Data Science vs. Business Intelligence: What’s the Difference?
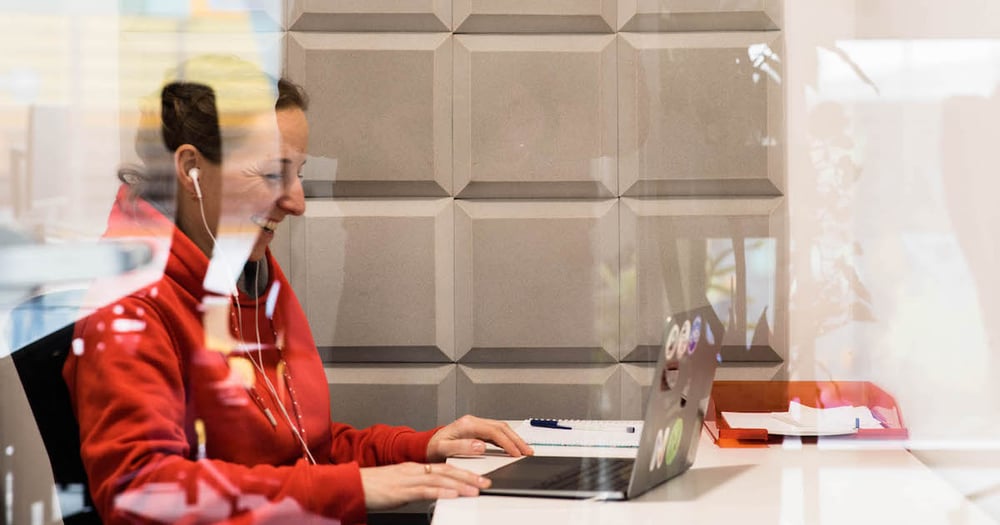
Data science and business intelligence provide different kinds of business insights, of equal value but with different usage possibilities. The core goal in both cases, however, is to provide meaningful data solutions that influence more efficient business processes and lead to accurate future predictions.
What is Data Science?
Data forms the basis of all business decisions in today’s heavily digitized and fast-developing technological world. Everything, from social network statistics to client reviews, falls under the heading of ‘data,’ and getting to the root of what it all means for your business is difficult. This is where data science comes in.
Data science translates the varied information drawn from countless potential data sources into actionable, meaningful insights. These insights can be used to help businesses solve problems, make predictions for the future, adapt to trends, and utilize opportunities more effectively.
Data science streamlines data management and cuts through the vast amount of data available to find those examples of customer interaction, customer feedback, and successful sales, both historically and in real-time, that can guide your business growth decisions.
At its core, data science helps businesses improve the processes that sit at their center and ensures that they stay one step ahead in all areas, from product innovation to website copywriting.
Machine learning and artificial intelligence (AI) can simplify and automate the time-intensive analysis required to get the truth out of business data, turning what was once an unintelligible mass of facts and figures into smooth-running systems that self-improve over time and can be understood by employees at every level.
Implementing data science methodologies into your business plan is a sensible and forward-thinking decision to make, regardless of your business type; but, how does data science differ from business intelligence?
What is Business Intelligence?
Business intelligence (BI) is, first and foremost, an insight into the immediate state of a company. While it cannot automatically provide predictions or suggestions, as data science can, it notably still illustrates trends and insights that can assist in making informed decisions based on the company’s current standing.
Data mining, reporting on data, and dashboard development (with vendors such as PowerBI and Tableau), produced through business intelligence tools present meaningful insights in a way that is easy to understand and react to.
This type of evaluation of business information can compare the successes and failures of one company with its competitors, helping the company implementing BI methodologies to get ahead and learn from mistakes made across the industry.
BI also streamlines structured and semi-structured data, including emails, web statistics, and marketing materials to paint a picture that is simply not possible if done manually. Without this kind of processing, businesses cannot reliably make well-informed decisions based on fact.
After BI technologies have processed, sorted, and organized the company’s data, BI analysts can use this data to predict certain outcomes in a similar way to data science, but this process is usually manual.
The key difference between these two disciplines is that BI offers a new way of looking at data that is already discovered and known, while data science deals almost entirely with the unknown, working without a prescriptive formula and offering insights and predictions that are tailored to each individual business and each individual problem.
In addition, BI requires more specific knowledge about the company’s business model to effectively map the data and draw out meaningful insights, while data scientists can begin with a much more general approach.
So how do these key similarities and differences work in practice?
Business Intelligence vs. Data Science
Here are differences between BI and DS in terms of types of analysis, scope, responsibilities, and more:
Type of Analysis
The starting point of BI and data science processes may highlight their biggest difference as it influences the type of analysis both sets of technology will carry out.
Analysis with Business Intelligence
BI works to a pre-existing formula. A business will approach a BI expert with an idea of what data they want to be extracted and analyzed and the formula they want to use.
The answer that the business hopes to get will already have been defined, at least in terms of scope, though the exact facts and figures that will make up the answer are still to be determined.
The resulting insights will be directly fed into a system that has worked before, to update marketing strategies that are already in place and inform teams on trends that they will already be in some part aware of. In this way, BI analysts work at the end of the data chain, while data scientists begin working with, shaping, and directing the data from the word go.
Analysis with Data Science
Data science starts with a question.
Rather than sticking to a tried-and-tested method of measuring success and failure within the business, data science tries to pre-empt future trends, opportunities, and problems.
Questions posed to data scientists can be far more specific and will often rely on multiple sets of data that are not immediately compatible: client reviews and website traffic statistics, for example. Scientists can then explore the relationship between all available data, rather than only that which is in certain formats, to find a methodology that untangles the query.
Once the best method of determining the answer to this question has been found, analytics tools, including machine learning and AI, are used to create an automatic system that keeps the insights up-to-date. The real-time features of data science also better lend it to mobile analytics, which is quickly becoming an essential asset for businesses who want to quickly and effectively react to the actions and responses of their clients.
Scope
While data science copes with both unstructured and structured sets of data from a variety of different sources, BI is better suited to more structured, static data. With the enormity of the data that is now available to access and analyze, data science is therefore far better equipped to deal with the sheer scope of possibility now on offer.
Part of this is due to the fact that BI technologies pre-date the scale of the big data in existence today, whereas data science has evolved specifically as a way to utilize it.
Data science has quickly developed in order to incorporate real-time data, enabling marketing teams to make timely interactions with a customer based on their real-time actions. BI projects, however, work best with static, historical data from a set period of time, from which complete trends can be discerned.
The simplest way to differentiate between the scope of data science vs. BI, is to consider that data science deals primarily with turning data from the past and present into actionable predictions for the future of a business, while BI focuses on analyzing the past, and sometimes the present, to identify key successes and failures after the event.
It is common, however, to combine the two, particularly if a business is used to implementing business intelligence tools and wants to take their analysis further.
Data science encompasses a wider range of possibilities for businesses than BI.
On its own, BI poses limitations and often relies on human interpretation, which makes the resulting insights and predictions slightly vulnerable. Data science can help propel the trends discovered through BI forwards. AI can react to events, interactions, and changes in trends instantly, generating recommendations at every stage, which often removes the need for heavy human interpretation.
In short, the scope of BI is greatly enhanced when it works alongside data science.
Responsibilities
The responsibilities of BI and data science differ; yet, at their heart, they have the same mission statement: to reveal what data means.
The Responsibilities of Business Intelligence
The BI analyst must turn years of historic data into models and visual tools that reveal trends and progression over time. This information is then compared to the data of competitors within the same industry to help determine cause-and-effect.
BI can uncover vital information that the business can learn from; for example, a breakdown in communication between employees or a dip in sales. This information can help businesses identify causes for profit loss as well as triggers for business growth. AI can help track anomalies and trends to assist in putting together hypotheses for the future.
The Responsibilities of Data Science
The data scientist will combine the same data, insights, and theories as BI, but with a wider range of data types to build predictive models and machine learning algorithms. This, in turn, will produce experimental hypotheses about future trends, events, and solutions to potential challenges.
The data scientist will then more closely collaborate with other departments within a business, including the product development team, to implement the results of these predictions and ensure that the intelligence is put to good use.
Data Integration
In our interview with Wenling Yao, Head of Business Intelligence at Clark, she raised the issue of how important it is to make sure your business is data-driven at every level. Ensuring your employees are all data-driven and -literate requires effective integration.
Easy-to-read and easy-to-access dashboards are key to delivering useful data to everyone. Certain datasets must be made available to relevant employees; for example, every member of the sales team should see who their ideal customer is (and how this changes over time), and every member of the marketing team should see the performance of past and current marketing campaigns.
These figures fall firmly within the scope of BI, and so require analysis only of past data without extrapolation.
While a company may have all of its structured and unstructured data collated together in the form of a data lake or data warehouse, the way that this data is viewed by business employees changes depending on whether the insights are provided by data science or BI technologies.
While BI queries will be shaped by predetermined questions and categories of data, data science queries are more unique, explorative questions that require a more sophisticated approach to sorting data.
Data warehouses and big data are well-suited to BI integration as the general focus of queries asked of the interface will be trends associated with large pools of historic, structured data. For many businesses, this kind of dashboard will work sufficiently.
With data science, however, integration often happens much further down the data analysis timeline. After BI integration has translated data into meaningful insights, data scientists can take these insights and use them to make accurate predictions, which are then delivered back to the business to be used via the dashboard. This allows business users to extract the up-to-date intelligence and forecasts that they need, at the time they need it.
In summary, integration of BI analytics offers business employees a narrower scope of queries to work with, while data science integration allows employees to ask an almost unlimited scope of potential questions.
Skill Set
Breaking down BI and data science into a set of integral core skills can help business owners understand the difference between the two and evaluate candidates who may be applying for data analyst roles within their company.
While there is a considerable amount of overlap, there are some key distinctions between the two disciplines that determine how beneficial they are to each business type.
BI Skill Set
- Understand and interpret data and statistics
- Knowledge about the specific business domain
- Run analysis on historic big data
- Visualize and present data in a way that reveals trends
- Hypothesize solutions to problems based on trends
- Integrate general data insights into day-to-day business transactions
Data Science Skill Set
- Understand and interpret data and statistics
- Practical knowledge of probability
- Run analysis on historic big data, as well as real-time and unstructured data
- Visualize and present data in a way that reveals trends and forecasts
- Hypothesize solutions to problems based on trends and forecasts
- Utilize machine learning and AI to create a self-sustaining data mechanism that will adapt over time to new data
- Integrate general and specific data insights into day-to-day business transactions
Businesses that rely on consistent, long-standing markets may find that BI provides enough insight to guide future business decisions. For those businesses that are constantly reacting to market changes, who want to get ahead in their particular field and improve how their business functions, data science offers enhanced analysis and fact-based, AI-generated predictions.
Conclusion
The current relationship between business and data suggests that we are moving towards a future where BI and data science necessarily go hand-in-hand.
BI will provide the foundation upon which data scientists can formulate their predictions. The streamlined, digestible insights produced by BI technologies form a detailed insight into company history that can be paired with less structured data types to forecast how present trends might change or develop in the future.
Project Brainwave, an AI devised by Microsoft, is an example of how data highlighted by BI technology has been translated into a product that improves business functionality.
The handover of data that will occur between the BI and data science teams will necessitate transparency of data, something that will only encourage the ease of use that is becoming increasingly important. Business users will be able to pick up insights at any point in the analysis process to see what has worked well in the past and what is recommended for the future, facilitating the roll-out of the data-driven workplace
For advice on implementing BI or data science technologies within your business, please speak to us.