How Leading Insurtech Companies Make Use of AI Solutions such as: Fraud Detection, Hyper-Personalization, and Underwriting
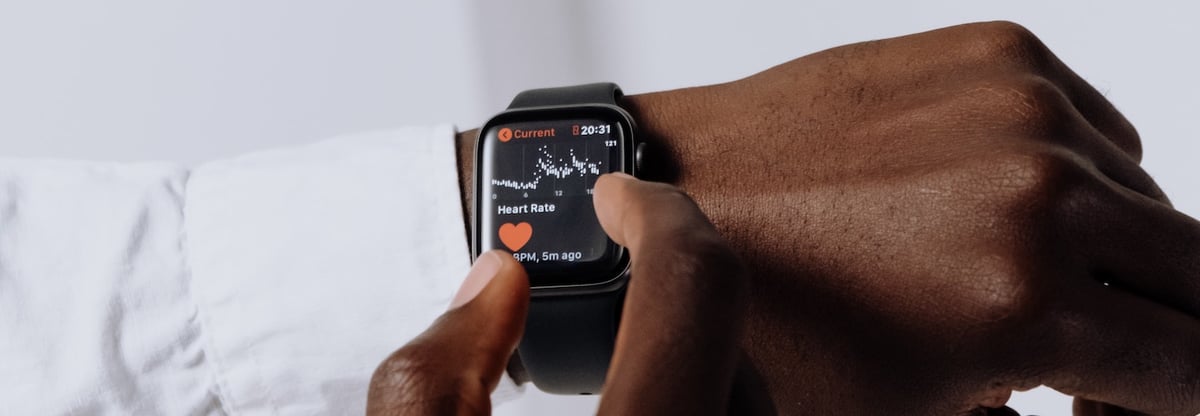
Both traditional players and insurtech disruptors leverage their strategies on big data analysis and machine learning models for customer service automation, claim processing, underwriting, or fraud prediction.
AI is also shifting the insurance business model with hyper-personalization and value-based healthcare, while IoT is enabling a widespread use of geographic information systems for risk assessment.
The pandemic has accelerated the shift to online distribution and self-service. The companies that can build on the common AI experience will have a better chance to succeed in digital transformation.
The development of open-source frameworks drives the rise of AI in the entire insurance industry. Companies have also learned how to collect and process big data sets, which are shared among organizations – also across the sectors. Finally, automation and optimization help the best scale their operations.
Although the international expansion in insurance is constrained by local regulations, the current technological revolution has already led to an even faster consolidation. The insurance industry has experienced an increased M&A activity in recent years, and AI development as well as the pandemic could increase the trend.
Insurtech startups and scale-ups such as Clover Health, Fabric, GetSafe, Trov, Lemonade, BIMA, Slice, neos, ZhongAn use AI to successfully challenge traditional companies. Many tech disruptors focus on delivering their services to the insurance industry.
Quantemplate is a good example. The startup offers self-service, cloud-based insurance data analytics, and automation tools to large and medium-sized companies. According to the company’s statements, Aegon Blue Square Re N.V., XL Insurance, SageSure, Chubb, RenaissanceRe are among its clients.
Meanwhile, the most prominent players don't stay behind, making use of advanced chatbots, fraud detection algorithms, or mechanisms improving cost-effectiveness and productivity. According to a report by Deloitte, 98% of insurance executives say that cognitive computing (models simulating the biological brain, such as neural networks) will play a disruptive role in the insurance industry.
A year ago, we named six ways machine learning (the most popular AI application in business) changed the insurance industry: process automation, better rating algorithms, improved underwriting, predicting customer lifetime value, fraud detection, and marketing personalization. The market has advanced since then. Here is how the applications of AI in insurance have matured over time.
Insurance AI trends common with other industries
Insurance uses AI for recommendation engines, marketing automation, and retention management systems. Chatbots help insurers ease the burden of standard customer service, just like in fintech companies, where AI-based communication solutions such as Cleo, Eno, or Wells Fargo Bot work great to enhance the customer support process.
Automatic communication systems can react quickly to immediate customer needs and improve their experience substantially. Robots can handle the exchange of multiple emails, making sure all documents required for underwriting are collected.
Lemonade created their own chatbot, Maya, to make the customer support process as quick and as pleasant. Swiss insurance company Zurich used a solution by conversational process automation (CPA) startup Spixii, to deliver a similar experience – the Zara chatbot – in just five weeks.
However the greatest value lies in automating core insurance processes such as profiling and underwriting. In the next part of the article, we'll take a closer look at the trends specific to insurtech, where AI is used to automate some key processes, improve essential KPIs, or turn the current business model upside down.
Insurance hyper-personalization
This is one of the strongest disruptions in the industry. In healthcare or car insurance, big data analysis is used to assess each individual's risk.
Wearables and telematic devices collect and send data about customers’ lifestyles or driving habits. The data is used to train neural networks to predict the probability of an accident. Customers opt-in for this program to earn a substantial premium discount, while insurers can estimate risk better. It's a mutual benefit.
Wearables are conquering the private healthcare insurance market. UnitedHealthcare Motion is a wellness opt-in program by an American insurance company that nudges participants into a healthy lifestyle.
Vitality is a behavior change platform launched by the South African insurer Discovery, and it’s also present in the US and the UK. Customers buying Vitality Heat insurance get a deal on an Apple Watch and can collect "activity points" for walking, running or having their blood pressure checked. The program is targeted at employers who want to improve the health of their teams.
While insurance personalization opens possibilities for microsegment marketing and timely assistance, privacy concerns limit hyper-personalization.
A Gartner survey among over 2,500 US customers showed that 38% of them would stop doing business with a company if they would find their efforts towards product or services personalization "creepy", or in other words too intrusive, making them feel uncomfortable. Insurance companies offering such products need to bear in mind that hyper-personalization is more a communication than technological challenge. As Luca Russignan, EY global insurance knowledge leader, points out, when it comes to trends “storytelling is more important than data-driven approaches”.
Value-based healthcare insurance
A value-based system may be more beneficial for both the insurance companies and customers than the traditional one.
In private healthcare systems, insurance companies are the primary payers. Customers pay their monthly premiums to be served in case of getting sick. When it happens, the insurance pays the clinic or hospital for the treatment.
In the US, this payment-per-procedure model proved to be unsustainable. The cost of healthcare is ballooning, while the results are shaky. The critics point out the system is mostly beneficial to pharmaceutical corporations and hospitals at the customer and insurance company's cost.
Insurtech companies see the crisis as an opportunity. According to Oscar Health CEO Mario Schlosser, the system in the US is broken. The company wants to fix it by simplifying the medical insurance experience. Clover Health uses AI models to assess the risks of each patient's condition and reduce unnecessary hospitalization.
Value-based healthcare emphasises the effectiveness of treatment. AI drives the trend. It allows the insurance companies to pay for the procedure's effect, not for spending three days in a hospital. Pharmaceutical companies are being disrupted, too, as they need to prove that a drug is effective.
Fraud detection
This is a typical use of neural networks in both fintech and insurtech. While real-time identification of suspicious activity can save bank customers from falling victim to theft, it is equally useful in the insurance business.
Marine insurance companies use satellite photos and ML image-recognition solutions to verify a claimant's credibility and claim integrity.
Paris-based startup Shift delivers AI-based software that helps insurers detect fraud patterns – their software-as-a-service solution flags suspicious claims with a 75% hit rate.
According to a customer story presented by Dutch fraud detection company FRISS, Turkish insurer Anadolu Signorta reached 210% ROI within 12 months of using their platform.
IoT in property and casualty insurance
Household insurance will be affected by Internet-of-Things devices, enabling geospatial analytics in insurance, which would affect events such as in-home flooding.
AI is used to analyze big data sets and geographic information systems (GIS) to map risk better. The growth of GIS in insurance is driven by the popularity of IoT. Insurance companies can collect geophysical and topographical data, cross-check it with addresses to provide location-based insights.
Although GIS systems have been present in insurance for decades, only the recent development of AI has made the practical use of the big data sets possible.
Automatic underwriting
Claim processing has become much more efficient thanks to automation. According to McKinsey, by 2030, the number of employees needed to conduct underwriting will be reduced by 70-90 percent compared to 2018.
Let's take a look at the car insurance sector, where automatic underwriting is being successfully implemented. In the traditional model, an insurance agent needs to visit each customer, take photographs, verify the claim, and estimate the damages.
Algorithms can do a large part of this process. Image-recognition algorithms can successfully analyze pictures taken by the client. So, provided that it is a standard claim, the agent doesn't have to travel at all.
One of the most valuable insurtech startups, Lemonade, can offer lower premiums thanks to AI. The company reports they have set a world record, handling a claim in just three seconds in a process that included running 18 anti-fraud algorithms.
Shift to digital distribution and self-service
Face-to-face interactions between insurance agents and customers are no longer necessary. This is true for both sales and underwriting. The lockdown caused by the COVID-19 pandemic has accelerated the changes.
In a McKinsey survey among US life insurance agents from January 2020, 90 percent of sales conversations and almost 70 percent of ongoing client conversations took place in person.
The study was done again in May, and less than 5 percent of agents had any face-to-face conversations with clients at all. The social isolation creates pressure for developing self-service models. Automation with the use of ML methods offers a great potential in this field.
Thanks to AI, customers buy insurance products effortlessly, and much faster. As McKinsey's Insurance 2030 outlook points out, AI solutions enabled the insurers to create high-quality risk profiles automatically.
By using neural networks plugged into sources coming from internal and external data providers (including reinsurers and product manufacturers), insurers can present instant quotes. As a result, a commercial, car, or life insurance purchase can take mere minutes or even seconds.
While bottom-up automation of cross-selling and underwriting is already well-developed, top-down data extraction and performance monitoring is still a challenge for many companies, the recent McKinsey "How insurance can prepare for the next distribution model" report indicates.
Wrap-up
New technology is changing the game for every sector of insurance. The pandemic has put to a test the already overextended healthcare system, with new value-based and telemedicine models. Global lockdowns have accelerated digital transformation in all industries, forcing even the most reluctant customer groups to embrace remote and mobile solutions.
On the other hand, trends like hyper-personalization, instant profiling, automatic underwriting and fraud detection can help cut costs and provide better experience to the customers.
Insurance companies need to build on AI use cases. Only the ones that can ride the wave of disruptive change will emerge as winners. It all starts with data collection with modular pipelines that scale.
Companies should build their competence in data extraction and processing. They can do it only by acquiring the right talent and developing scalable technology infrastructures that can adapt to the future changes in the environment. Insurtech should use modular software architecture that can easily integrate with external systems, and can be migrated to a yet unknown future stack.