price elasticity to their advantage.
Streamlined Inventory Management and New Customer Segment Targeting
Another advantage of applying machine learning to price optimization is streamlined inventory management. Machine learning algorithms can help enterprises clear excess stock and stimulate sales. After identifying surplus inventories, the algorithm can recommend specific markdown strategies. For example, items that have been in inventory the longest may be discounted first, or items with the highest storage costs may be prioritized for sale.
Furthermore, machine learning models can automatically identify overstocked items and reduce their prices to expedite sales. Dynamic pricing algorithms can also help in identifying new customer segments and targeting them effectively. Thus, smart inventory management creates opportunities and strengthens businesses, showcasing the power of machine learning in price optimization.
Practical Illustrations of Machine Learning for Price Optimization
From transportation to hospitality and eCommerce, machine learning has transformed the landscape of price optimization. By harnessing the power of predictive analytics, businesses can now fine-tune their pricing strategies to maximize profitability, capitalize on market trends, and respond to consumer behavior in real-time. This shift from traditional pricing methods to machine learning-based approaches is not merely a technological advancement; it's a paradigm shift that redefines how businesses operate and compete.
Let's delve into some practical examples of how different industries leverage machine learning for price optimization. We will explore the strategies employed by ride-sharing companies, hotels, and fashion retailers, providing a broad perspective on the applications of machine learning in these highly competitive sectors.
Transportation: How Ride-Share Companies Leverage Dynamic Price Optimization
In the fast-paced world of transportation, ride-share companies utilize machine learning to implement dynamic pricing strategies. These strategies allow them to adjust their pricing in real-time based on various factors such as demand, traffic patterns, and competitor pricing. This approach is particularly advantageous in highly competitive markets where anticipating demand is challenging. By harnessing the power of machine learning, ride-share companies can swiftly react to market fluctuations and optimize their pricing accordingly.
Dynamic pricing strategy, coupled with machine learning, enables ride-sharing companies to navigate the complexities of their operating environment. It empowers them to meet the dual objectives of maximizing their profits while maintaining customer satisfaction. The dynamic nature of this pricing strategy plays a pivotal role in their ability to take quick advantage of certain market situations.
Hospitality: Flexible Room Rates and Effective Inventory Allocation
The hospitality industry has also harnessed the power of machine learning for price optimization. Hotels are now leveraging machine learning to support their inventory management and pricing decisions. With the rise of online distribution and transparent pricing, static hotel pricing has become economically ineffective. As a result, many hotel brands have begun experimenting with dynamic pricing, which allows them to adjust room rates based on factors such as demand, seasonality, and competitor pricing.
Machine learning plays a crucial role in this process by providing predictive insights that enhance decision-making. It allows hotels to manage their room inventory more effectively and adapt their pricing strategies to maximize revenue. This approach not only boosts profitability but also improves customer satisfaction by offering price flexibility.
eCommerce: The Use of Machine Learning-Based Price Optimization in Fashion Retail
In the realm of eCommerce, machine learning is revolutionizing the way retailers optimize their prices. Fashion retailers, for instance, utilize machine learning to respond to demand changes with high accuracy. By analyzing historical sales data, customer reactions to different pricing strategies, and external factors such as market trends, machine learning algorithms can predict optimal prices for various products and sales scenarios.
Take for example, fashion retailer Ralph Lauren. By leveraging machine learning, the company can dynamically adjust the prices of its products, thus optimizing revenue and profit margins. This approach, adopted by a growing number of e-commerce retailers, demonstrates the transformative impact of machine learning on the industry. It empowers businesses to make data-driven decisions that enhance their competitiveness and profitability.
Strategies for Implementing a Machine Learning-Based Price Optimization Solution
Implementing a machine learning-based price optimization solution entails a strategic approach. It requires a deep understanding of business activities, thorough data analysis, and clearly defined business objectives. Gathering substantial historical sales data is crucial to comprehend customer behaviors and patterns, thereby aiding in the formulation of effective price optimization techniques. The goals of the strategy may vary from boosting low-season sales to maximizing revenue or balancing supply-demand. The choice of strategy fundamentally depends on the business aim, necessitating a blend of pricing expertise and machine learning skills for successful implementation.
While tailoring a price optimization solution, it's critical to be mindful of your client's needs. Price changes, if not thoughtfully executed, may be counterproductive. Therefore, it's essential to consider the industry type, business activities, and the potential impact of price changes on customers while crafting a strategy. Ultimately, the success of a machine learning-based price optimization solution hinges on a deep understanding of the business and its customers, coupled with the strategic use of data and technology.
Feasibility and Comprehensive High-Quality Data
A successful machine learning model relies heavily on the availability of comprehensive, high-quality data. For optimal performance, it's imperative to have clean data that's relevant to the product or service. This includes a multitude of data points, each representing a unique variable. Lower quality data often necessitates more feature engineering and sparsity reducing to make the data useful. Therefore, the focus should be on collecting and maintaining high-quality data for more precise and insightful learning.
Another crucial component of the data set is historical prices for each product or service, along with information regarding demand. This data helps to understand the past performance and trends, which in turn aids in making accurate predictions and formulating effective price optimization strategies. Hence, the feasibility and success of implementing a machine learning-based price optimization solution largely rest on the availability of comprehensive and high-quality data.
Adding Pricing Boundaries and Performance Tracking
While machine learning can significantly improve price optimization, it's essential for online retailers to establish pricing boundaries. These boundaries, both minimum and maximum, ensure that margins stay at a preselected rate and prices align with the brand identity. By setting a lower limit, retailers can maintain their profit margin, whereas an upper limit prevents overpricing that might damage the brand's reputation or deter customers.
Moreover, performance tracking is a critical aspect of price optimization. It's not enough to set the prices, but also important to monitor the impact of price changes on sales and revenue. Modern solutions often include a deep neural network-based algorithm that controls the price ceiling and floor relative to competitors, thereby enabling effective price management. Thus, adding pricing boundaries and performance tracking is a strategic move towards effective price optimization.
Machine Learning Models and Techniques for Pricing Optimization
Machine learning offers a myriad of models and techniques that can be leveraged for price optimization. These tools can be particularly useful for setting prices based on data analytics and customer segmentation. However, choosing the right machine learning technique that aligns with your business objectives might be a challenging task. It requires a thorough understanding of different machine learning models, their capabilities, and how they can be utilized for pricing optimization.
Predictive analytics, for instance, can be used to predict demand and set prices accordingly to maximize profitability. On the other hand, data science can be employed to segment customers and tailor prices based on different customer segments, thereby maximizing revenue. By choosing the right machine learning techniques and models, businesses can set prices that not only maximize profit but also enhance customer satisfaction and loyalty.
Demand Prediction Modeling
In the realm of price optimization, demand prediction modeling serves as a critical component. By leveraging machine learning for price optimization, businesses can tap into the potential of demand prediction to set prices that align with customer demand. The power of this technique lies in its ability to forecast changes in demand, enabling businesses to adjust prices proactively based on predicted shifts.
Several key techniques used in machine learning for price optimization include regression-based models, sequence models, and time-series models. These models can help a business anticipate customer demand, taking into account factors such as competitor prices, market conditions, and historical sales. The use of such models can equip businesses to set optimal prices that reflect the price elasticity of demand, thus maximizing their revenue.
Multiple Regression Modeling and Optimization
For those seeking to balance maximum revenue with minimal discounts, multiple regression models can offer an effective solution. Businesses must first train a multiple regression model to obtain pricing coefficients that reflect the relationship between different variables. These variables can range from customer behavior to competitor pricing and more. This model serves as a basis for crafting a pricing strategy rooted in data-driven insights.
However, before implementing a new pricing strategy, it is crucial to test it on a smaller scale. This allows businesses to validate the model's predictions and fine-tune the strategy as needed. By leveraging the power of machine learning in this way, businesses can harness a wealth of data to optimize pricing and drive profitability.
Overcoming Challenges in Implementing Machine Learning for Price Optimization
Implementing machine learning for price optimization is not without its challenges. One of the main hurdles is the need for high-quality historical data. This data serves as the foundation for training machine learning models and deriving insights that inform pricing strategies. To maximize profits, businesses must leverage logistic regression, neural networks, and other advanced machine learning technologies to optimize prices.
Several libraries and frameworks in Python can provide robust support for price optimization using machine learning. These include Scikit-learn, TensorFlow, Keras, PyTorch, and XGBoost. Each of these resources offers a suite of tools and algorithms that businesses can utilize to implement machine learning-based price optimization strategies effectively.
Dealing with Historical Data Scarcity
One of the biggest challenges in implementing machine learning for price optimization is dealing with a scarcity of historical data. This issue, known as the cold start problem, arises when pricing new products or when there is limited historical pricing data available. In such situations, generating reliable price predictions can be challenging.
Businesses can overcome this issue by leveraging data from similar products or categories to bootstrap their machine learning models. Additionally, techniques such as reinforcement learning can prove beneficial. This approach allows the model to learn and adapt as more data becomes available, thus helping businesses navigate the unpredictable waters of price optimization in the stock market and other volatile environments.
Addressing Cross-Pricing Impact and Supply Chain Volatility
In the realm of price optimization, the cross-pricing impact and supply chain volatility pose significant challenges. External factors such as unforeseen disruptions in the supply chain can greatly impact the pricing strategy. Situations like shipping delays, increased production costs, or scarcity of raw materials can cause a divergence between the predictions of the pricing model and the actual outcomes.
To deal effectively with these challenges, integrating real-time supply chain data into the machine learning model is recommended. This makes the model more adaptable to external factors. Including additional features like current stock levels, lead times, and supplier conditions into the model can also help. By doing so, prices can be adjusted dynamically in response to changes in the supply chain, thereby maintaining a balance between profitability and customer satisfaction.
How Different Industries are Benefiting from Machine Learning for Price Optimization
Machine learning has transformed the art of pricing into a science across various industries. The application of advanced technological solutions for price optimization has proven advantageous in many sectors. This shift from traditional pricing strategies to machine learning-based ones has allowed businesses to make data-driven decisions that enhance their profitability.
Industries ranging from retail to transportation are reaping the benefits of machine learning for price optimization. Advanced tech solutions enable these industries to adjust their pricing strategies in real-time, respond promptly to market fluctuations, and make well-informed decisions that ultimately lead to an increase in revenue and customer satisfaction.
Case Studies and Success Stories
An illustrative case study of applying machine learning in price optimization can be seen in the retail sector. Retailers face a complex process of managing promotion calendars that include long-term preliminary planning and frequent adjustments. With the help of machine learning, these retailers have been able to optimize their promotion activities effectively. The model used allows for both long-term planning and weekly adjustments, ensuring optimal price adjustments.
Another success story comes from the apparel retail industry. End-of-season campaigns require continuous tracking of sales progress and timely price adjustments. Using a machine learning-based price optimization model, these retailers have been able to maximize their sell-thru rates and margins efficiently. The model takes into account inventory constraints and uses markdown adjustments, helping businesses to optimize their inventory turnover.
The Future of Price Optimization with Machine Learning
The future of price optimization is promising with machine learning playing a pivotal role. The transformation of pricing strategies from a mere art to a precise science due to machine learning indicates a bright future. The advent of advanced tech solutions has opened up new avenues for price optimization, making it more efficient and effective.
As machine learning continues to evolve, it will provide new opportunities for businesses to further optimize their pricing strategies. The rise of human-made, machine learning-driven decisions is indicative of a future where pricing strategies are more data-driven, adaptable, and successful. The power of machine learning in price optimization is growing, and it is expected to redefine the way businesses operate in the years to come.
More Opportunities and Advancements on the Horizon
As technology continues to evolve, the potential of machine learning models in price optimization is vast and promising. These models can be utilized to perform a variety of tasks related to pricing in retail and other sectors. For instance, given a new product, machine learning can swiftly analyze it and associate it with similar items to identify a suitable price segment. This not only enhances the pricing strategy but also ensures that the new product is competitively priced.
Moreover, machine learning is not limited to predicting prices for existing items; it can also anticipate prices and demands for products that have not yet been sold. Furthermore, machine learning offers in-depth insights into the dynamics of product sales. It can answer important questions such as: How does a drastic price cut on shirts affect the sale of pants? What is the impact on related products when efforts are made to sell more pens? Which customers are likely to buy monitors after purchasing a certain computer? Can a promotion campaign re-engage customers who have been inactive over the past year? The ability of machine learning to answer these questions reveals its potential for future opportunities and advancements in price optimization.
Wrapping It Up: The Power of Machine Learning in Price Optimization
The world of pricing has experienced a shift from art to a science, made possible by the power of machine learning. This advanced technology has brought about significant changes, enabling businesses to harness dynamic pricing strategies and establish optimal price points. With the help of price optimization software, companies can consider factors that influence pricing, such as competition, production costs, customer loyalty, and inventory levels.
Machine learning enables the creation of predictive models. Data scientists develop machine learning models, transforming vast amounts of data into insightful information. The process often involves data analysis techniques to handle missing values and model training to enhance the model's performance. This information informs a price optimization strategy that aligns with business goals and constraints. It enables companies to gain a competitive advantage in the market by predicting customer churn and adjusting their pricing accordingly.
In sectors like car rental, for instance, machine learning is used to optimize prices based on factors such as demand, time, and location. Similarly, assortment optimization in retail can be achieved by analyzing factors like sales history and inventory levels. The future of price optimization is promising, with human-made, ML-driven decisions playing a vital role. In conclusion, the application of machine learning in price optimization presents a powerful tool for businesses, redefining the traditional pricing strategies and paving the way for more accurate and profitable decisions.