.jpg?width=1&height=1)
.jpg?width=1920&height=1282&name=office%20(1).jpg)
Hire AI Engineers
Improve your operations and business performance thanks to AI engineering
Unleash the potential of Artificial Intelligence (AI) to transform your business. Our state-of-the-art solutions are crafted to tackle challenges, streamline operations, and elevate customer journeys. Through AI-powered systems, we excel in providing personalized product suggestions, accurately recognizing objects in images, and optimizing routine tasks to maximize efficiency.
AI solutions have endless applications in various sectors and industries
-
Increase sales. 49% of customers are willing to purchase more frequently when AI is present.
-
Improve productivity. Artificial Intelligence technologies are projected to increase labor productivity by up to 40% by 2035.
-
Analyze large volumes of data. AI gives apps the ability to learn and improve over time. It is extremely adept at quickly identifying patterns and trends.
-
Improve customer satisfaction. 75% of enterprises using AI and machine learning enhance customer satisfaction by more than 10%.
Airbnb enjoys nearly a 4% lift in booking conversions thanks to AI solutions
Airbnb has successfully used AI to create new products, improve its service, and take advantage of new marketing strategies. In addition, it has leveraged machine learning to detect host preferences.
For each search query that a guest enters, Airbnb’s model computes the likelihood that relevant hosts will want to accommodate the guest’s request. In A/B testing, the model showed about a 3.75% increase in booking conversions, resulting in many more matches on Airbnb.

-
What started as a small research project resulted in the development of a machine learning model that learns our hosts’ preferences for accommodation requests based on their past behavior. The model showed about a 3.75% increase in booking conversions, resulting in many more matches on Airbnb.
Bar Ifrach
Former Director of Data Science at Airbnb
Starting an AI project can be daunting – we’ll make sure you find the right solution
-
Data Engineering. Prepare your data to make the most of AI algorithms
-
Data Science. Uncover meaningful insights to improve your products or services
-
Recommender Systems. Create a personalized experience for every user, thanks to an accurate recommendation system
-
Natural Language Processing. Build natural interactions with your users and identify patterns in unstructured data
-
Computer Vision. Automate difficult decision-making processes based on images
-
Audio Recognition. Identify patterns in audio data, enabling voice communication using a range of devices
The right process is a key advantage
Over the years, our team has developed and implemented a robust and efficient workflow for AI projects. Our processes ensure our customers receive more reproducible results faster and in a more flexible way.
Our workflow focuses on three stages:
The Role of AI Engineers
Contents
An AI Engineer is a tech enthusiast who is deeply entrenched in the world of Artificial Intelligence (AI) and Machine Learning (ML). They are driven by a relentless passion to leverage AI to create valuable products that revolutionize business operations.
Their role sits at the intersection of data science, software engineering, and machine learning, carrying immense responsibility and ownership. They must stay agile, just like the technology they work with, which is why we at Netguru endorse the Agile methodology.
Agile methodology is a streamlined process that turns a vision for an AI-driven solution into a working product. It involves continuous planning, learning, improvement, and collaboration within a self-organizing team, paving the way for iterative development and delivery.
AI Engineering serves as a bridge between business objectives, user experience, and technical development, driven by innovation. An AI Engineer helps to:
- Achieve business objectives
- Bridge communication gaps
- Align a common vision with the tech team
- Focus on user needs
- Represent the user inside a company
Why Research is Vital for AI Implementation
In-depth research lies at the core of an AI Engineer's responsibilities. Being the voice of technological innovation requires extensive and up-to-date knowledge about AI and ML trends, coupled with an ability to identify significant technological shifts.
Research into AI implementation offers profound insights that facilitate the decision-making process. Vital information can also be gleaned from a thorough analysis of the AI landscape. Data-driven qualitative and quantitative research helps you make the right AI investment decisions.
Why Validation is Critical for AI Solutions
An idea that is not validated is merely a hypothesis. A key responsibility of an AI Engineer is to probe, question, and find answers. Before implementing an AI solution or a new feature, it's crucial to validate the existing problem and the proposed solution for specific target groups. User feedback helps in steering the AI solution in the right direction or pivoting when necessary.
Various experiments and methods can validate your assumptions. However, poorly designed experiments can lead to misleading and damaging results. An AI Engineer is skilled in conducting both low-fidelity experiments that require minimal investment and complex, high-fidelity experiments that suit the product's needs. Credible validation minimizes the risk of AI solution failure and enhances the likelihood of success.
The Key Objectives of AI Engineering
AI Engineering is a function within a business that deals with AI-driven product development and its justification. It focuses on implementing a new and effective AI solution or enhancing an existing one. The primary objectives of AI Engineering include:
- Building AI Solutions
- Measuring AI Effectiveness
- Improving AI Functionality
The primary aim of AI Engineering is to find answers to critical AI questions: What problem does our AI solution solve? How does it achieve this more effectively than other methods?
The AI Engineering process is about finding the right audience for your AI solution and identifying key features that resonate with the audience. It's a crucial step in validating your business idea to increase your chances of success.
The Importance of Communication in AI Engineering
Dealing with numerous internal and external stakeholders requires excellent communication skills. Good storytelling, public speaking skills, and emotional intelligence play a significant role in the everyday life of an AI Engineer.
A universal tool to maintain transparency and clarity within an organization is an AI roadmap, which represents a high-level concept of the AI solution. An AI Engineer ensures every person in the organization is aligned with a common goal.
Application of AI Engineering in Different Industries
Businesses in all industries can benefit from AI engineering services. Every business sells some sort of product or service that requires a unique approach and specific knowledge. Finance is one example.
In a fintech product, for example, it is crucial to cope with legal affairs and security, while in healthcare, AI-driven solutions need to comply with regulatory requirements and patient privacy laws. Retail businesses can leverage AI to enhance user experience and streamline internal processes.
Netguru specializes in AI engineering for a variety of industries, from the finance sector to healthcare, retail, and beyond.
AI Algorithms and Learning Methods
AI algorithms and learning methods are the driving forces behind any AI solution. For instance, supervised learning involves training an AI model with labeled data, while unsupervised learning works with unlabeled data to find hidden patterns and structures.
Clustering techniques like density-based, hierarchical, and partitioning methods are used to group datasets into clusters based on their similarity. Metric learning, on the other hand, focuses on distance metrics to identify similarities or differences between data points.
Furthermore, cutting-edge techniques like few-shot learning have emerged, allowing AI models to understand and make accurate predictions from very few examples. This ability significantly reduces the need for large amounts of training data and accelerates the learning process.
Scikit-learn and Keras are examples of libraries offering a host of algorithms for various machine learning tasks. Scikit-learn provides simple and efficient tools for predictive data analysis, including clustering, regression, and classification algorithms. Keras, a user-friendly neural network library, is built over TensorFlow and Theano, specifically designed to enable fast experimentation with deep neural networks.
By integrating these cutting-edge tools and resources, Netguru's team of seasoned AI Engineers ensures the development of powerful AI solutions that are tailored to your business needs, heralding a smarter, AI-driven future.
In conclusion, AI Engineering is a dynamic and continually evolving field, powered by a range of tools, frameworks, and algorithms. Netguru's team of experienced AI Engineers harness these resources to deliver AI solutions tailored to your business needs, paving the way for a smarter, AI-driven future.
Why Hire Netguru for AI Engineering Services?
When you partner with Netguru throughout the entire AI lifecycle, you have a reliable ally dedicated to helping you create well-researched and effective AI solutions. We offer end-to-end AI development services, meaning our skilled team is here throughout the process, from validating your ideas and devising an AI strategy to ensuring your AI implementation is successful.
Our AI engineers and teams are equipped to identify market gaps, research user needs, and make sure you achieve an AI-market fit. They're also responsible for building AI roadmaps and ensuring the process runs smoothly, whether you're implementing an AI solution from scratch or improving an existing one.
Reach out to Netguru today and leverage our expertise to create powerful AI-driven solutions that revolutionize your business operations.
Why Netguru should be your AI partner?
-
Practical AI focus. We specialize in translating tech trends into practical and commercially viable AI applications.
-
Diverse AI team. Diverse group of experts, including researchers, product managers, and engineers, ensuring comprehensive solutions.
-
Industry Experience. Hands-on exprience with unique challenges and opportunities in Finance, Retail, Proptech, and Healthtech.
-
One-stop Partner. Comprehensive range of services, including AI, consulting, product design, mobile and web development and more.
World-class specialists at your disposal
Our developers are open source contributors with more than 220 repositories on GitHub.
We constantly invest in developing new technologies and testing solutions in our R&D department, sharing our experience both on expert blogs and at various tech industry conferences such as IGARSS, AAIA, and MICCAI.
Our team has worked on a variety of engagements, including many end-to-end projects.
Personalized shopping with Countr
Countr is a personalized shopping app that enables its users to shop with their friends, receive trusted recommendations, showcase their style, and earn money for their taste – all in one place. Using machine learning, we delivered recommendation and feed-generation functionalities and improved the user search experience.

Speech-to-text transcription with CocoonWeaver
We designed an intuitive UX and developed a neural network that, together with Siri, enables the app to perform speech-to-text transcription and accurately produce notes with correct grammar and punctuation.

Audio recognition with BabyGuard
To achieve high performance, we used custom audio processing algorithms and neural networks to handle the classification of the signal. The system can detect a baby’s cry rapidly and accurately. Our designers handled the UX to make the app easy and intuitive to use.
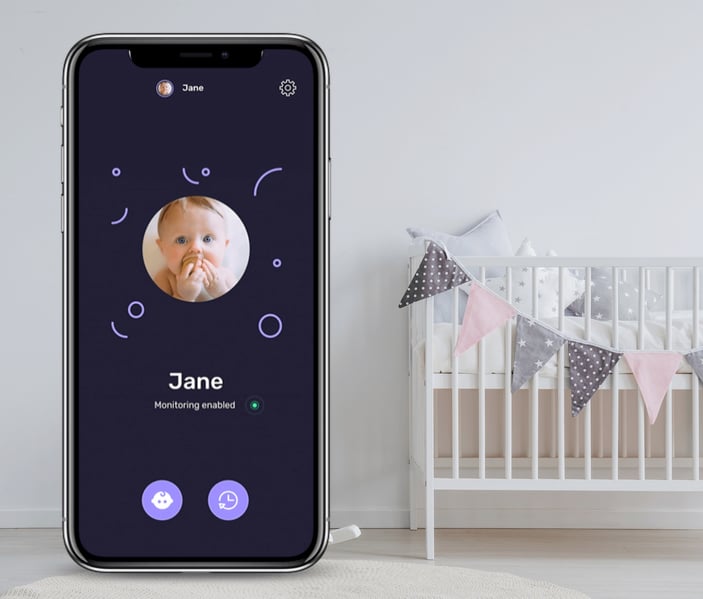
Our partners on working with Netguru
-
My experience working with Netguru has been excellent. Outstanding software teams are resilient, and our developers at Netguru have certainly proven to be that. Our Netguru friends have become as close to team members as possible, and I am grateful for the care and excellence they have provided.
Gerardo Bonilla
Product Manager at Moonfare -
Netguru has been the best agency we've worked with so far. Your team understands Kelle and is able to design new skills, features, and interactions within our model, with a great focus on speed to market.
Adi Pavlovic
Director of Innovation at KW -
Working with Netguru has been a fantastic experience. We received a lot of support in terms of thinking about how we track metrics, how we design this properly, and how we build the architecture. We are extremely grateful for making our platform what it is today.
Manon Roux
Founder at Countr
15+
Years on the Market400+
People on Board2500+
Projects Delivered73
Our Current NPS Score
Delivered by Netguru
We are actively boosting our international footprint across various industries such as banking, healthcare, real estate, e-commerce, travel, and more. We deliver products to such brands as solarisBank, IKEA, PAYBACK, DAMAC, Merck, Volkswagen, Babbel, Santander, Keller Williams, and Hive.
$5M
Granted in funding for Shine. Self-care mobile app that lets users practice gratitude$28M
Granted in funding for Moonfare. Investment platform that enables investment in private equity funds$20M
Granted in funding for Finiata. Data-driven SME lending platform provider$47M
Granted in funding for Tourlane. Lead generation tool that helps travelers to make bookings
AI services: All your questions answered
AI Development Tools and Frameworks
What are AI Development Tools and Frameworks?
What are Advanced AI Models?
What is Data Warehousing and Management?
What are Cloud Computing Platforms?
What are Data Visualisation and Reporting tools?
What are Machine Learning Platforms?
What are the most impactful skills in AI Engineering?
What is AI Development?
What is AI Tooling?
What is Azure OpenAI Service?
Read more on our blog
Check out our knowledge base collected and distilled by experienced professionals
Our work was featured on
Start your project with us or take an existing one to the next level

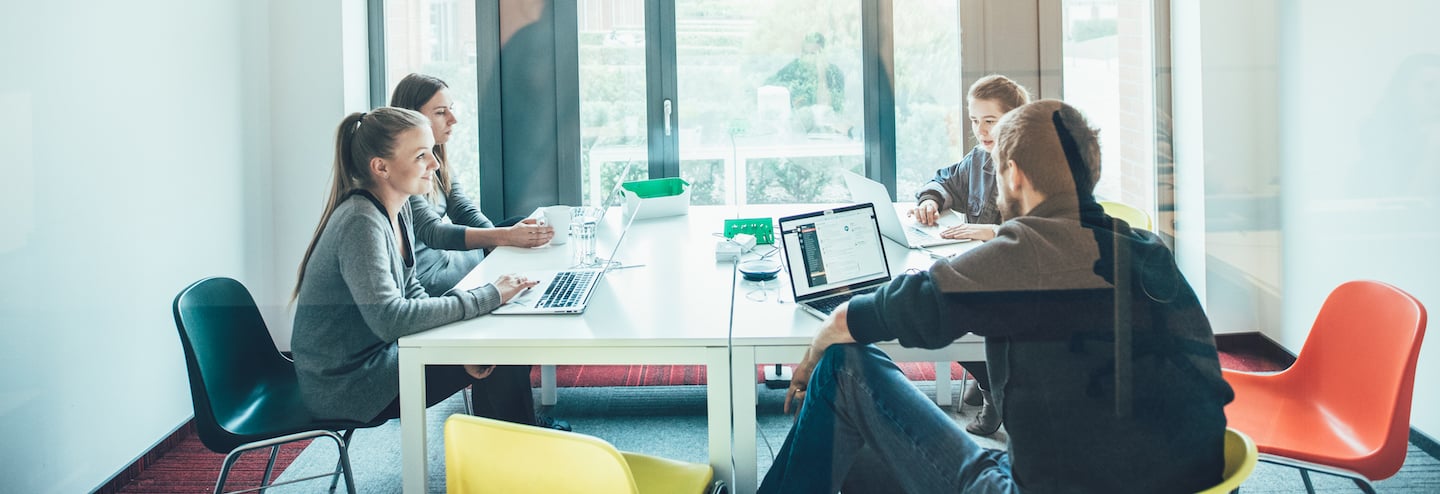
Looking for other services?
-
AI Solutions. Get ahead of the competition with Artificial Intelligence solutions
-
Mobile App Development. Grow your business with the right mobile solutions.