What is Strong AI? The Truth Behind AGI
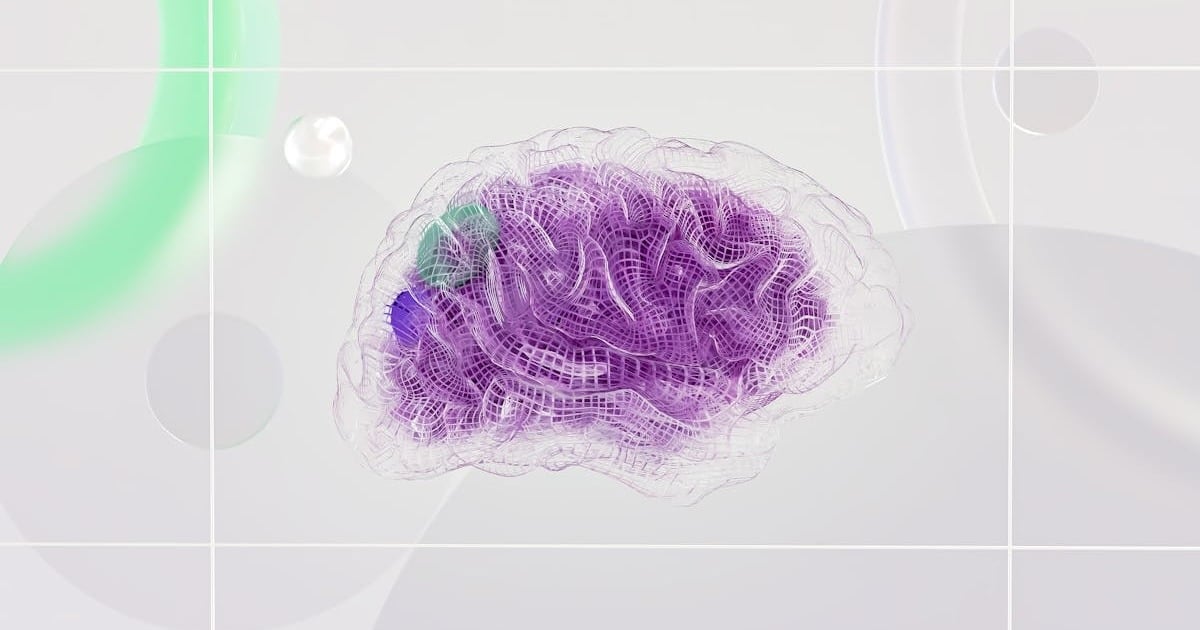
This statistic reveals how far artificial intelligence has come, yet it pales in comparison to the ambitions of Strong AI. Also known as artificial general intelligence (AGI), Strong AI aims to develop systems with human-level intelligence and self-awareness—going far beyond these limited interaction tests.
The divide between current AI systems and Strong AI is substantial. Today's narrow AI excels at specific tasks like image recognition or playing chess, but lacks versatility beyond its programmed domains. In contrast, Strong AI would possess the open-ended ability to learn, reason, and adapt across any field. This means mastering everything from language comprehension and logical reasoning to strategic planning and creative thinking—essentially matching or even surpassing human cognitive capabilities.
No examples of Strong AI exist today, despite the rapid advancements in machine learning and neural networks. The development of true artificial general intelligence would mark a significant milestone in technological evolution. This article examines what Strong AI really means, how it fundamentally differs from the AI systems we currently use, and the various technical approaches researchers are pursuing to achieve human-level artificial intelligence.
We'll explore the key benchmarks used to measure progress toward AGI, clarify the crucial differences between weak and strong AI, and explain why this field continues to captivate researchers and technologists worldwide.
Defining Strong AI: Beyond Narrow Intelligence
Strong artificial intelligence represents a theoretical milestone in AI development that extends beyond the capabilities of today's systems. Scientists and researchers define strong AI as an artificial intelligence system that can match or exceed human cognitive abilities across any intellectual task. In essence, it's AI that doesn't just simulate human intelligence but possesses it.
The Core Characteristics of Strong Artificial Intelligence
At its core, strong AI (also known as artificial general intelligence or AGI) aims to create machines that are indistinguishable from the human mind. While today's AI excels at specific tasks, a truly strong AI system would demonstrate several fundamental characteristics:
-
General problem-solving capability across unlimited domains
-
Self-improvement without human intervention
-
Adaptability to new situations and unforeseen challenges
-
Common sense reasoning and deep contextual understanding
-
Consciousness or self-awareness resembling human cognition
Strong AI would learn from experience and improve its performance over time, eventually developing a form of human-like consciousness instead of merely simulating it. This marks a significant departure from the narrow, task-specific systems dominating today's AI landscape.
How AGI Differs from Current AI Systems
The gap between current AI systems and AGI isn't simply incremental—it's fundamental. Today's AI technologies, classified as narrow or weak AI, excel at specific predefined tasks but lack versatility. An AI might master chess or image recognition but fails entirely when asked to transfer that knowledge to solve unrelated problems.
AGI, in contrast, would demonstrate remarkable flexibility across diverse domains. The primary difference lies in AGI's ability to generalize knowledge from one area and apply it elsewhere without requiring separate training for each new task. AGI would make decisions independently, much like humans, without needing constant programming instructions.
This capability gap becomes obvious when comparing current systems with the theoretical potential of strong AI. Today's narrow AI operates within strictly defined parameters, while AGI would reason through complex problems using logic and abstract thinking to generate solutions. Strong AI would handle a wide range of tasks and adapt to new environments without reprogramming.
The Quest for Human-Level Reasoning
Achieving human-level reasoning in AI represents one of computing's greatest challenges. A system exhibiting true AGI would need to demonstrate sophisticated reasoning across domains, potentially developing novel problem-solving approaches that might differ from human patterns. This includes the capacity to understand and interpret context in a way that aligns with human comprehension.
Recent advances in large language models have accelerated progress toward this goal. Some researchers note that generative AI systems like GPT-4 demonstrate capabilities approaching certain AGI benchmarks, passing tests like the bar exam and coding playable video games. Nevertheless, these systems still lack the comprehensive reasoning abilities characteristic of true AGI.
The computational requirements for building strong AI remain daunting. Experts estimate that achieving AGI would require processing power at least 100 times greater than today's most advanced supercomputers. The development of strong AI also raises profound philosophical questions about whether machines can truly "think" or "feel" like humans.
As research continues, the pursuit of strong AI merges insights from neuroscience, cognitive science, and computational models to understand how the brain processes information and how machines might replicate these processes.
The Evolution of Strong AI Research
The journey toward strong artificial intelligence began long before the term itself was popularized. Tracing the path of AGI research reveals a fascinating history of bold predictions, disappointments, and renewed enthusiasm that continues to shape our understanding of machine intelligence.
Early Theoretical Foundations (1950-1980)
The intellectual groundwork for strong AI emerged in 1950 when Alan Turing published his seminal paper "Computing Machinery and Intelligence," which introduced the imitation game—now known as the Turing Test. This paper addressed the fundamental question "Can machines think?" and established a framework for measuring machine intelligence that remains influential today.
A pivotal moment occurred in 1956 at the Dartmouth College summer conference, where John McCarthy coined the term "artificial intelligence". The attendees of this historic gathering became the leading figures in AI research for decades, with many boldly predicting that machines as intelligent as humans would exist within a single generation.
During this period, significant early systems emerged. In 1957, Allen Newell, Herbert Simon, and J. Clifford Shaw developed the General Problem Solver (GPS) program, which could solve an impressive variety of puzzles using trial and error approaches. Simon famously declared they had "solved the venerable mind-body problem, explaining how a system composed of matter can have the properties of mind".
AI Winters and Renewed Interest
Despite initial optimism, the field experienced its first major setback in 1973 when James Lighthill published a critical report on AI research in Great Britain. The "Lighthill Report" concluded that AI had failed to deliver on its early promises, leading to drastic reductions in government funding. This triggered what would later be termed an "AI winter"—a period of diminished interest and investment in AI research.
A similar pattern repeated in the 1980s. In 1984, Marvin Minsky and Roger Schank warned of an impending second AI winter at a meeting of the Association for the Advancement of Artificial Intelligence. Their prediction proved accurate as funding collapsed again within three years, following a growing gap between promises and achievements.
Interestingly, these AI winters differed from what might happen with AGI today. Historical winters were crises of practicality, driven by failures to translate narrow AI paradigms into reliable applications. In contrast, narrow AI now enjoys commercial viability independent of AGI progress.
Current Research Paradigms in AGI Development
Contemporary approaches to strong AI have evolved significantly since those early days. Current paradigms include:
-
Cognitive Architectures: Frameworks such as Soar and ACT-R aim to model underlying processes of human intelligence by integrating perception, reasoning, and learning into unified systems.
-
Neural Networks at Scale: Advanced deep learning approaches have set the stage for AI advancements, though researchers recognize their limitations for achieving true general intelligence.
-
Hybrid Systems: Many researchers now pursue pluralistic AGI frameworks that evaluate diverse methodologies rather than over-relying on a single paradigm.
-
Reinforcement Learning: This approach focuses on taking signals from the environment to build representations that anticipate behavior and coordinate actions—potentially accelerating in coming years.
Today, achieving AGI requires breakthroughs not only in hardware and algorithms but also in understanding human intelligence itself. The field increasingly recognizes that this demands a paradigm shift that reimagines intelligence as an evolving, self-organizing process capable of adapting to new challenges—critical milestones on the journey toward general intelligence.
Technical Approaches to Building Strong AI
Building a machine with human-level intelligence requires multiple technical approaches, as no single methodology has yet proven sufficient for achieving strong artificial intelligence. The puzzle of AGI demands innovative architectures that can replicate the brain's remarkable capabilities without its limitations.
Neural Network Architectures for AGI
Neural networks form the backbone of nearly all modern artificial intelligence methods, yet they remain poor candidates for AGI in their current form. Deep learning has achieved impressive results in specific domains, primarily through scaling up model size and computational resources. Nonetheless, current neural architectures face fundamental limitations when it comes to generalization across domains.
One promising direction is the development of neural networks with "internal complexity" rather than simply expanding "external complexity." Scientists in China have created a computing architecture inspired by the human brain that can train advanced AI models while consuming fewer computing resources. This approach focuses on making individual artificial neurons more complex—mirroring how the human brain functions efficiently with 100 billion neurons and nearly 1,000 trillion synaptic connections while consuming merely 20 watts of power.
Symbolic AI and Hybrid Systems
Symbolic AI—also called the "top-down" approach—attempts to replicate intelligence by analyzing cognition independent of biological brain structure. This method uses formal logic and explicit rules that humans can understand and verify, making the decision-making process transparent and interpretable. However, purely symbolic systems often break down when confronted with real-world complexity.
Consequently, hybrid approaches combining neural networks with symbolic reasoning have gained traction. Neuro-symbolic AI integrates the pattern recognition capabilities of neural networks with the logical precision of symbolic reasoning. This integration addresses fundamental limitations of each individual approach:
-
Neural networks provide adaptive learning from large amounts of data
-
Symbolic components maintain logical consistency and enable knowledge transfer
-
Together, they create more robust systems requiring less training data
Reinforcement Learning at Scale
Reinforcement learning (RL) has emerged as another promising avenue toward AGI. This approach mimics how humans learn through experience, with algorithms receiving rewards or punishments based on their actions. RL has demonstrated remarkable success in complex environments with many rules and dependencies, making it particularly relevant for developing general intelligence.
Importantly, RL focuses on long-term reward maximization, making it suitable for scenarios where actions have prolonged consequences. This aligns with the hypothesis that "reward is enough" to drive behaviors exhibiting various abilities associated with intelligence. As one researcher notes, "the concept of AGI should track whatever is sufficient to trigger/sustain a singularity by autonomously converting compute to research progress".
Computational Requirements for Human-Level AI
The computational demands for achieving human-level AI remain daunting. The human brain performs approximately 10^14 synaptic operations per second, but these operations are highly sparse. In comparison, modern GPUs can perform a similar number of operations, but only for dense matrix multiplication—making true brain emulation vastly more computationally expensive.
Furthermore, achieving AGI requires enormous amounts of computational power that grows exponentially as AI systems become more complex. Studies show that compute used in the largest AI training runs has been doubling every 3.4 months since 2012, far outpacing Moore's Law. This computational challenge has led tech giants to invest heavily in specialized AI chips and massive chip factories.
Ultimately, no single approach appears sufficient, suggesting that the path to AGI will likely involve integrating multiple methodologies within a unified framework.
Measuring Progress: Benchmarks for Strong AI
Evaluating progress toward strong AI requires sophisticated benchmarks that go beyond simple performance metrics. As AI systems grow more advanced, traditional evaluation methods prove increasingly inadequate for measuring genuine machine intelligence.
Beyond the Turing Test: Modern Evaluation Methods
The Turing Test, once considered the gold standard for AI evaluation, has fundamental limitations for assessing strong artificial intelligence. Although chatbots now routinely pass this test, they lack true understanding or reasoning capabilities. But how do we actually measure something as complex as general intelligence? This question has prompted researchers to develop more rigorous benchmarks.
Among these, the ARC-AGI benchmark stands out for its focus on measuring "skill-acquisition capability" rather than performance on predefined tasks. This benchmark evaluates how efficiently an AI system uses its resources to learn new skills—a core characteristic of general intelligence. ARC-AGI features tasks requiring abstract reasoning without domain-specific knowledge, where humans solve over 80% of problems while even advanced AI systems struggle to exceed 60% accuracy.
The Chinese Room Argument and Understanding
John Searle's Chinese Room thought experiment remains a pivotal critique of strong AI claims. Searle imagined himself following instructions to manipulate Chinese symbols without understanding their meaning, arguing that computers similarly manipulate symbols without comprehension. This highlights a crucial distinction: syntax (formal symbol manipulation) doesn't automatically produce semantics (meaning).
The argument specifically challenges claims that properly programmed computers genuinely understand language or possess actual intelligence. Although computers might simulate thought, Searle contends they lack the biological processes necessary for consciousness and understanding.
Cognitive Architecture Benchmarks
Researchers increasingly rely on cognitive architecture benchmarks to evaluate AGI progress. These frameworks assess sixteen essential functional components across six categories necessary for human-like intelligence. Currently, no existing cognitive architecture incorporates more than 60% of these necessary functions.
Effective AGI benchmarks follow the principle of "Easy for Humans, Hard for AI"—identifying tasks humans solve effortlessly that machines find challenging. This approach targets fundamental gaps in reasoning and adaptability, focusing on intelligence characteristics that distinguish general intelligence from narrow skills.
Weak AI vs Strong AI: Fundamental Differences
Beyond performance metrics and technical approaches, the fundamental distinction between weak and strong AI lies in their inherent nature. These differences illustrate why creating true artificial general intelligence remains such a profound challenge.
Capability Boundaries of Narrow AI Systems
The artificial intelligence we interact with today—from voice assistants to recommendation engines—represents narrow or weak AI. These systems excel within strictly defined parameters but cannot venture beyond their programmed domains. What makes them fundamentally different from strong AI? Weak AI simply simulates human thought processes without actually possessing understanding. It performs specialized tasks effectively, yet struggles with unpredictable scenarios or novel challenges that humans navigate effortlessly. Even IBM's Deep Blue, which famously defeated world chess champion Gary Kasparov in 1997, remains an example of weak AI designed for a single specific function.
Domain-General vs Domain-Specific Intelligence
The contrast between domain-specific and domain-general intelligence forms the core distinction in the weak AI vs strong AI debate. Domain-specific systems (weak AI) function within limited contexts, requiring separate training for each new task. They classify data using machine learning and artificial neural networks but cannot transfer knowledge between domains. Think of these systems as specialists who excel in one area but are completely lost when facing problems outside their expertise.
In contrast, domain-general intelligence—the hallmark of what is strong AI—would demonstrate versatility across diverse contexts without requiring reprogramming. Strong artificial intelligence would possess the capacity to evaluate situations independently and choose appropriate actions, even when they deviate from human instructions. This flexibility represents a quantum leap beyond current technology.
The Consciousness Question in Strong Artificial Intelligence
Perhaps the most profound difference concerns consciousness. Weak AI lacks self-awareness or consciousness of any kind, operating purely on algorithms and predefined patterns. Meanwhile, true strong AI would theoretically possess self-awareness and consciousness resembling human cognition. Indeed, researchers broadly agree that contemporary machines lack consciousness, with only 3% of students in one survey believing current computers possess conscious awareness. This consciousness question remains central to the philosophical debate surrounding strong artificial intelligence.
Conclusion
Strong artificial intelligence represents a quantum leap beyond today's narrow AI systems. Rather than simply executing predefined tasks, AGI would demonstrate human-like reasoning, adaptability, and consciousness across unlimited domains.
The path toward AGI combines multiple technical approaches. Neural networks provide pattern recognition capabilities, while symbolic systems offer logical precision. Additionally, reinforcement learning mimics human experience-based learning. These methods, though promising, highlight the vast computational and architectural challenges ahead.
Progress measurement remains complex, moving beyond simple benchmarks like the Turing Test toward sophisticated evaluations of skill acquisition and reasoning capabilities. The fundamental differences between weak and strong AI - particularly regarding consciousness and self-awareness - underscore why achieving true AGI requires breakthroughs in both technology and our understanding of human intelligence.
Ultimately, strong AI development continues pushing boundaries in computer science, neuroscience, and philosophy. Though current AI systems excel at specific tasks, the quest for artificial general intelligence drives researchers to explore new frontiers in machine consciousness and human-level reasoning. This journey not only advances technology but also deepens our understanding of human cognition and intelligence itself.