8 Types of AI You Should Know About in 2025
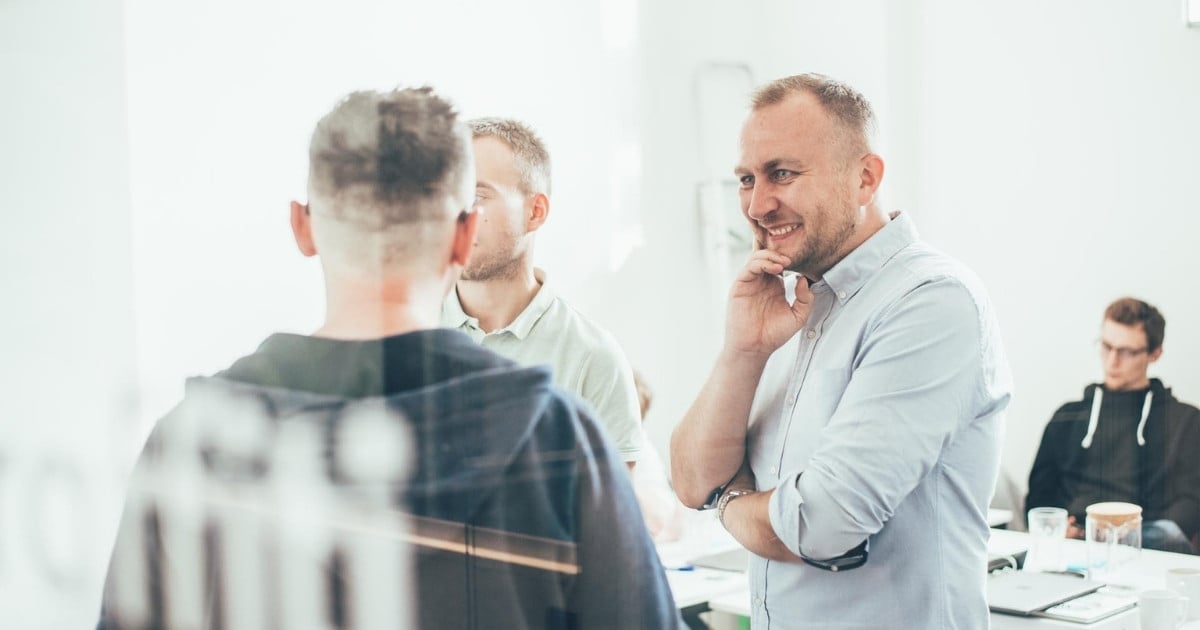
From narrow AI systems that excel in specific tasks to more advanced forms like generative AI and predictive AI, each type brings its own set of unique capabilities and challenges.
Explore a comprehensive look into the different types of AI, exploring their applications, strengths, and limitations. Whether it's the use of AI in self-driving cars, virtual assistants, or cutting-edge machine learning models, this guide aims to help you navigate the diverse landscape of AI technologies and their potential to shape the future.
Narrow AI (ANI): The Foundation of Current AI Applications
Narrow AI (ANI) surrounds us daily, silently powering the technological tools we interact with regularly. Unlike the sci-fi depictions of fully conscious machines, ANI excels at specific tasks without possessing broader capabilities.
What Makes Narrow AI Different from Other Types of Artificial Intelligence
Narrow AI, also called "weak AI," refers to systems designed for specific, predefined tasks. This type fundamentally differs from theoretical concepts like Artificial General Intelligence (AGI) in its scope and capabilities.
The key distinction lies in flexibility—Narrow AI systems operate within strict parameters and excel only at tasks they're specifically trained to perform. For instance, a fraud detection system can identify suspicious transactions but cannot handle customer service inquiries or market predictions.
Narrow AI lacks several critical human-like capabilities:
-
No genuine creativity or innovation beyond programming
-
Inability to understand nuance or context
-
No capacity for adaptation to novel situations
-
Cannot transfer knowledge from one domain to another
Despite these limitations, Narrow AI often outperforms humans in speed, accuracy, and efficiency within its specialized domain. Consequently, it remains the predominant form of AI in practical applications today.
Real-World Examples of Narrow AI in 2025
Presently, Narrow AI implementations are widespread and increasingly sophisticated:
Language Processing Systems: Large language models (LLMs) like ChatGPT, Claude, and Gemini generate text, analyze data, and answer questions within their programming constraints.
Autonomous Systems: Self-driving vehicles use multiple specialized Narrow AI systems for specific functions like navigation, obstacle detection, and decision-making.
Smart Assistants: Virtual assistants like Siri, Alexa, and Google Assistant process commands using Narrow AI to understand human language.
Financial Tools: AI systems analyze transactions in real-time to flag anomalies suggesting fraud, evaluate credit risk, and make trading decisions.
Recommendation Engines: Streaming services and e-commerce platforms use Narrow AI to analyze user preferences and suggest content or products.
Limitations and Future Developments
Despite impressive capabilities, Narrow AI faces significant constraints. It operates essentially as a "black box," making it difficult to understand how decisions are made. Additionally, these systems are only as good as their training data—if the data contains biases or inaccuracies, the AI will perpetuate these flaws.
On the other hand, future developments show considerable promise. As we move toward 2025, we can expect:
-
Enhanced real-time processing capabilities
-
Greater transparency in decision-making
-
Deeper integration with IoT, blockchain, and edge computing
-
More sophisticated applications in healthcare diagnostics and patient care
-
Advanced fraud detection systems in financial services
Key Industries Leveraging Narrow AI
Several sectors are at the forefront of Narrow AI adoption:
Healthcare: AI systems analyze medical images to detect diseases earlier and with greater accuracy than human physicians alone. According to industry data, AI-powered diagnostic tools can process and analyze medical data significantly faster than human capabilities allow.
Finance: Banks use AI for risk assessment, fraud detection, and algorithmic trading. Institutions implementing these technologies report substantial cost savings and improved accuracy in identifying fraudulent activities.
Manufacturing: Predictive maintenance systems analyze sensor data in real-time to determine when machines need repairs, reducing downtime and maintenance costs by up to 30%.
Customer Service: AI-driven chatbots provide 24/7 support and immediate responses to customer queries, lowering operational expenses while maintaining service quality.
Agriculture: Narrow AI analyzes data from drones and ground sensors to monitor crop health, predict yields, and optimize farm operations.
As technology evolves and data becomes increasingly abundant, Narrow AI will continue expanding its capabilities while remaining focused on specific tasks—the foundation upon which more advanced AI concepts may eventually build.
Generative AI: Creating Content and Solutions
Generative AI transforms raw data into entirely new content, making it one of the most exciting types of artificial intelligence in 2025. This technology has evolved from experimental novelty to essential business tool, with the market projected to grow from USD 1.59 billion in 2023 to USD 259.80 billion by 2030.
How Generative AI Works
At its core, generative AI uses neural networks to analyze existing data patterns and produce fresh, original content. Unlike other types of AI that simply categorize or predict, generative models create something entirely new.
Two primary technologies power these systems:
-
Generative Adversarial Networks (GANs): These involve two neural networks—a generator that creates content and a discriminator that evaluates whether that content appears authentic.
-
Variational Autoencoders (VAEs): These compress input data into a compact representation before expanding it to generate new data points.
The process involves analyzing vast datasets during training, enabling the AI to learn underlying patterns without directly copying any specific piece. This allows for creating text, images, video, audio, animation, 3D models, and other types of data.
Popular Generative AI Tools in 2025
Several generative AI tools dominate the market in 2025:
-
ChatGPT and GPT-4: Leading text generation platforms that create human-like content.
-
DALL-E 3 and Midjourney: Advanced image generators producing hyper-realistic visuals.
-
Jasper, Copy.ai, and Writesonic: Specialized in marketing copy generation.
-
Synthesia: Creates AI-generated videos with digital avatars.
-
Runway: Transforms text and images into videos and virtual worlds.
-
Writer: Helps companies create customized content through fine-tuned language models.
ChatGPT remains the most widely used AI tool, with Canva (now AI-enhanced) in second place. Moreover, 73% of U.S. marketers reported using generative AI tools in their work as of 2023.
Content Creation Applications
Generative AI has revolutionized content creation across industries.
Firstly, it streamlines marketing campaigns by generating personalized content, emails, social media posts, and ad copy. By 2025, businesses will use AI for hyper-personalization, tailoring every message and recommendation to individual customers in real-time.
Secondly, it enhances creative processes by offering brainstorming assistance, suggesting alternative perspectives, and helping professionals overcome creative blocks. The global generative AI market in creative industries is projected to reach USD 21.60 billion by 2032.
Additionally, it powers document summarization, knowledge retrieval, and multilingual content creation, making it valuable for businesses operating globally.
Product Design and Development Use Cases
In product development, generative AI has proven particularly valuable:
It accelerates ideation and prototyping by quickly generating multiple design concepts based on parameters. In one case, automotive designers created 25 dashboard variations in just two hours.
Primarily, it enables faster iteration cycles, reducing product development time by up to 70%. This gives teams more opportunity to conduct consumer testing, refine designs, and optimize for manufacturability.
Final Aim and Yamaha compressed what would have been a multi-quarter process into just six weeks when designing customized versions of an electric vehicle, exploring 30 categories of product ideas resulting in over 2,500 concept designs.
Ethical Considerations in Generative AI
Despite its benefits, generative AI raises several ethical concerns.
Intellectual property issues are significant, as generative models trained on copyrighted materials may create outputs that infringe on existing rights. Importantly, many AI vendors do not disclose the data used to train their models.
Environmental impact remains a challenge, as building and running these models requires significant energy and water for cooling.
Bias is another concern, as these systems can perpetuate prejudices present in their training data. This can lead to harmful or discriminatory content if not properly addressed.
Finally, misinformation risks exist because generative AI can create convincing but false information, including deepfakes that could damage reputations or spread propaganda.
As adoption increases, businesses must establish clear guidelines and governance structures while maintaining human oversight of AI-generated content.
Predictive AI: Forecasting the Future
Peering into the future isn't science fiction anymore—predictive AI models analyze vast datasets to forecast outcomes with remarkable accuracy. This technology marks a distinct category among types of artificial intelligence, focused specifically on anticipating what might happen next based on historical patterns.
Core Technologies Behind Predictive AI
Predictive AI harnesses several foundational technologies to generate its forecasts. At its heart, predictive AI combines statistical analysis with machine learning to identify patterns, anticipate behaviors, and forecast upcoming events. This technology examines thousands of factors and potentially decades of data to reach conclusions.
The effectiveness of these systems relies heavily on:
-
Machine Learning Algorithms: Various algorithms power predictive models, including linear regression, decision trees, neural networks, and support vector machines.
-
Data Processing Techniques: Before analysis, data must undergo cleaning, normalization, and feature engineering to improve model performance.
-
Big Data Technologies: Distributed computing frameworks and cloud computing provide the infrastructure to process large datasets.
The accuracy of predictive AI models largely depends on data quality and quantity—as more data is provided to the algorithms, predictions become increasingly reliable.
Business Intelligence Applications
Throughout businesses, predictive AI has transformed decision-making processes. AI-powered predictive analytics enhances operations by:
Foremost, it enables more accurate forecasting of business metrics like profit and savings, estimating the business value that will be realized by deploying predictive models. Instead of relying solely on technical metrics (precision, recall, AUC), forward-thinking companies now evaluate predictive AI based on tangible business outcomes.
Moreover, predictive AI optimizes pricing strategies by examining sales data, seasonality, and non-financial factors to forecast consumer demand and upcoming market trends. This capability allows businesses to adjust their forecasts based on current conditions and consumer behavior.
Nevertheless, most predictive AI initiatives outside Big Tech routinely fail to deploy. What's missing? According to experts, a specialized business practice called "bizML" could bridge this gap by establishing a strategic framework that connects technical capabilities with business objectives.
Healthcare Implementations
In healthcare, predictive AI is revolutionizing patient care with remarkable applications. AI systems analyze patient records to identify subtle patterns, facilitating early diagnosis and treatment of diseases like cancer and Alzheimer's.
Notably, healthcare facilities implementing predictive analytics have reduced patient readmission rates by 10% to 20% according to recent studies. This improvement stems from AI's ability to identify at-risk patients and suggest personalized follow-up care.
Similarly, AI-driven wearable devices monitor vital signs in real-time, alerting healthcare providers to anomalies and enabling timely interventions. These implementations help identify disease outbreaks early and optimize resource allocation in healthcare facilities.
Financial Services Use Cases
Financial institutions increasingly rely on predictive AI for critical operations. Within this sector, AI applications include:
-
Risk Assessment: AI evaluates credit applications and predicts potential losses with greater accuracy than traditional methods.
-
Fraud Detection: Machine learning models analyze transaction patterns to identify suspicious activities in real-time.
-
Market Forecasting: Predictive models analyze past data to forecast market movements and optimize investment strategies.
Traditionally, banks relied on rule-based Anti-Money Laundering transaction monitoring systems that generated numerous false positives. In contrast, machine learning techniques have improved performance and reduced human intervention.
As regulators continue to focus on risk management supervision, financial institutions are developing more reliable AI models, particularly in credit risk management—a trend gaining popularity especially in Fintech and Digital Banking markets.
Reactive Machine AI: Real-Time Decision Making
Unlike other types of artificial intelligence that learn and evolve, reactive machine AI operates on a straightforward principle—specific inputs always produce identical outputs. This fundamental type of AI makes decisions based solely on current information without relying on memory or past experiences.
Understanding Reactive AI Systems
Reactive machine AI represents one of the most basic yet practical forms of artificial intelligence. These systems:
-
Process information in real-time without storing memories
-
Respond to identical situations with consistent outputs
-
Operate within specific parameters for designated tasks
-
Make decisions based only on current data
Reactive AI lacks the ability to learn from previous interactions or predict future outcomes beyond what it's been programmed to analyze. Though limited in scope compared to memory-based systems, reactive AI excels in reliability—delivering consistent results when given the same inputs.
Gaming and Entertainment Applications
The gaming industry showcases some of the most recognizable applications of reactive machine AI. IBM's Deep Blue, which defeated chess grandmaster Garry Kasparov in the late 1990s, stands as a classic example. This supercomputer analyzed chess pieces on the board and evaluated potential moves without any memory of previous games.
In modern gaming, reactive AI powers non-player characters (NPCs) that respond dynamically to player actions, creating more immersive experiences. Games like Red Dead Redemption 2 implement reactive AI systems that analyze player strategies and adjust enemy behaviors accordingly.
Beyond entertainment value, reactive AI in gaming demonstrates how machines can process complex scenarios and make decisions in milliseconds—a capability increasingly valuable across industries.
Industrial Automation Examples
Throughout manufacturing environments, reactive machine AI enables real-time decision making that has transformed production processes. These systems continuously monitor equipment performance, immediately detecting anomalies and initiating corrective actions without human intervention.
Industrial settings benefit from reactive AI's consistency and speed. AI-driven adaptive automation systems can analyze production line data and react to changing conditions instantaneously, maintaining process integrity. Companies implementing these technologies report up to 30% reduction in downtime and 25% improvement in overall equipment effectiveness.
Furthermore, reactive AI enables manufacturing facilities to process real-time data streams for anomaly detection, allowing proactive measures that maintain production quality. This capability proves especially valuable for facilities managing complex operations with numerous variables.
Comparing Reactive AI with Other Types of AI
Reactive machine AI differs fundamentally from other artificial intelligence types primarily through its lack of memory and limited scope:
Albeit simpler than memory-based systems, reactive AI offers superior reliability in specific contexts. Unlike limited memory AI (used in self-driving vehicles), reactive systems cannot learn from past experiences to improve future decisions.
Subsequently, reactive AI contrasts with generative AI, which creates new content, and predictive AI, which forecasts outcomes based on historical data. However, reactive systems excel where consistent, immediate responses matter most—hence their continued relevance in time-sensitive applications.
Indeed, as AI capabilities advance, reactive systems remain valuable foundation components, providing the immediate responsiveness required for real-time applications across industries.
Limited Memory AI: Learning from Experience
Beyond basic reactive systems, limited memory AI represents a significant advancement in artificial intelligence by incorporating short-term data retention capabilities. This type processes and temporarily stores recent information to enhance decision-making, offering a bridge between simple reactive systems and more complex AI forms.
How Limited Memory AI Differs from Reactive AI
Limited memory AI fundamentally diverges from reactive systems through its ability to recall past events and monitor specific situations over time. Rather than operating solely on current inputs, these systems:
-
Temporarily store recent experiences and observations
-
Use both past and present data for decision-making
-
Improve performance as they gather more data
-
Adapt to changing conditions based on recent interactions
Meanwhile, reactive machines remain permanently confined to the present moment, incapable of using historical data to inform their actions. Prior to limited memory capabilities, response times for systems like autonomous vehicles could take up to 100 seconds—afterward, this decreased dramatically, highlighting the practical value of temporary memory retention.
Self-Driving Vehicle Applications
Self-driving cars exemplify limited memory AI in action. These vehicles continuously collect data from their environment, including:
-
Speed and distance of nearby vehicles
-
Traffic signals and road conditions
-
Obstacles and pedestrian movements
-
Speed limits and navigational information
Therefore, autonomous vehicles can interpret their surroundings and make split-second decisions based on both current and recently observed data. This allows them to navigate complex traffic situations safely and efficiently.
Customer Service Implementations
In customer service, limited memory AI powers increasingly sophisticated solutions. Virtual assistants like Siri, Alexa, and Google Assistant combine natural language processing with limited memory capabilities to understand queries and provide contextually relevant responses. Likewise, customer service chatbots retain conversation history to deliver personalized assistance and handle routine questions with remarkable accuracy.
Personalization and Recommendation Systems
Recommendation engines represent another prominent application of limited memory AI. Tools like Amazon Personalize deliver hyper-personalized user experiences by analyzing billions of interactions across millions of items. These systems continuously learn from user behavior, adapting recommendations in real-time as preferences evolve.
For example, streaming platforms use limited memory AI to suggest content based on viewing history, generating up to 80% of watched content on services like Netflix. In retail, personalized recommendations can account for up to 31% of e-commerce revenues.
Future Developments in Limited Memory AI
Ongoing research focuses on enhancing memory capabilities to enable more nuanced decision-making processes. As these systems evolve, we can expect:
-
Improved retention of relevant information
-
Enhanced ability to extract patterns from historical data
-
Expanded applications across additional industries
-
Integration with other AI types for more robust solutions
Computer Vision AI: Seeing and Understanding
Computer vision AI gives machines the ability to interpret and understand visual information from the world around them, making it one of the most influential types of artificial intelligence in 2025. By processing and analyzing images and videos, these systems can perform tasks that previously required human vision.
Core Technologies Behind Computer Vision
Computer vision systems process visual data through several key stages. Initially, raw images are captured through cameras or sensors and enhanced through preprocessing techniques. The crucial step of feature extraction follows, where the system identifies significant visual elements like edges, shapes, and patterns.
Various techniques power modern computer vision:
-
Image classification categorizes entire images into predefined classes
-
Object detection identifies and locates specific objects within images
-
Semantic segmentation meticulously labels each pixel with its category
At the heart of these capabilities lie convolutional neural networks (CNNs), which excel at learning complex visual hierarchies and discerning intricate patterns. Through deep learning, these systems have evolved from 50% accuracy to an impressive 99% accuracy in less than a decade.
Security and Surveillance Applications
In surveillance, computer vision has essentially replaced human monitoring in many contexts. Modern AI-powered security systems can:
Simultaneously analyze multiple camera feeds to detect anomalies like stopped vehicles, intrusions, or abnormal pedestrian activity. These systems perform facial recognition for automated person identification, while also conducting crowd analysis to estimate scene density.
Notably, behavioral biometrics technologies analyze subtle individual characteristics including facial expressions, hand gestures, and body movements to identify specific persons. Advanced systems can even detect aggressive behavior, brawls, robbery, or theft through pattern recognition.
Medical Imaging Breakthroughs
Medical imaging has been transformed by computer vision applications. AI-powered systems analyze X-rays, CT scans, and MRIs with near-human accuracy, identifying patterns too subtle for the human eye to detect.
Currently, these technologies play vital roles in disease detection and diagnosis, with implementation resulting in up to 20% reduction in patient readmission rates. Furthermore, computer vision facilitates personalized treatment planning by accurately delineating structures of interest within medical images.
Retail and Manufacturing Implementations
In retail environments, computer vision monitors shelves in real time, tracking inventory levels and detecting when items are running low. Cashierless checkout systems identify products as shoppers select them, automatically charging customers as they exit.
Coupled with this, manufacturing facilities employ computer vision for quality control and defect detection. These systems perform final checks on finished products, ensuring only the highest quality items reach customers. As a result, companies implementing these technologies report up to 30% reduction in downtime and 25% improvement in equipment effectiveness.
Natural Language Processing AI: Understanding Human Communication
Natural language processing (NLP) bridges the gap between human communication and computer understanding, allowing machines to interpret, analyze, and respond to our language in increasingly sophisticated ways. This specialized type of artificial intelligence has become fundamental to how we interact with technology daily.
Evolution of NLP Technologies
NLP's journey began in the 1950s with early machine translation efforts that aimed to convert Russian into English during the Cold War. These initial attempts were largely unsuccessful yet provided crucial foundations for future development. The field gained momentum in the 1960s with ELIZA, the first chatbot, and SHRDLU, a program that processed language commands for block manipulation.
Throughout the decades, NLP shifted from rule-based approaches to statistical models. The 1980s marked a significant turning point as computing power increased and machine learning algorithms emerged. Today's systems utilize deep neural networks and sophisticated algorithms that grasp context, meaning, and even emotional undertones.
Translation and Transcription Applications
Modern translation tools have advanced beyond word-for-word conversion to understanding cultural nuances and idiomatic expressions. By 2025, AI will facilitate real-time translation for text, audio, and video calls, enabling seamless communication across language barriers.
Transcription services now convert speech to text with up to 99% accuracy across more than 50 languages. Platforms like Sonix and Trint automatically transcribe media content and offer additional features:
-
AI-powered summaries of transcribed content
-
Chapter title generation and thematic analysis
-
Subtitle creation for enhanced accessibility
Content Analysis and Sentiment Detection
Sentiment analysis—a critical NLP application—evaluates emotional tone in text data, categorizing opinions as positive, negative, or neutral. This capability allows businesses to monitor brand perception, social media reactions, and customer feedback at scale.
Text analytics extract valuable information from unstructured data sources, identifying trends and patterns human analysts might miss. By 2025, advanced sentiment analysis will process voice tone, facial expressions, and word choice to assess customer emotions during interactions.
Voice Assistants and Conversational AI
Voice interfaces represent one of the most visible NLP applications. Contemporary assistants like Siri, Alexa, and Google Assistant process commands through natural language understanding (NLU) to interpret human intent.
As we approach 2025, voice assistants are becoming more proactive—anticipating needs before users articulate them. These systems increasingly integrate with other services, transitioning seamlessly between voice and text interactions based on user preferences.
NLP in Business Intelligence
Integrating NLP with business intelligence solutions democratizes data access across organizations. Non-technical employees can extract insights using everyday language rather than complex queries or specialized knowledge.
NLP-enabled BI chatbots retrieve data through conversational interfaces, eliminating the need to access specialized applications for every information request. This advancement transforms how organizations think about growing their businesses and the expertise they require from new hires.
Expert Systems AI: Domain-Specific Intelligence
Expert systems represent a distinctive category among types of artificial intelligence, designed to mimic human expertise within highly specialized domains. These systems excel by focusing on specific fields rather than attempting general intelligence capabilities.
How Expert Systems Differ from Other AI Types
Expert systems fundamentally differ from other AI types through their architecture and operation. They consist of a knowledge base containing domain-specific facts and an inference engine that applies rules to solve complex problems. Unlike generative or predictive AI, expert systems don't hallucinate—they simply stop processing when faced with unknowns.
Typically, these systems work best in environments with low variance and clearly defined parameters. For classification tasks, expert systems follow binary logic—if a rule is met, they classify accordingly. This approach yields superior results in situations where precision and specificity matter more than adaptability.
Healthcare Diagnostic Applications
In healthcare, expert systems analyze patient data to support diagnosis and treatment decisions. They process medical imaging, evaluate bio-signals like ECG and EEG, and interpret laboratory results. Modern diagnostic tools powered by expert systems can detect diseases earlier and with greater accuracy than human physicians alone.
Currently, healthcare organizations implementing AI-powered diagnostic tools report up to 20% reduction in patient readmission rates. These systems prove particularly valuable for analyzing complex genomic data, helping identify disease risks and support personalized treatment strategies.
Legal and Compliance Use Cases
Legal expert systems emulate decision-making abilities of human legal experts, automating tasks like contract analysis and compliance management. These systems review contracts in seconds, flagging potential risks and ensuring compliance with legal standards.
Markedly, law firms using expert systems report significant efficiency gains—document review that once took hours now completes in minutes. This technology effectively addresses the rapid expansion of legal information that began intensifying in the late 1960s.
Financial Advisory Implementations
Within financial services, expert systems evaluate vast amounts of data to uncover trends, make predictions, and support decision-making. AI-powered financial advisors analyze transaction patterns to identify fraud, assess risk, and optimize investment strategies.
Banks implementing AI-based anti-money laundering systems report substantial improvements over traditional rule-based approaches, with reduced false positives and minimized human intervention. As the technology evolves, financial institutions increasingly leverage domain-specific AI for personalized financial planning and advisory services.
Comparison Table
AI Type |
Primary Function |
Key Technologies/Components |
Notable Applications |
Main Industries |
Key Limitations/Challenges |
---|---|---|---|---|---|
Narrow AI (ANI) |
Performs specific, predefined tasks |
Specialized algorithms for single-purpose functions |
Language processing, autonomous systems, smart assistants |
Healthcare, Finance, Manufacturing, Customer Service, Agriculture |
No genuine creativity, cannot adapt to novel situations, operates as "black box" |
Generative AI |
Creates new content from existing data |
GANs, VAEs, Neural Networks |
Text generation, image creation, video synthesis |
Marketing, Creative Industries, Product Design |
IP issues, environmental impact, bias concerns, misinformation risks |
Predictive AI |
Forecasts future outcomes based on historical data |
Machine Learning Algorithms, Big Data Technologies, Statistical Analysis |
Business forecasting, risk assessment, market analysis |
Healthcare, Financial Services, Business Intelligence |
Requires large datasets, accuracy depends on data quality |
Reactive Machine AI |
Makes real-time decisions based on current inputs |
Real-time processing systems |
Gaming NPCs, industrial automation, quality control |
Gaming, Manufacturing, Industrial Automation |
Cannot learn from past experiences, limited to current data only |
Limited Memory AI |
Processes current data while retaining recent information |
Temporary data storage systems, monitoring algorithms |
Self-driving vehicles, virtual assistants, recommendation systems |
Automotive, Customer Service, E-commerce |
Limited retention of historical data, temporary memory only |
Computer Vision AI |
Interprets and analyzes visual information |
CNNs, Image Classification, Object Detection |
Security surveillance, medical imaging, quality control |
Healthcare, Retail, Manufacturing, Security |
Requires significant processing power, dependent on image quality |
Natural Language Processing AI |
Understands and processes human language |
Deep Neural Networks, Language Models |
Translation, transcription, sentiment analysis |
Business Intelligence, Customer Service, Technology |
Complex language nuances, cultural context challenges |
Expert Systems AI |
Mimics human expertise in specific domains |
Knowledge Base, Inference Engine |
Medical diagnosis, legal analysis, financial advisory |
Healthcare, Legal, Finance |
Limited to specific domains, cannot handle unknowns |
Conclusion
Artificial intelligence exists in many specialized forms rather than as the human-like robots often depicted in science fiction. Through our exploration of eight distinct AI types, we see how each serves unique purposes - from Narrow AI handling specific tasks to Expert Systems making complex domain-specific decisions.
These AI technologies deliver measurable business value across industries. Healthcare organizations report 20% lower readmission rates using predictive AI, while manufacturing facilities achieve 30% less downtime through reactive machine systems. Computer vision enables near-human accuracy in medical imaging, and NLP powers increasingly sophisticated language understanding.
Though each type has limitations, their combined capabilities create powerful solutions. Narrow AI excels at specialized tasks, Generative AI creates new content, Predictive AI forecasts outcomes, while Expert Systems apply deep domain knowledge. Limited Memory AI learns from experience, Computer Vision interprets visual data, and NLP bridges human-machine communication gaps.
The future points toward enhanced AI capabilities through improved data processing, deeper learning capabilities, and broader practical applications. Yet success requires understanding each AI type's strengths and limitations while maintaining human oversight of critical decisions. This knowledge helps organizations choose and implement the right AI solutions for their specific needs.